Hierarchical Network Analysis of Behavior and Neuronal Population Activity
2019 Conference on Cognitive Computational Neuroscience(2019)
摘要
Recording of neuronal population activity in behaving animals is becoming increasingly popular. Computational markerless annotation tools allow for tracking of animal body-parts throughout the experiment. However, the question remains of how to cross-correlate the extracted behavioral data with the simultaneously acquired neuronal population activity, when both datasets are of high dimensionality. Here we propose a combined analysis, where the behavioral data is clustered into discrete states using a deep learning model and the occurrence of each state is correlated to clusters of neuronal activity. We then model the relationship between behavioral states as a network, where related states are hierarchically grouped while the similarity between their neuronal correlates is maximized. This type of analysis allows for hierarchical exploration of the bidirectional relationship between behavior and its neuronal correlates at different temporal scales.
更多查看译文
关键词
Neural Activity,Radial Basis Function Networks,Neural Synchrony,Cortical Networks,Symbolic Regression
AI 理解论文
溯源树
样例
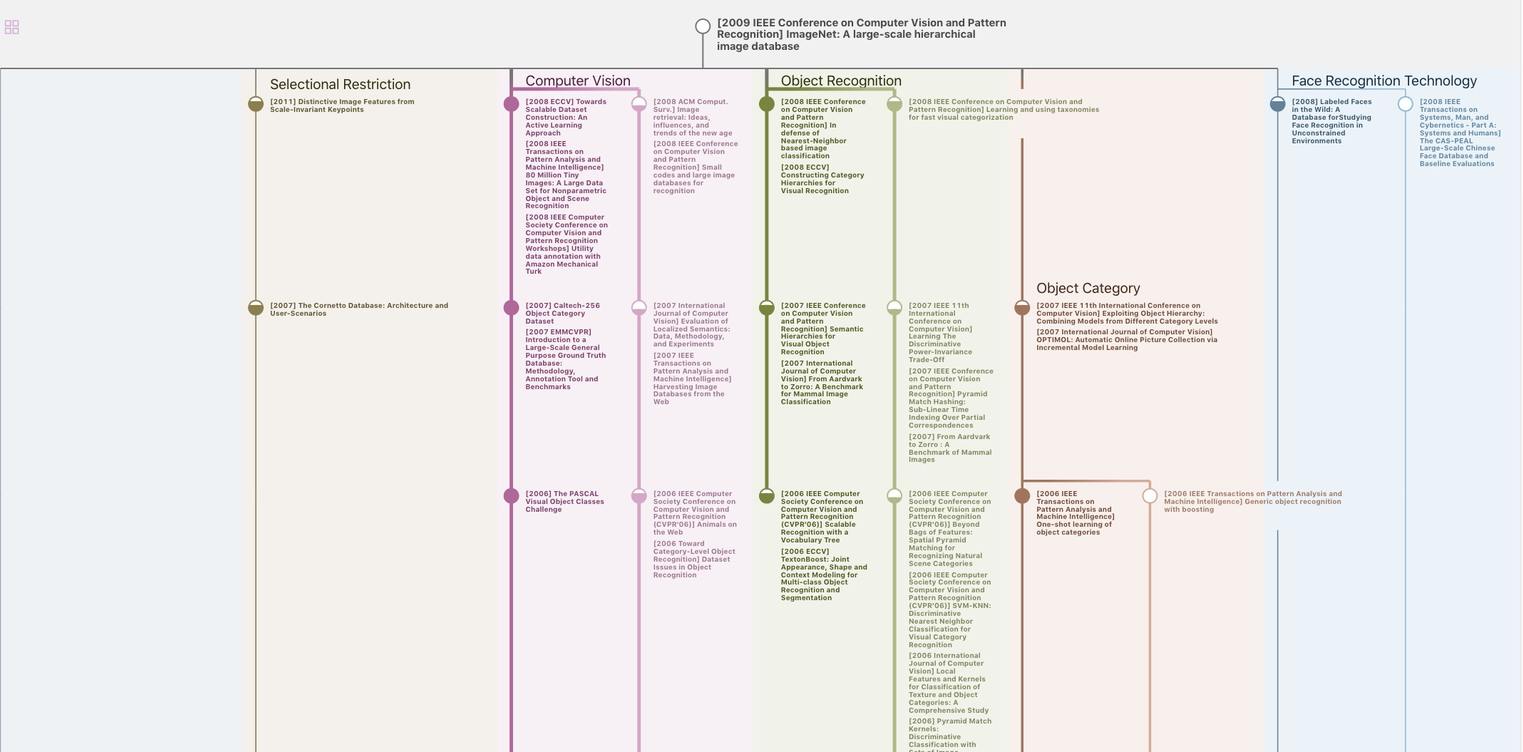
生成溯源树,研究论文发展脉络
Chat Paper
正在生成论文摘要