A Review of Machine Learning Techniques for Anomaly Detection in Static Graphs
Implementing Computational Intelligence Techniques for Security Systems DesignAdvances in Computational Intelligence and Robotics(2020)
摘要
Detection of anomalies in a given data set is a vital step in several applications in cybersecurity; including intrusion detection, fraud, and social network analysis. Many of these techniques detect anomalies by examining graph-based data. Analyzing graphs makes it possible to capture relationships, communities, as well as anomalies. The advantage of using graphs is that many real-life situations can be easily modeled by a graph that captures their structure and inter-dependencies. Although anomaly detection in graphs dates back to the 1990s, recent advances in research utilized machine learning methods for anomaly detection over graphs. This chapter will concentrate on static graphs (both labeled and unlabeled), and the chapter summarizes some of these recent studies in machine learning for anomaly detection in graphs. This includes methods such as support vector machines, neural networks, generative neural networks, and deep learning methods. The chapter will reflect the success and challenges of using these methods in the context of graph-based anomaly detection.
更多查看译文
关键词
static graphs,anomaly detection,machine learning,machine learning techniques
AI 理解论文
溯源树
样例
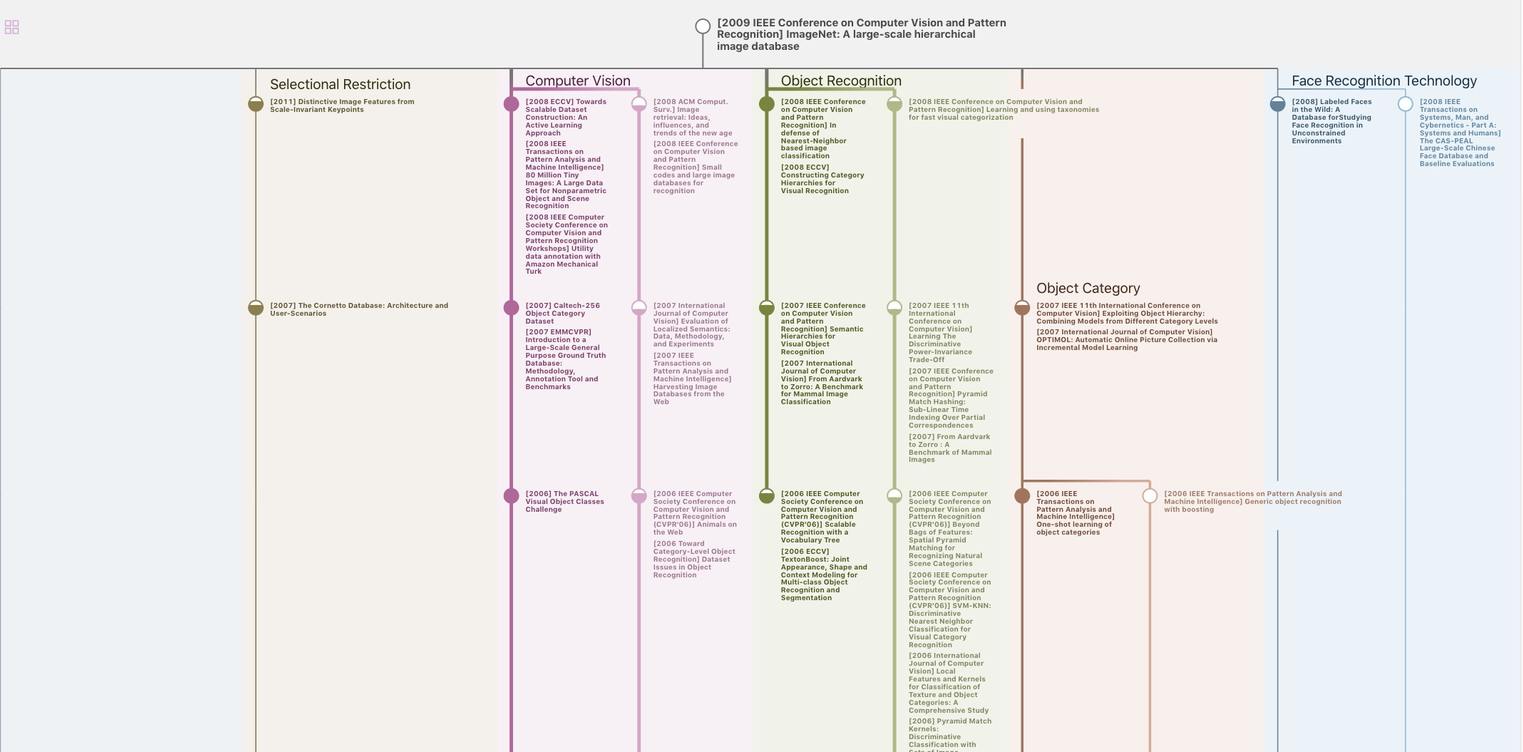
生成溯源树,研究论文发展脉络
Chat Paper
正在生成论文摘要