Machine learning approach for detection of fileless cryptocurrency mining malware
Journal of Physics: Conference Series(2020)
摘要
Abstract Cybercrime is the highest threat to every private company and government agency in the world. Using synergistic threats to attack provides many success alternatives that lead to the same goal, which is to take over the network and carry out illegal mining activities using CPU resources from the victim’s computer. One of the main motives for the success of this criminal business is its relatively low cost and high return of investment. Using the infection chain method in carrying out cryptocurrency mining malware attacks with fileless techniques involves loading malicious code into system memory. Monero (XMR) is by far the highest popular cryptocurrency among threat actor installing mining malware because it comes with full anonymity and resistance to an application-specific circuit mining (ASIC). This work proposes a better method for classifying conventional malware and cryptocurrency mining malware. On the other hand, grouping specific of suitable features extracted from the sources of EMBER dataset shown as malware and need to categorize as a cryptocurrency mining malware. The proposed approach is defining a better algorithm for enhancing accuracy and efficiency for cryptocurrency mining malware detection.
更多查看译文
AI 理解论文
溯源树
样例
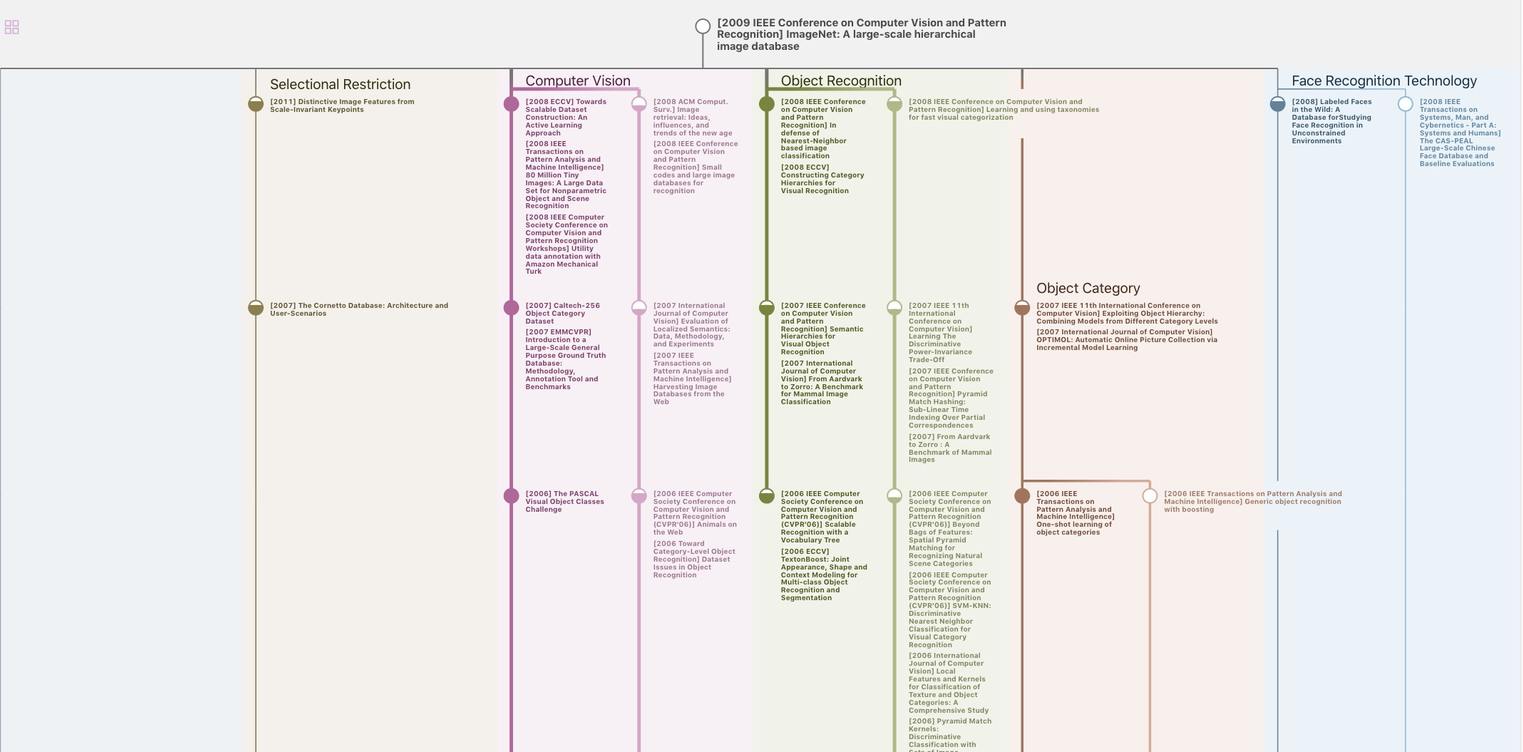
生成溯源树,研究论文发展脉络
Chat Paper
正在生成论文摘要