Abstract P6-13-03: Potential cost effectiveness of18-Flouroestradiol (FES)-PET in metastatic breast cancer
Poster Session Abstracts(2020)
摘要
Background: Potential discordance in estrogen receptor (ER) status between primary and metastatic breast cancer (mBC) site mandates the need for accurate diagnosis. Biopsy is not performed routinely due to inaccessible sites. Additionally, interpretation is limited by specimen processing and a likelihood that the tumor is persistently ER+, leading to variations in diagnosis and therapy selection. Flouroestradiol (FES) is an estrogen PET analogue imaging tracer which measures ER expression at multiple tumor sites simultaneously. FES can potentially be applied to ascertain ER status across multiple sites, thereby providing a more clinically feasible and accurate means to guide therapy selection. To inform the high-value translation of this technology, we used data from the literature to evaluate the potential cost-effectiveness of FES vs. biopsy to determine ER status in mBC. Methods: We created a decision model to evaluate clinical and economic outcomes in a hypothetical cohort of 60-year-old patients with suspected mBC to assess the value of FES vs biopsy to establish ER status. Patients received appropriate/inappropriate therapy based on the accuracy of the test for determining ER status. We obtained test sensitivity, test specificity, costs, and utility inputs from the peer-reviewed literature. The model assessed outcomes with endocrine and non-endocrine treatment regimens to reflect the range of current practice and associated variation in costs and outcomes. The base case evaluated therapy with aromatase inhibitor (AI) for endocrine therapy or capecitabine for non-endocrine therapy. Model outcomes included life years (LYs), quality adjusted-life-years (QALYs), and cost. Probabilistic analyses were conducted to assess uncertainty in model outcomes. We took a payer perspective, evaluated a lifetime horizon, and outcomes were discounted at 3% per year. Results: In the base case, appropriate therapy was provided for 93.1% of patients receiving FES and 80.1% of patients receiving biopsy. FES resulted in appropriate endocrine therapy selection for 63.7% vs 51.5% of patients with biopsy strategy in our model. Table 1 shows outcomes for all strategies. In the base case, FES was expected to increase QALYs by 0.23 and reduce cost by $11,254 per patient—thereby dominating the biopsy strategy. FES resulted in more QALYs than the biopsy strategy (range: 2.5 to 3.2 QALYs) in all alternative scenarios, and FES resulted in higher costs in scenarios applying CDK4/6 therapies (range: $591,843 to $620,631). In probabilistic analyses of FES vs. biopsy, FES increased QALYs in 94% of simulations, decreased cost in 94% of simulations, and was cost-effective ( Citation Format: Poorni Manohar, Hannah Linden, Joshua Roth. Potential cost effectiveness of 18-Flouroestradiol (FES)-PET in metastatic breast cancer [abstract]. In: Proceedings of the 2019 San Antonio Breast Cancer Symposium; 2019 Dec 10-14; San Antonio, TX. Philadelphia (PA): AACR; Cancer Res 2020;80(4 Suppl):Abstract nr P6-13-03.
更多查看译文
AI 理解论文
溯源树
样例
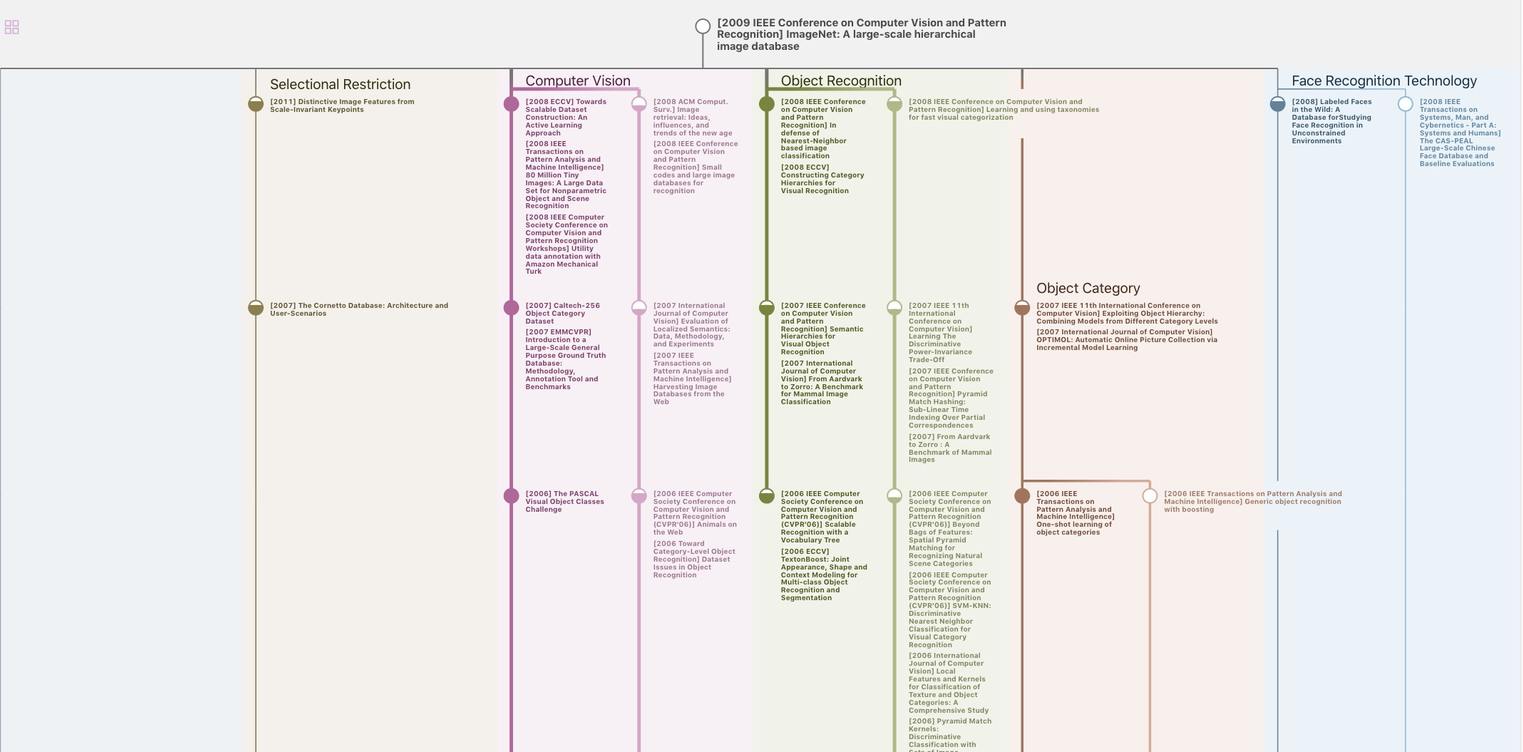
生成溯源树,研究论文发展脉络
Chat Paper
正在生成论文摘要