A Multimodal Non-Intrusive Stress Monitoring From the Pleasure-Arousal Emotional Dimensions
IEEE Transactions on Affective Computing(2022)
摘要
With the increasing development of advanced unmanned aerial vehicles (UAVs), communication between operators and these intelligent systems is becoming more stressful. For the safety of UAV flights, automatic psychological stress detection is becoming a key research topic for successful missions. Stress can be reliably estimated via some biological markers which are not appropriate in many cases of human-machine-interaction setups. In this article, we propose a non-intrusive deep learning-based stress level estimation approach. The goal is to identify the region where the operator's emotional state projects in the space defined by the latent dimensional emotions of arousal and valence since the stress region is well delimited in this space. The proposed multimodal approach uses sequential temporal CNN and LSTM with an Attention Weighted Average layer in the vision modality. As a second modality, we investigate local and global descriptors such as Mel-frequency cepstral coefficients, i-vector embeddings as well as Fisher-vector encodings. The multimodal-fusion approach uses a strategy referred to as “late-fusion” that involves the combination of unimodal model outputs as inputs of the decision engine. Since we have to deal with more naturalistic behavior in operator-machine interaction contexts, the One minute Gradual Emotion Challenge dataset was used for predictive model validation.
更多查看译文
关键词
Operator state monitoring,emotion analysis,continuous emotions,stress monitoring,face analysis,deep learning,feature representation
AI 理解论文
溯源树
样例
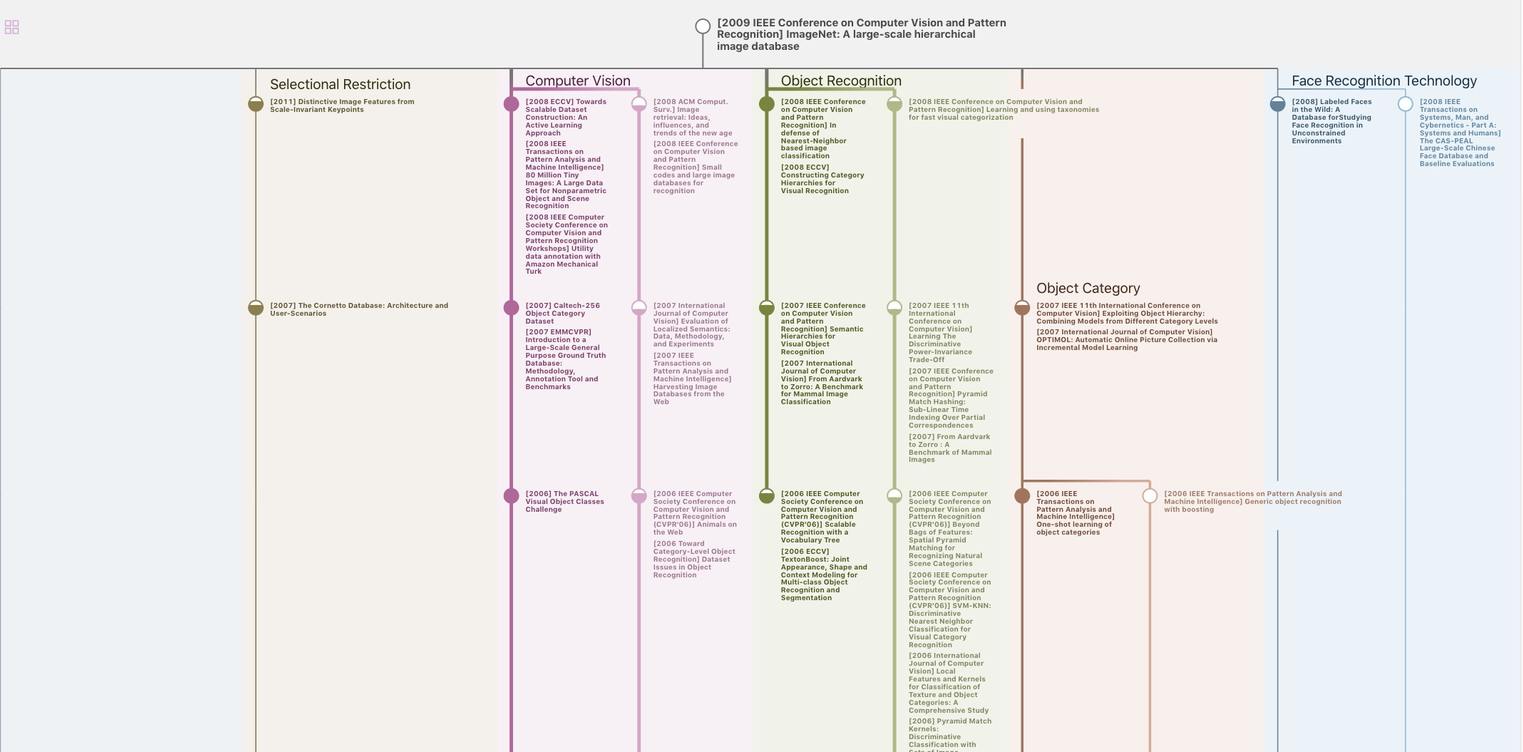
生成溯源树,研究论文发展脉络
Chat Paper
正在生成论文摘要