A Right Ventricle Segmentation Method based on U-Net with Weighted Convolution and Dense Connection
Proceedings of the 2020 2nd International Conference on Intelligent Medicine and Image Processing(2020)
摘要
To solve the problem that the traditional convolutional neural network uses the pooling layers to reduce the image feature dimensions, which leads to information loss and affects the accuracy of right ventricular segmentation, a right ventricular segmentation method based on U-Net improved network is proposed. The dense blocks are used to combine the bottom features of the contracting path, and shortcut connections are used to connect the low-level features and high-level features on the expanding path, which increase the reusability of features. Depthwise separable weighted convolutions are used to enhance the edge detail information and improve the possibility of information reconstruction. An improved shinkage loss function is proposed to solve the problem of unbalanced positive and negative samples. Finally, RVSC-MACCAI 2012 datasets are used in the comparison experiments of different models, and the results show the effectiveness of the improved algorithm with the Dice coefficient of 0.90 and Hausdorff distance of 6.42.
更多查看译文
AI 理解论文
溯源树
样例
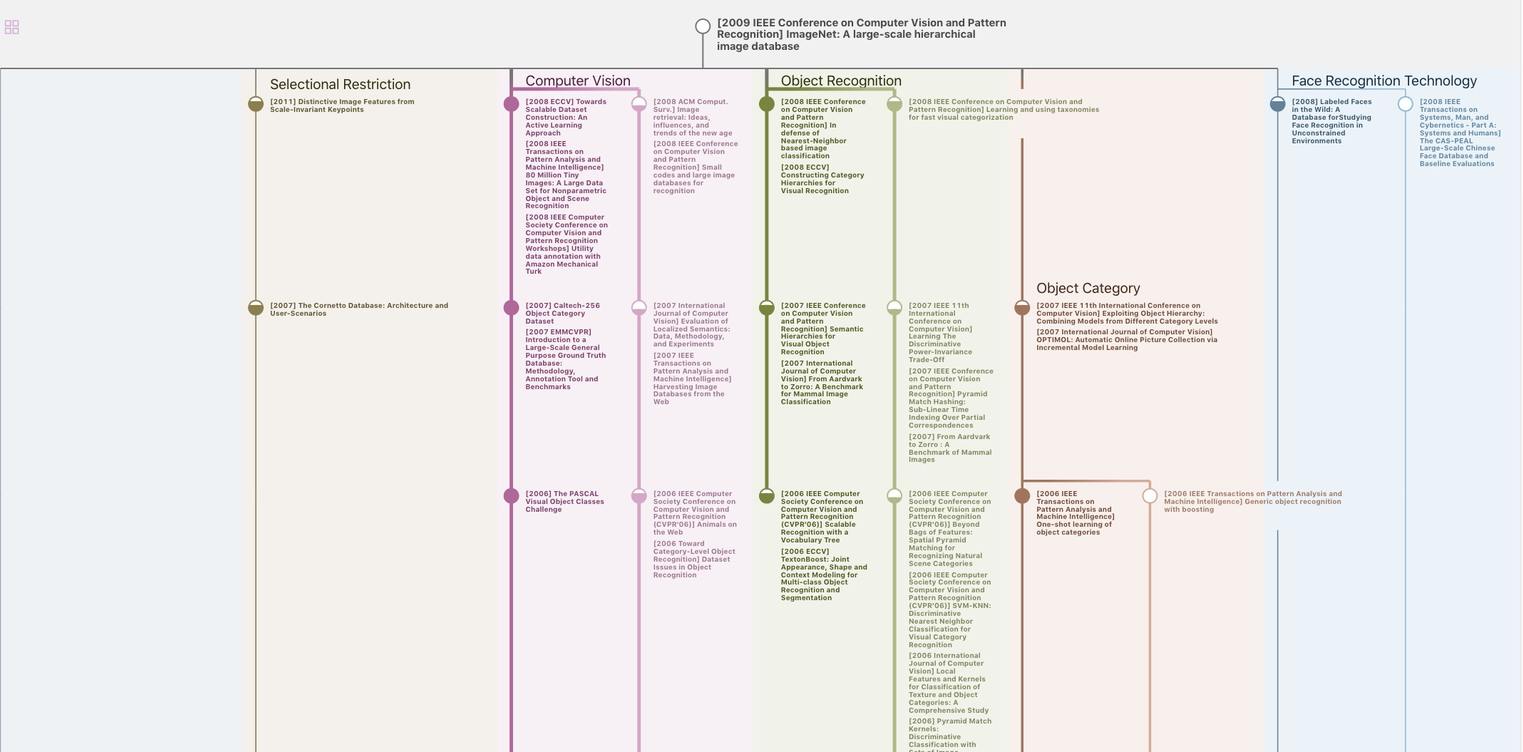
生成溯源树,研究论文发展脉络
Chat Paper
正在生成论文摘要