An Integrated Multi-Disciplinary Modeling and Simulation Approach to History Matching and Forecasting Shale Wells - An Eagle Ford Case Study
Day 1 Tue, April 20, 2021(2021)
摘要
Abstract In the current business environment, oil and gas operators in unconventional plays are continually pushed to reduce risk and increase profitability. Recent advances in horizontal drilling, hydraulic fracturing, and completion design in shale wells have helped reduce costs. However, accurate reserve estimation and production forecasting remain the greatest unknowns impacting the bottom line. The use of advanced subsurface models is gaining momentum to address this challenge. Typical techniques currently used to build and history match these models are based on simplified assumptions and ignore uncertainty in the subsurface characterization. This paper presents an integrated multi-disciplinary shale modeling, simulation, and history matching approach. It incorporates uncertainty from different modeling domains, leading to the generation of improved predictive shale models. The proposed approach leverages micro-seismic data to create a more realistic representation of the Stimulated Reservoir Volume (SRV). Micro-seismic event locations, magnitude, and fracture plane characteristics are used to construct a Discrete Fracture Network (DFN) required for petrophysical modeling. A forward model comprising DFN modeling, an application to generate relative permeability curves, and a reservoir simulator is set up using a common platform integrator. These applications are run in tandem to generate an ensemble of history matched shale models that capture the range of uncertainties in fracture attributes, relative permeability, and other important dynamic parameters. Production data is assimilated into the shale model using Bayesian statistics and state-of-the-art supervised machine learning techniques. Our approach is demonstrated using data acquired from three hydraulically fracked wells drilled in the Eagle Ford shale oil window. The use of Bayesian statistics and machine learning techniques led to the identification of multiple shale models calibrated to the production data. Fracture attributes, saturation endpoints, relative permeability curvature, matrix porosity, and initial water saturation were found to significantly affect the history match. A comparison of prior and posterior ensembles showed a significant reduction in uncertainty in predicted production. The proposed technique ensures that important, previously neglected subsurface uncertainties and their dependencies are captured and used as input to the simulation model. Improved SRV delineation using micro-seismic data and property modeling using DFN, combined with the ability to capture uncertainty through an integrated multi-disciplinary approach, deliver predictive shale models that enable future decisions to be made with a high degree of confidence.
更多查看译文
关键词
forecasting shale wells,history matching,multi-disciplinary
AI 理解论文
溯源树
样例
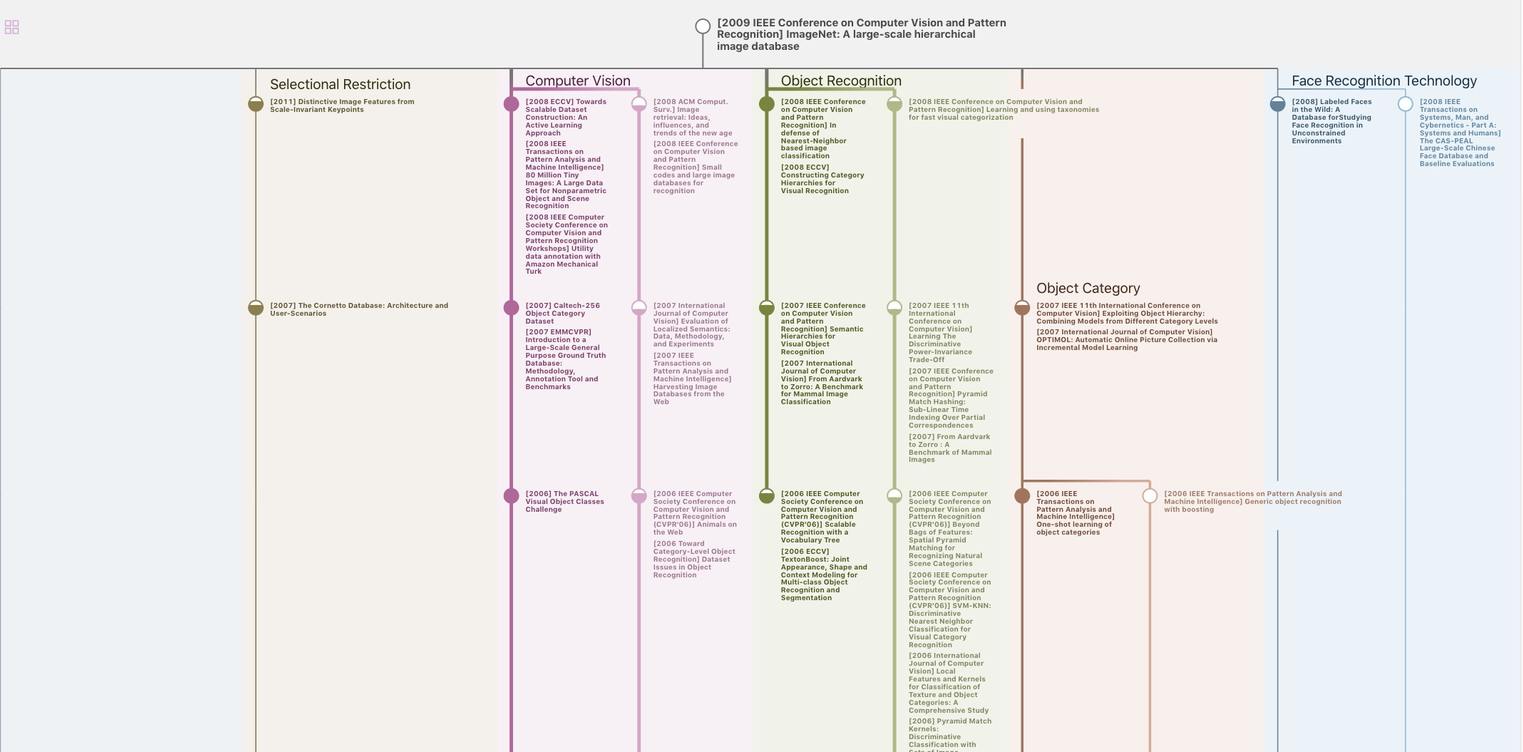
生成溯源树,研究论文发展脉络
Chat Paper
正在生成论文摘要