Selecting a Presmoothing Model in Kernel Equating
Springer Proceedings in Mathematics & StatisticsQuantitative Psychology(2020)
摘要
In the kernel method of test score equating, the first step of the procedure is to presmooth the score distributions. The most common way of doing so is by fitting a log-linear model to the observed-score distributions. In this way, irregularities in the score distributions are smoothed, yielding a more stable estimated equating transformation. Within the kernel equating framework, an alternative way of presmoothing by using item response theory models has recently been suggested. There are furthermore several model selection criteria available for both of these classes of models. Here the model selection criteria are studied for both log-linear and item response theory models. Specifically, the likelihood ratio, AIC, and BIC measures are compared using real admissions data. Results show that the different model selection criteria result in equated scores that have real impact differences.
更多查看译文
关键词
Model selection, Log-linear models, Item response theory
AI 理解论文
溯源树
样例
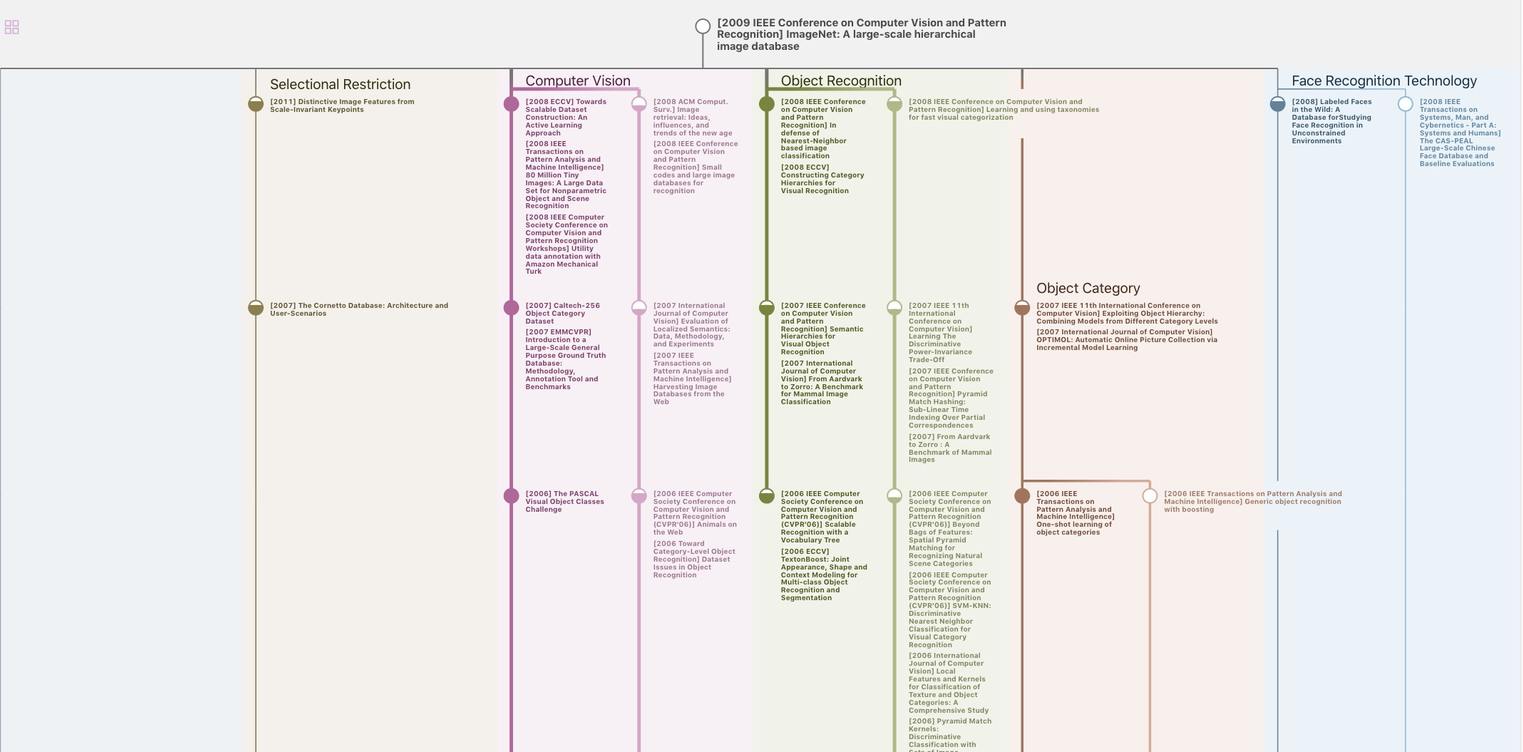
生成溯源树,研究论文发展脉络
Chat Paper
正在生成论文摘要