Manifestation Location-based Classification of Uncertainty Factors Considering Characteristics of System-of-Systems
KIISE Transactions on Computing Practices(2020)
AI 理解论文
溯源树
样例
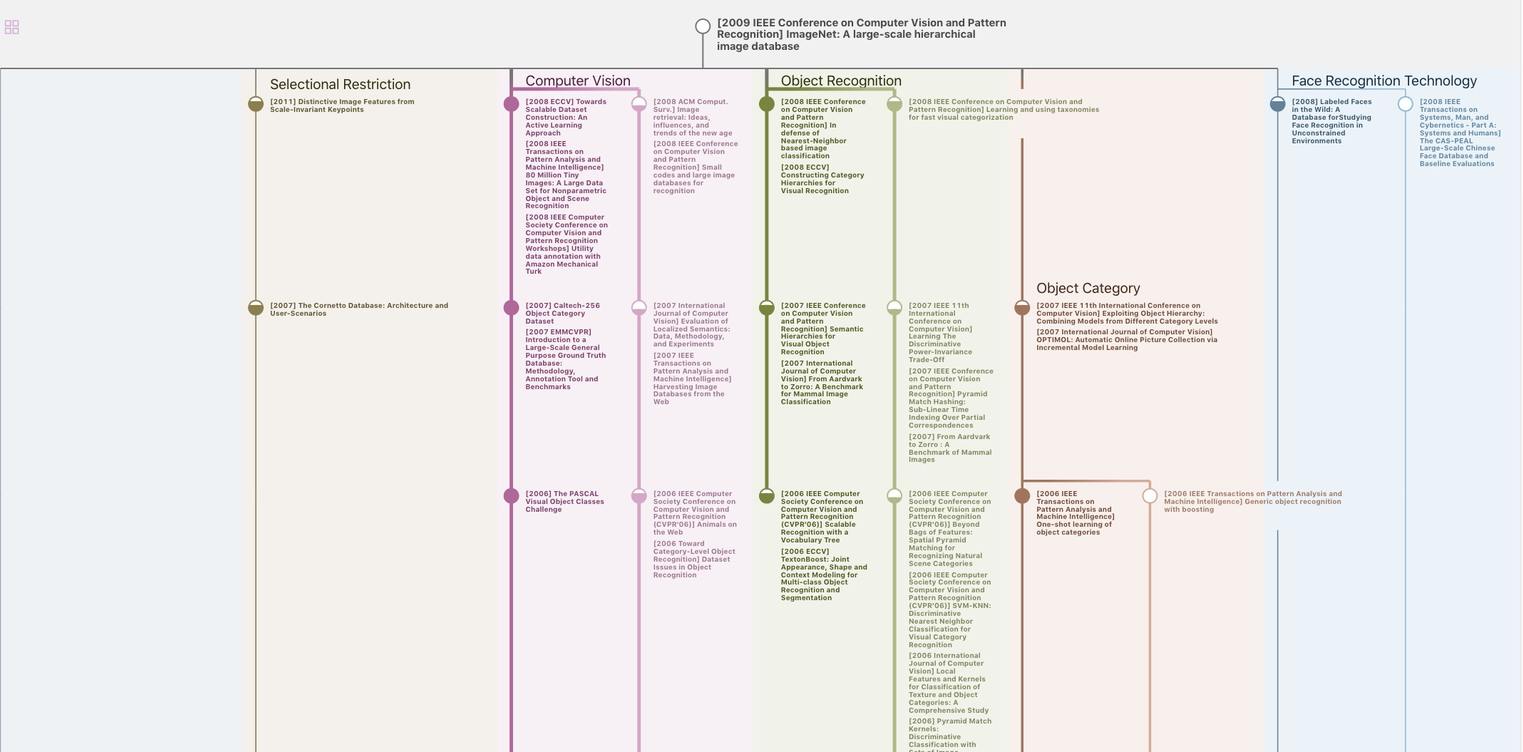
生成溯源树,研究论文发展脉络
Chat Paper
正在生成论文摘要
KIISE Transactions on Computing Practices(2020)