Machine Learning Approach to the Analysis of Traffic Management Initiatives
Journal of Air Transportation(2021)
摘要
Ground delay programs and ground stops are implemented to ensure that airport and flight operations remain safe in spite of constraint(s) at affected airports. Occasionally, ground stops are issued during ongoing ground delay programs, and vice versa, which hinders their efficient planning and implementation. Consequently, this research outlines a methodology for the analysis of their coincidence so as to identify underlying factors and better understand their occurrence. This is achieved by 1) fusing weather, ground delay program, and ground stop data; and 2) leveraging machine learning algorithms, partial dependence plots, and decision trees to assess and understand how different factors influence the coincidence of ground delay programs and ground stops. The methodology was implemented and tested using data from LaGuardia and San Francisco International Airports in order to test its robustness at airports with different weather conditions, locations, etcetera. The boosting ensemble algorithm was identified as the best-suited algorithm for both airports, and it was observed that different factors influence the occurrence of this phenomenon at both airports. This work also discusses how decision trees and partial dependence plots can be leveraged to analyze how different factors influence the coincidence of weather-related ground delay programs and ground stops.
更多查看译文
关键词
machine learning,management
AI 理解论文
溯源树
样例
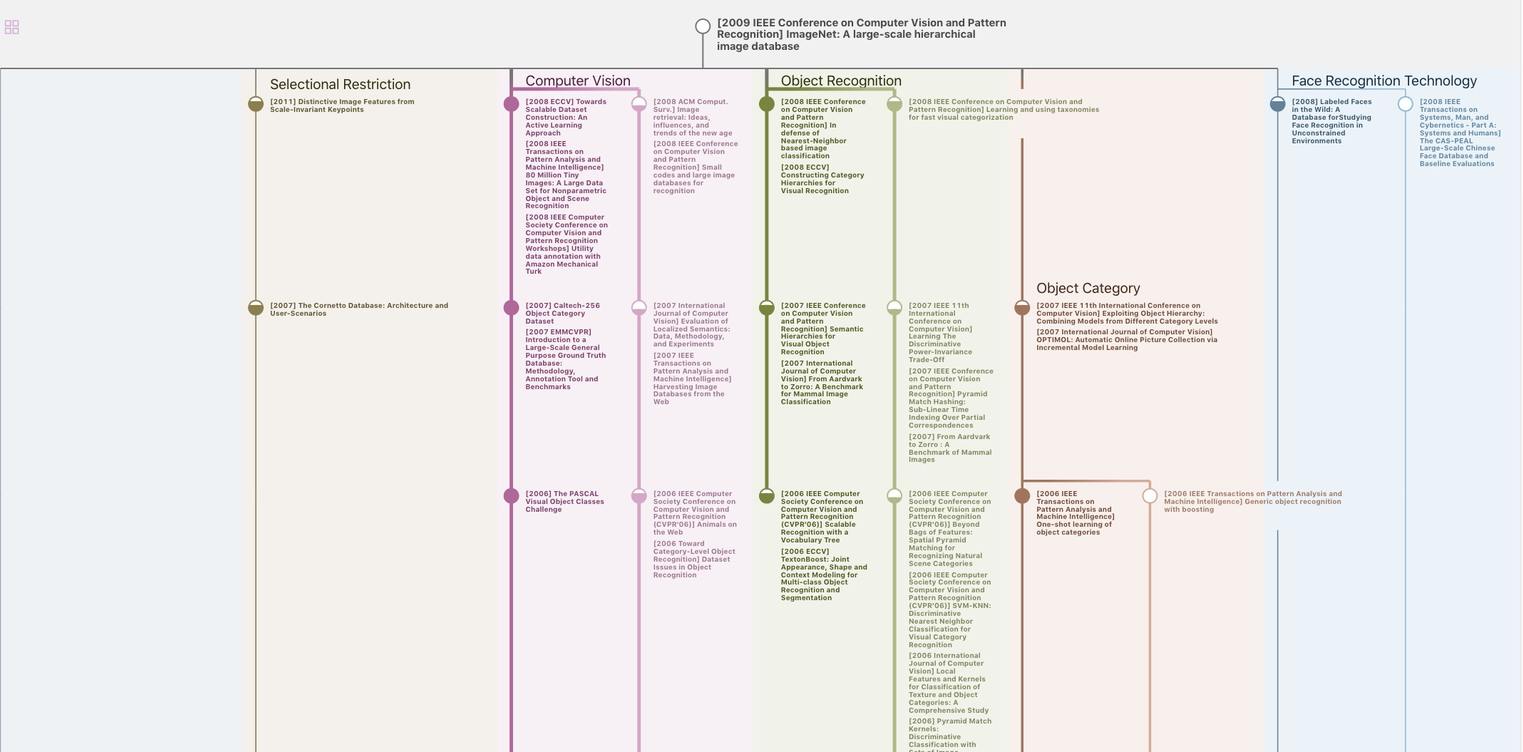
生成溯源树,研究论文发展脉络
Chat Paper
正在生成论文摘要