A new model for sparse and low-rank matrix decomposition
Journal of Applied Analysis & Computation(2017)
摘要
The robust principal component analysis (RPCA) model is a popular method for solving problems with the nuclear norm and l1 norm. However, it is time-consuming since in general one has to use the singular value decomposition in each iteration. In this paper, we introduce a novel model to reformulate the existed model by making use of low-rank matrix factorization to surrogate the nuclear norm for the sparse and low-rank decomposition problem. In such case we apply the Penalty Function Method (PFM) and Augmented Lagrangian Multipliers Method (ALMM) to solve this new nonconvex optimization problem. Theoretically, corresponding to our methods, the convergence analysis is given respectively. Compared with classical RPCA, some practical numerical examples are simulated to show that our methods are much better than RPCA.
更多查看译文
AI 理解论文
溯源树
样例
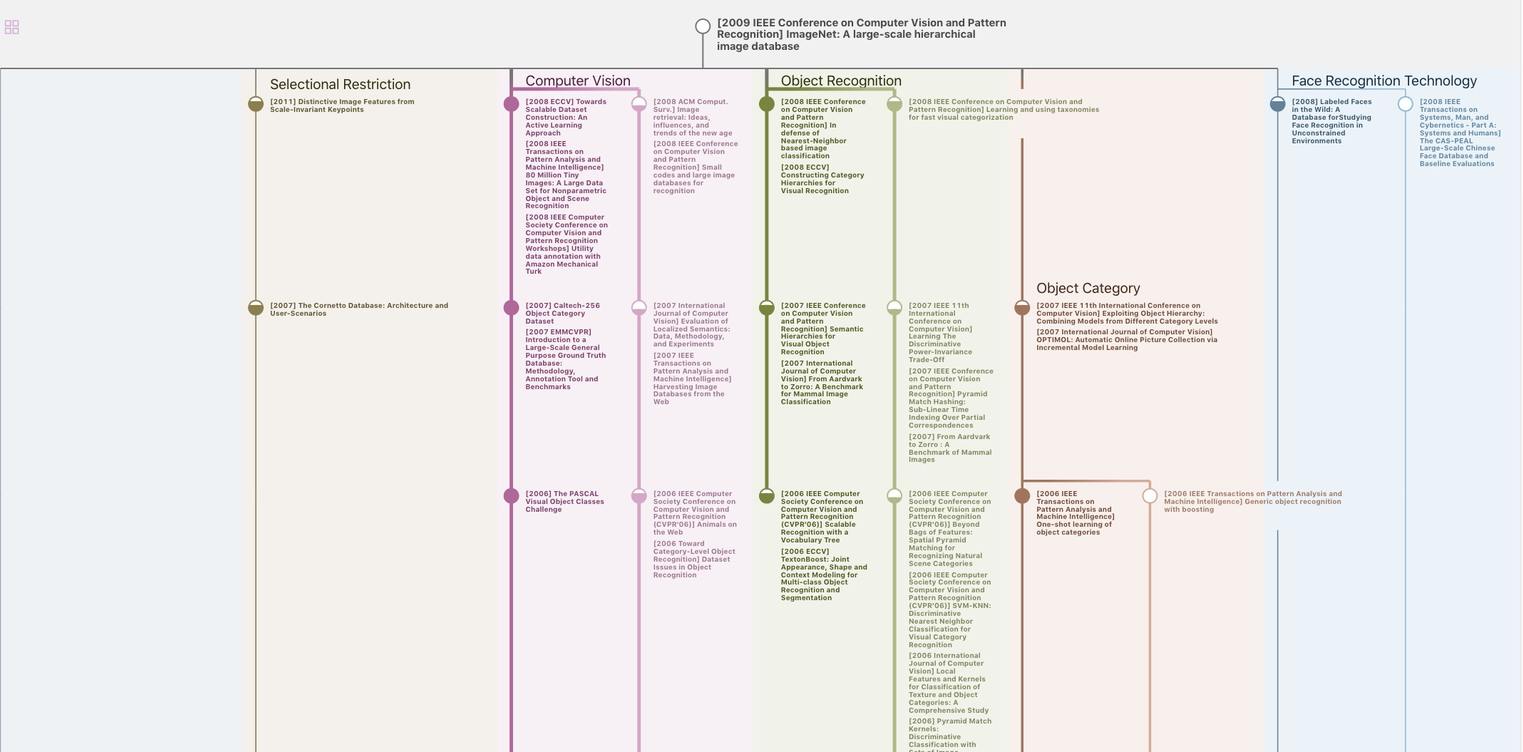
生成溯源树,研究论文发展脉络
Chat Paper
正在生成论文摘要