An adaptive prediction model for sparse data forecasting.
Int. J. Auton. Adapt. Commun. Syst.(2022)
摘要
Sparse data generated by the limitations of data acquisition are ubiquitous for prediction. However, the general prediction model is challenging to deal with those sparse data. Therefore, this paper aims to propose an adaptive sparse data prediction model. Firstly, we introduced the aXreme Gradient Boosting (XGBoost) algorithm to build an adaptive prediction model to correct sparse data constantly. Secondly, the sparsity perception of the XGBoost algorithm is used for parallel tree learning. Finally, we applied the model to the PM2.5 concentration forecasting of Nanjing, China. We trained the model and adjusted the parameters to get better prediction results, and compared the prediction results with actual data to prove the feasibility of the model.
更多查看译文
关键词
adaptive prediction model,sparse data,XGBoost algorithm,PM2.5 concentration,forecasting
AI 理解论文
溯源树
样例
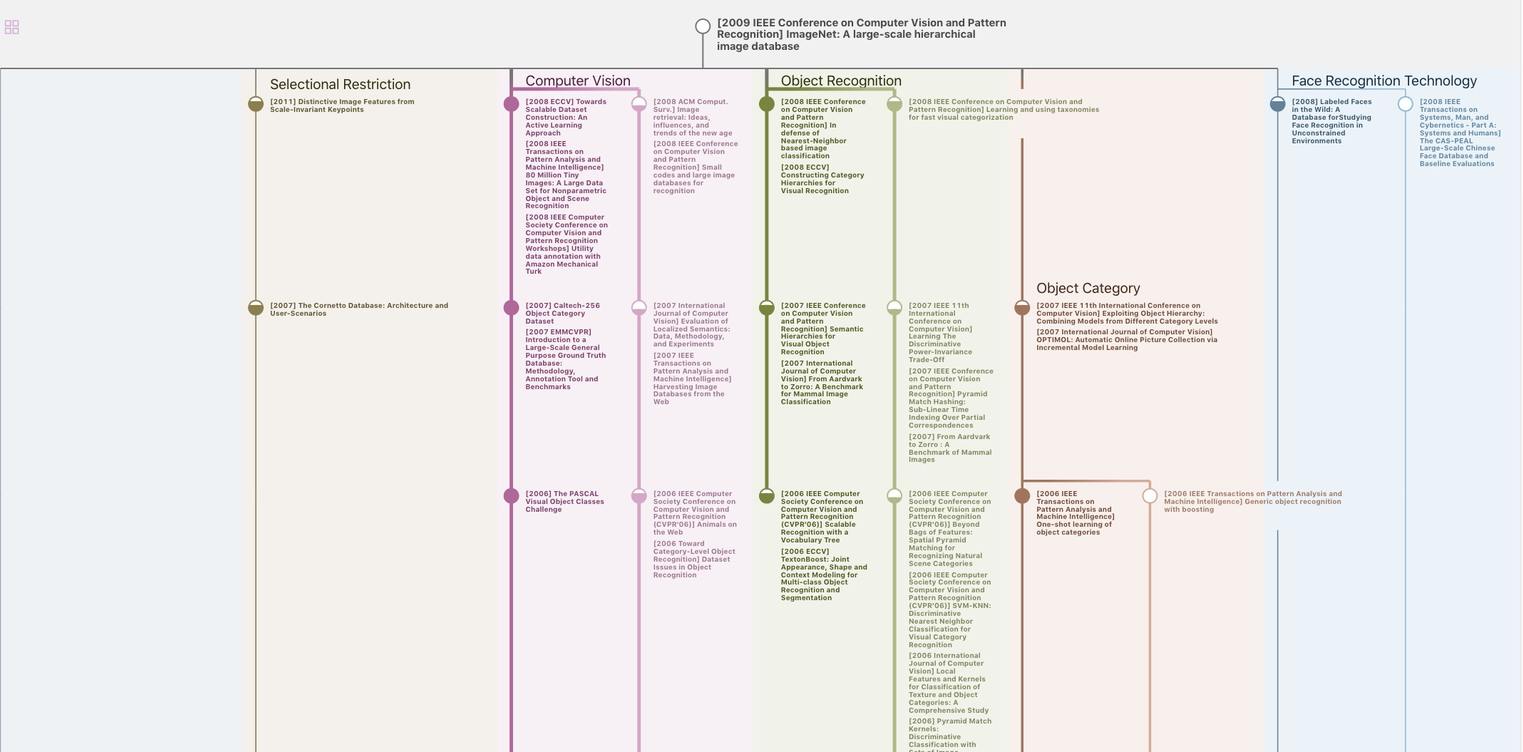
生成溯源树,研究论文发展脉络
Chat Paper
正在生成论文摘要