Explainable Artificial Intelligence for Developing Smart Cities Solutions
Smart Cities(2020)
摘要
Traditional Artificial Intelligence (AI) technologies used in developing smart cities solutions, Machine Learning (ML) and recently Deep Learning (DL), rely more on utilising best representative training datasets and features engineering and less on the available domain expertise. We argue that such an approach to solution development makes the outcome of solutions less explainable, i.e., it is often not possible to explain the results of the model. There is a growing concern among policymakers in cities with this lack of explainability of AI solutions, and this is considered a major hindrance in the wider acceptability and trust in such AI-based solutions. In this work, we survey the concept of 'explainable deep learning' as a subset of the 'explainable AI' problem and propose a new solution using Semantic Web technologies, demonstrated with a smart cities flood monitoring application in the context of a European Commission-funded project. Monitoring of gullies and drainage in crucial geographical areas susceptible to flooding issues is an important aspect of any flood monitoring solution. Typical solutions for this problem involve the use of cameras to capture images showing the affected areas in real-time with different objects such as leaves, plastic bottles etc., and building a DL-based classifier to detect such objects and classify blockages based on the presence and coverage of these objects in the images. In this work, we uniquely propose an Explainable AI solution using DL and Semantic Web technologies to build a hybrid classifier. In this hybrid classifier, the DL component detects object presence and coverage level and semantic rules designed with close consultation with experts carry out the classification. By using the expert knowledge in the flooding context, our hybrid classifier provides the flexibility on categorising the image using objects and their coverage relationships. The experimental results demonstrated with a real-world use case showed that this hybrid approach of image classification has on average 11% improvement (F-Measure) in image classification performance compared to DL-only classifier. It also has the distinct advantage of integrating experts' knowledge on defining the decision-making rules to represent the complex circumstances and using such knowledge to explain the results.
更多查看译文
关键词
explainable AI,multi-object,coverage detection,semantic Rules,hybrid image classification,flood monitoring
AI 理解论文
溯源树
样例
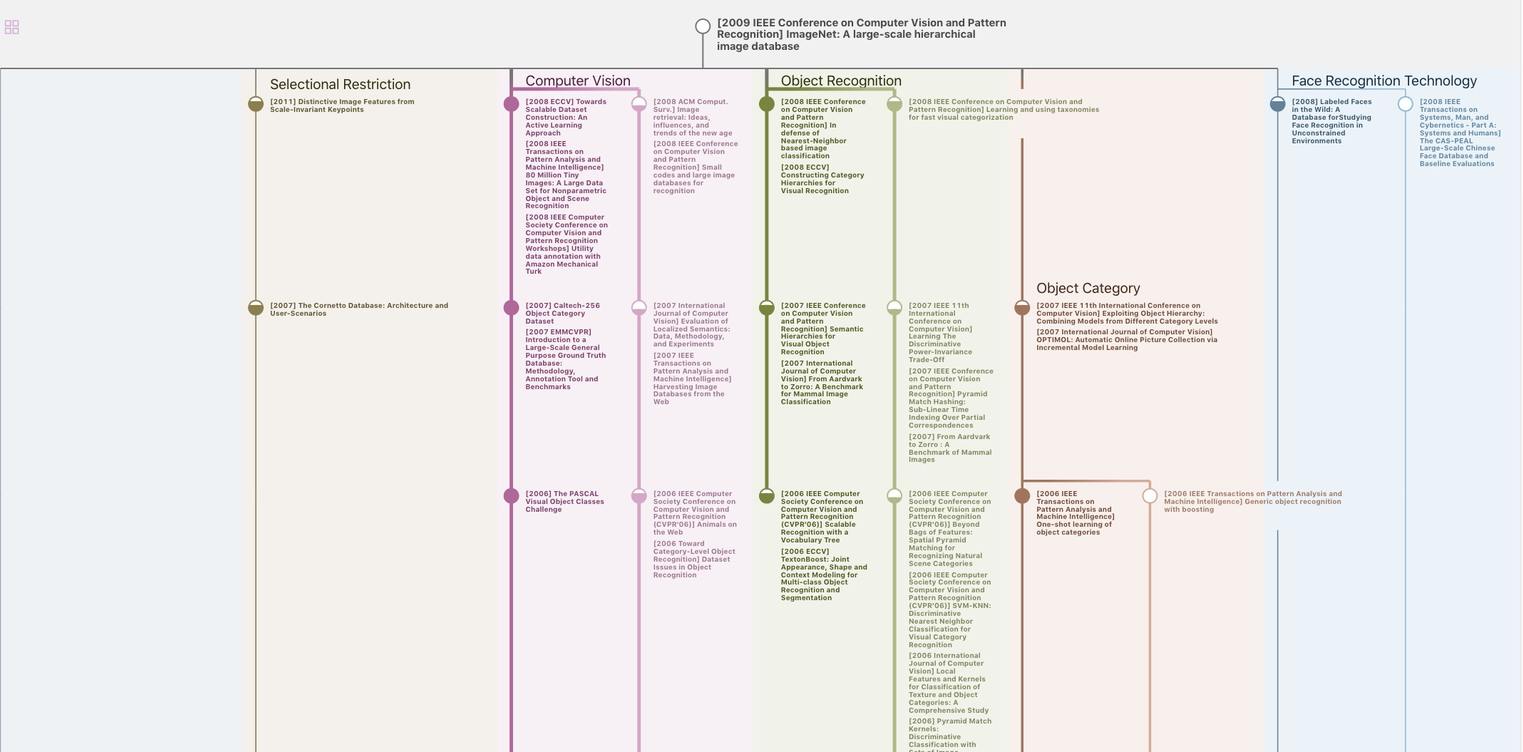
生成溯源树,研究论文发展脉络
Chat Paper
正在生成论文摘要