Parametric study of lithium-ion battery using electrochemical model in conjunction with machine learning techniques
ECS Meeting Abstracts(2020)
摘要
Lithium-ion batteries (LIBs) are widely used in consumer electronics because of its high energy density, superior rate capability, and long cycling life. Lately, the demand for LIBs in other sectors such as automobiles and grid level storage has been growing because of emphasis on renewable energy. However, performance such as rate of charging, energy density, and cost of storing energy ($/kWh) of LIBs does not meet the US-DOE targets for battery electric vehicles. Since, the LIB consists of several components like cathode, anode, electrolyte and separator, its performance is limited by the performance of individual components in the assembled LIB. Further, the performance represents the full LIB and it is difficult to isolate the performance of individual components in the assembled battery. Electrochemical modelling can be extensively used to identify the limitation and improve the performance of individual components since it is an efficient, and cost effective tool. In this work, an isothermal electrochemical model is developed for LIBs with LiC6 anode; LiPF6 based electrolyte, and LiMnO4 cathode. A porous electrode theory is used to describe the insertion and de-insertion of Li+ ions into electrode. A concentrated solution theory is used to describe the transport mechanism in the electrolyte. The Butler-Volmer kinetics is used to model reactions at the electrode/ electrolyte interface. The electrochemical model developed is solved using COMSOL Multiphysics® 5.1a finite element modelling software. The modeling results are verified with the experimental results available in the literature. The model is used to evaluate the influence of various design parameters that influence the performance of LIB such as the electrode dimensions, structure, conductivity, etc. Furthermore, the electrochemical model data was used to develop machine learning techniques such as random forests (RF), support vector machine (SVM) and gradient boosting (GB) for feature-analysis of the LIB parameters. Feature analysis, an extremely important metric for estimating relative importance of a particular parameter, were studied towards understanding the relative importance of other parameters for maximizing energy and power density of a battery. Random forest techniques performed well for predicting the energy density which is depend on thickness (after current) but gradient boosting failed to predict it (as shown in Fig.1). However, gradient boosting techniques performed well for forecasting power density which depend on conductivity (after current), here random forest failed to predict this. Without such feature-analysis by machine learning techniques, it would have been very difficult to have a quantitative estimation of their ranking towards understanding the relative importance of other battery parameters for maximizing energy and power density of LIBs. Figure 1
更多查看译文
AI 理解论文
溯源树
样例
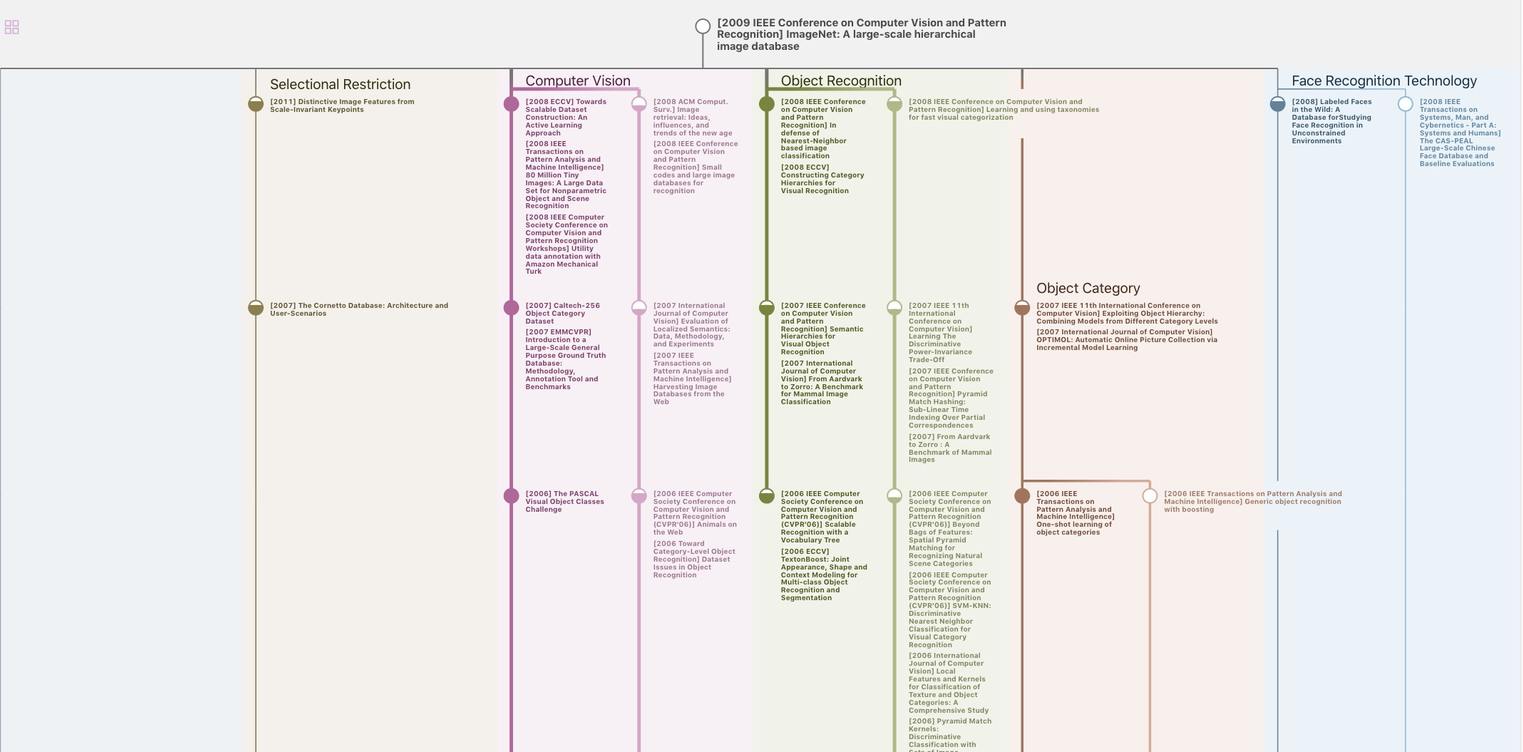
生成溯源树,研究论文发展脉络
Chat Paper
正在生成论文摘要