Evaluation of Docking Machine Learning and Molecular Dynamics Methodologies for DNA-Ligand Systems
PHARMACEUTICALS(2022)
摘要
DNA is a molecular target for the treatment of several diseases, including cancer, but there are few docking methodologies exploring the interactions between nucleic acids with DNA intercalating agents. Different docking methodologies, such as AutoDock Vina, DOCK 6, and Consensus, implemented into Molecular Architect (MolAr), were evaluated for their ability to analyze those interactions, considering visual inspection, redocking, and ROC curve. Ligands were refined by Parametric Method 7 (PM7), and ligands and decoys were docked into the minor DNA groove (PDB code: 1VZK). As a result, the area under the ROC curve (AUC-ROC) was 0.98, 0.88, and 0.99 for AutoDock Vina, DOCK 6, and Consensus methodologies, respectively. In addition, we proposed a machine learning model to determine the experimental Delta T-m value, which found a 0.84 R2 score. Finally, the selected ligands mono imidazole lexitropsin (42), netropsin (45), and N,N '-(1H-pyrrole-2,5-diyldi-4,1-phenylene)dibenzenecarboximidamide (51) were submitted to Molecular Dynamic Simulations (MD) through NAMD software to evaluate their equilibrium binding pose into the groove. In conclusion, the use of MolAr improves the docking results obtained with other methodologies, is a suitable methodology to use in the DNA system and was proven to be a valuable tool to estimate the Delta T-m experimental values of DNA intercalating agents.
更多查看译文
关键词
computer drug design, molecular docking, molecular dynamic simulation, virtual screening, MolAr, DNA intercalating agents
AI 理解论文
溯源树
样例
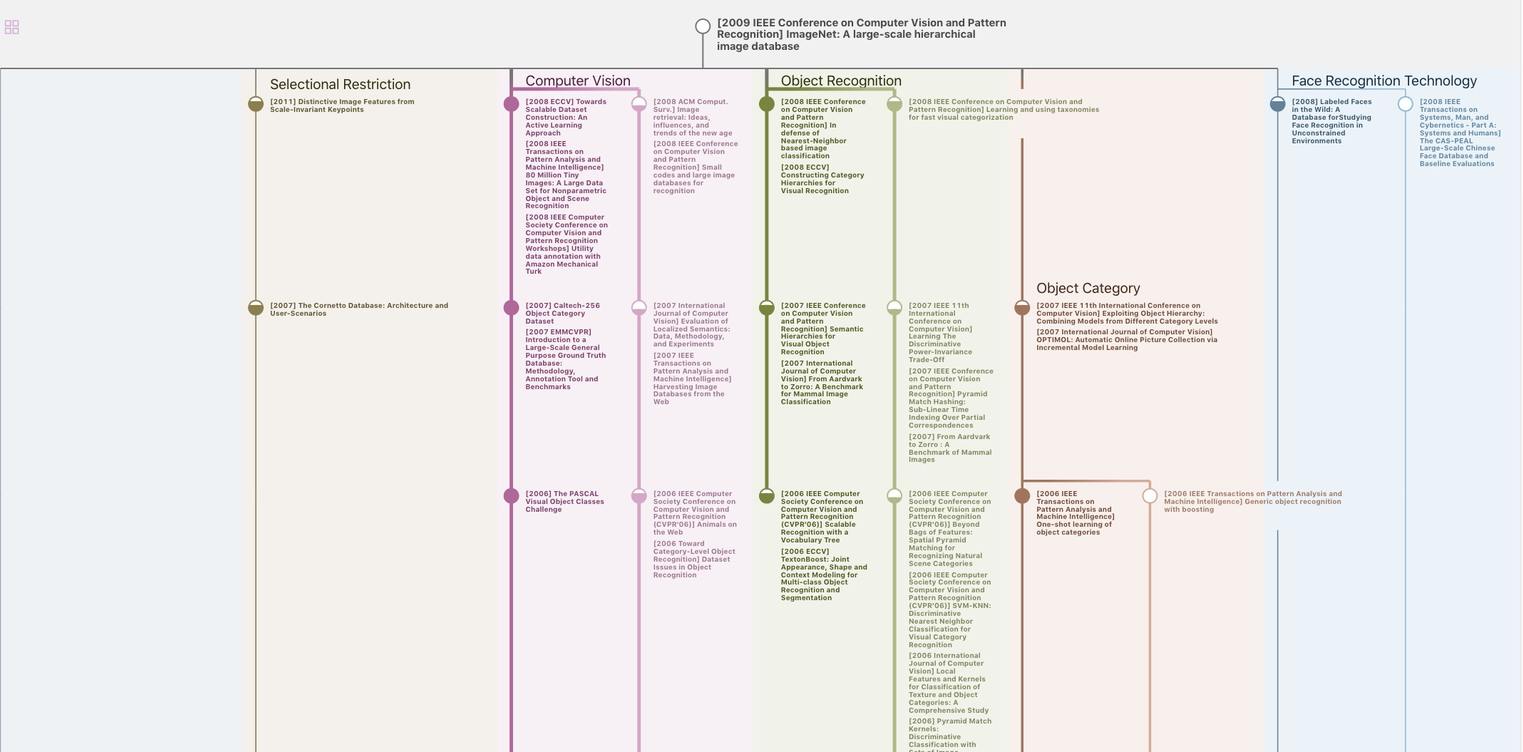
生成溯源树,研究论文发展脉络
Chat Paper
正在生成论文摘要