A Globally Convergent LP and SOCP-based algorithm for Semidefinite Programming
arXiv (Cornell University)(2022)
摘要
Semidefinite programs (SDP) are one of the most versatile frameworks in numerical optimization, serving as generalizations of many conic programs and as relaxations of NP-hard combinatorial problems. Their main drawback is their computational and memory complexity, which sets a practical limit to the size of problems solvable by off-the-shelf SDP solvers. To circumvent this fact, many algorithms have been proposed to exploit the structure of particular problems and increase the scalability of SDPs for those problem instances. Progress has been less steep, however, for general-case SDPs. In this paper, motivated by earlier results by Ahmadi and Hall, we show that a general SDP can be solved to $\epsilon$-optimality, in polynomial time, by performing a sequence of less computationally demanding Linear or Second Order Cone programs. In addition, we provide a bound on the number of iterations required to achieve $\epsilon$-optimality. These results are illustrated using random SDPs and well-known problems from the SDPLib dataset.
更多查看译文
关键词
globally convergent lp,semidefinite
AI 理解论文
溯源树
样例
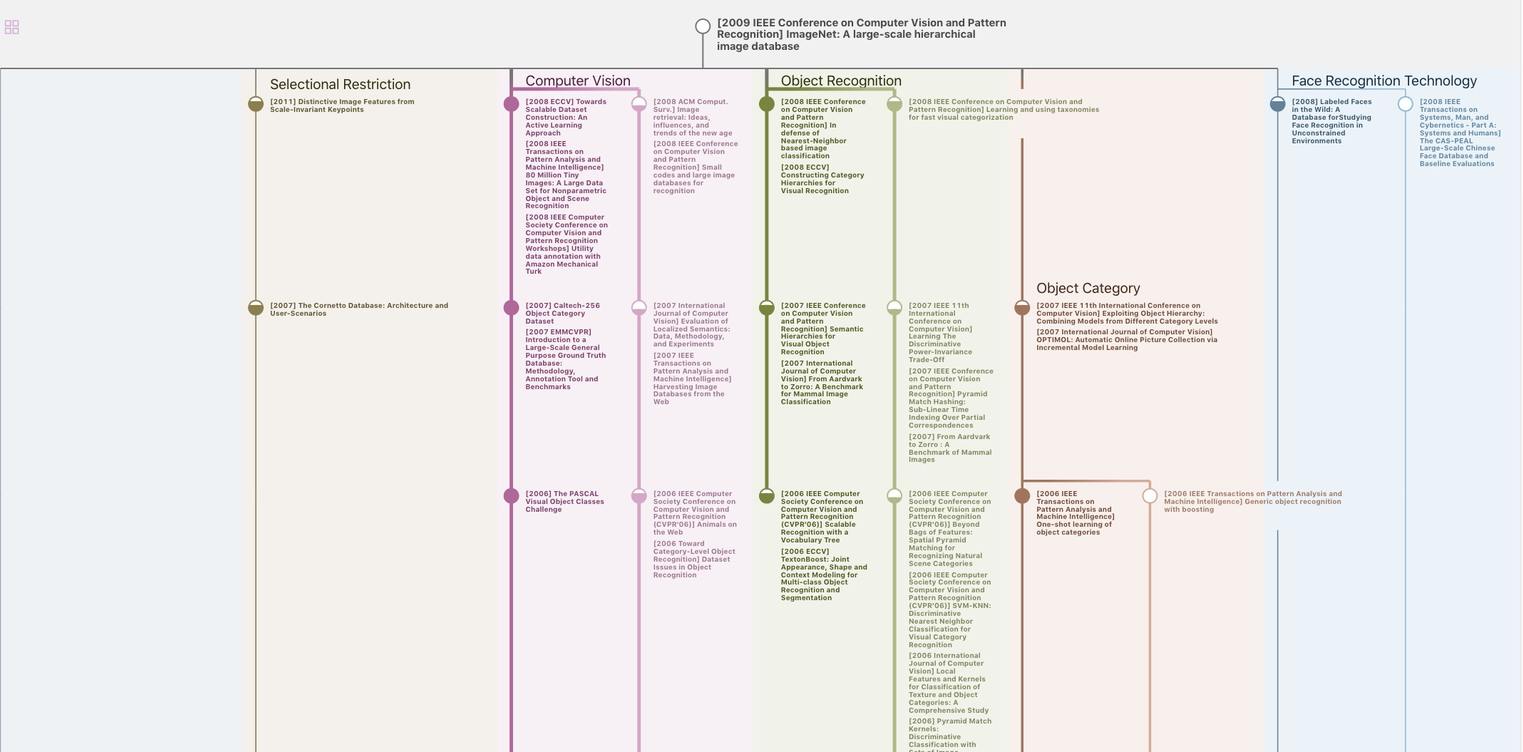
生成溯源树,研究论文发展脉络
Chat Paper
正在生成论文摘要