Confidence Calibration for Object Detection and Segmentation
arxiv(2022)
摘要
Calibrated confidence estimates obtained from neural networks are crucial, particularly for safety-critical applications such as autonomous driving or medical image diagnosis. However, although the task of confidence calibration has been investigated on classification problems, thorough in\-ves\-tiga\-tions on object detection and segmentation problems are still missing. Therefore, we focus on the investigation of confidence calibration for object detection and segmentation models in this chapter. We introduce the concept of multivariate confidence calibration that is an extension of well-known calibration methods to the task of object detection and segmentation. This allows for an extended confidence calibration that is also aware of additional features such as bounding box/pixel position, shape information, etc. Furthermore, we extend the expected calibration error (ECE) to measure mis\-ca\-li\-bra\-tion of object detection and segmentation models. We examine several network architectures on MS COCO as well as on Cityscapes and show that especially object detection as well as instance segmentation models are intrinsically miscalibrated given the introduced definition of calibration. Using our proposed calibration methods, we have been able to improve calibration so that it also has a positive impact on the quality of segmentation masks as well.
更多查看译文
关键词
confidence calibration,object detection
AI 理解论文
溯源树
样例
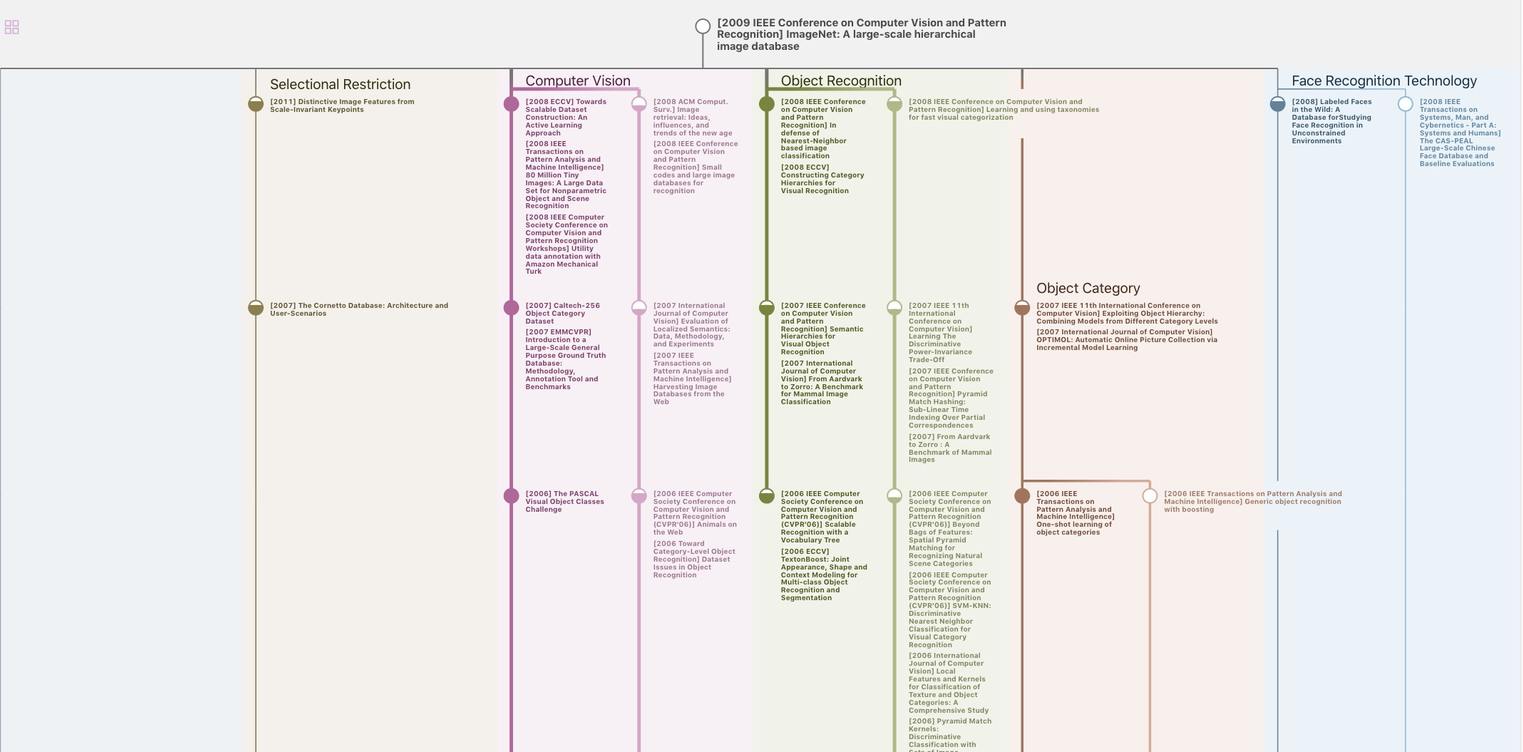
生成溯源树,研究论文发展脉络
Chat Paper
正在生成论文摘要