Explainable Artificial Intelligence for COVID-19 Diagnosis Through Blood Test Variables
Journal of Control, Automation and Electrical Systems(2022)
摘要
This work proposes an explainable artificial intelligence approach to help diagnose COVID-19 patients based on blood test and pathogen variables. Two glass-box models, logistic regression and explainable boosting machine, and two black-box models, random forest and support vector machine, were used to assess the disease diagnosis. Shapley additive explanations were used to explain predictions for the black-box models, while glass-box models feature importance brought insights into the most relevant features. All global explanations show the eosinophils and leukocytes, white blood cells are among the essential features to help diagnose the COVID-19. Moreover, the best model obtained an AUC of 0.87.
更多查看译文
关键词
COVID-19 diagnosis, Machine learning, Explainability, Interpretability, Shapley additive explanations, Explainable boosting machine
AI 理解论文
溯源树
样例
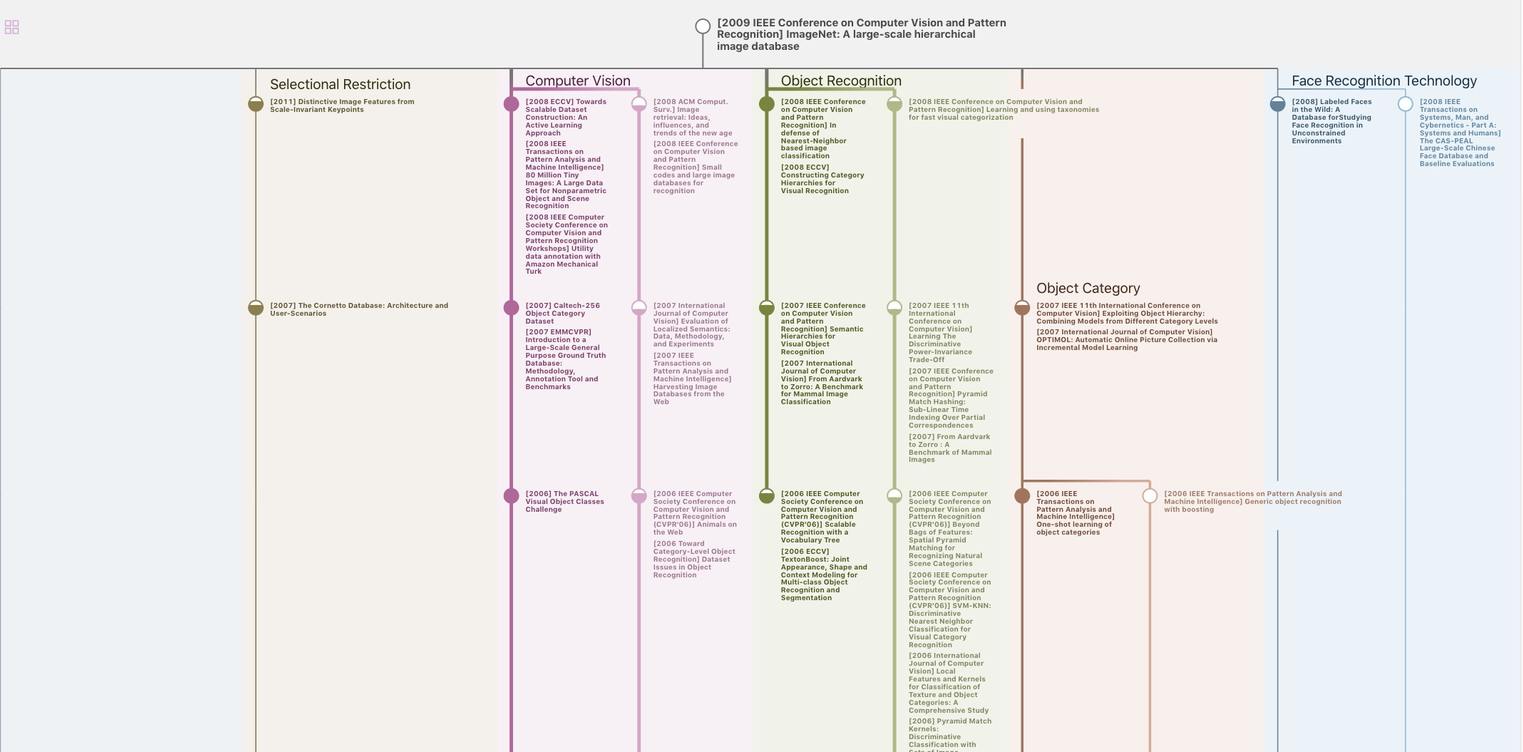
生成溯源树,研究论文发展脉络
Chat Paper
正在生成论文摘要