Neuro-Inspired Deep Neural Networks with Sparse, Strong Activations
arxiv(2022)
摘要
While end-to-end training of Deep Neural Networks (DNNs) yields state of the art performance in an increasing array of applications, it does not provide insight into, or control over, the features being extracted. We report here on a promising neuro-inspired approach to DNNs with sparser and stronger activations. We use standard stochastic gradient training, supplementing the end-to-end discriminative cost function with layer-wise costs promoting Hebbian ("fire together," "wire together") updates for highly active neurons, and anti-Hebbian updates for the remaining neurons. Instead of batch norm, we use divisive normalization of activations (suppressing weak outputs using strong outputs), along with implicit $\ell_2$ normalization of neuronal weights. Experiments with standard image classification tasks on CIFAR-10 demonstrate that, relative to baseline end-to-end trained architectures, our proposed architecture (a) leads to sparser activations (with only a slight compromise on accuracy), (b) exhibits more robustness to noise (without being trained on noisy data), (c) exhibits more robustness to adversarial perturbations (without adversarial training).
更多查看译文
关键词
neural networks,sparse,deep,neuro-inspired
AI 理解论文
溯源树
样例
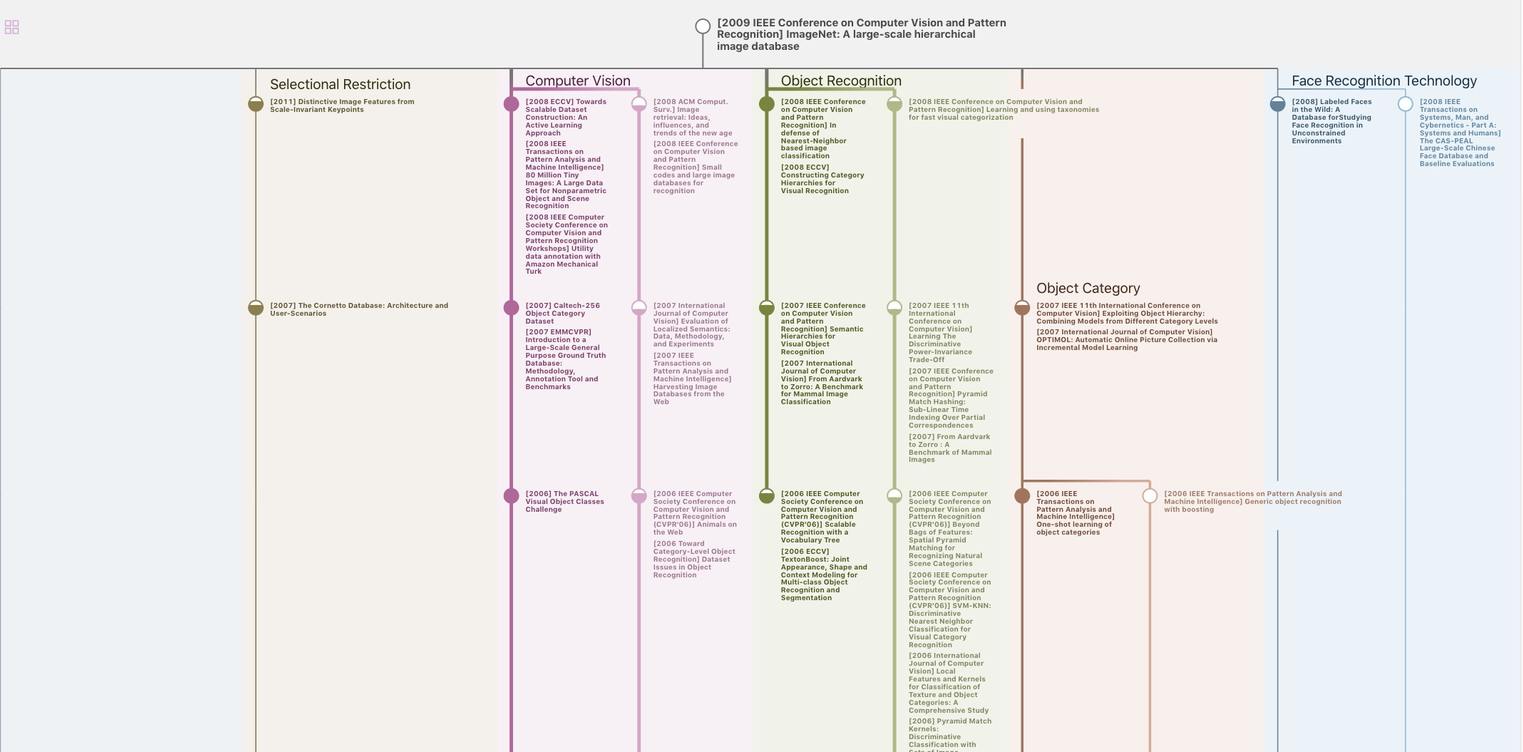
生成溯源树,研究论文发展脉络
Chat Paper
正在生成论文摘要