Metropolis Adjusted Langevin Trajectories: a robust alternative to Hamiltonian Monte Carlo
arxiv(2022)
摘要
Hamiltonian Monte Carlo (HMC) is a widely used sampler, known for its efficiency on high dimensional distributions. Yet HMC remains quite sensitive to the choice of integration time. Randomizing the length of Hamiltonian trajectories (RHMC) has been suggested to smooth the Auto-Correlation Functions (ACF), ensuring robustness of tuning. We present the Langevin diffusion as an alternative to control these ACFs by inducing randomness in Hamiltonian trajectories through a continuous refreshment of the velocities. We connect and compare the two processes in terms of quantitative mixing rates for the 2-Wasserstein and $\mathbb{L}_2$ distances. The Langevin diffusion is presented as a limit of RHMC achieving the fastest mixing rate for strongly log-concave targets. We introduce a robust alternative to HMC built upon these dynamics, named Metropolis Adjusted Langevin Trajectories (MALT). Studying the scaling limit of MALT, we obtain optimal tuning guidelines similar to HMC, and recover the same scaling with respect to the dimension without additional assumptions. We illustrate numerically the efficiency of MALT compared to HMC and RHMC.
更多查看译文
关键词
metropolis adjusted langevin trajectories,hamiltonian monte carlo,robust alternative
AI 理解论文
溯源树
样例
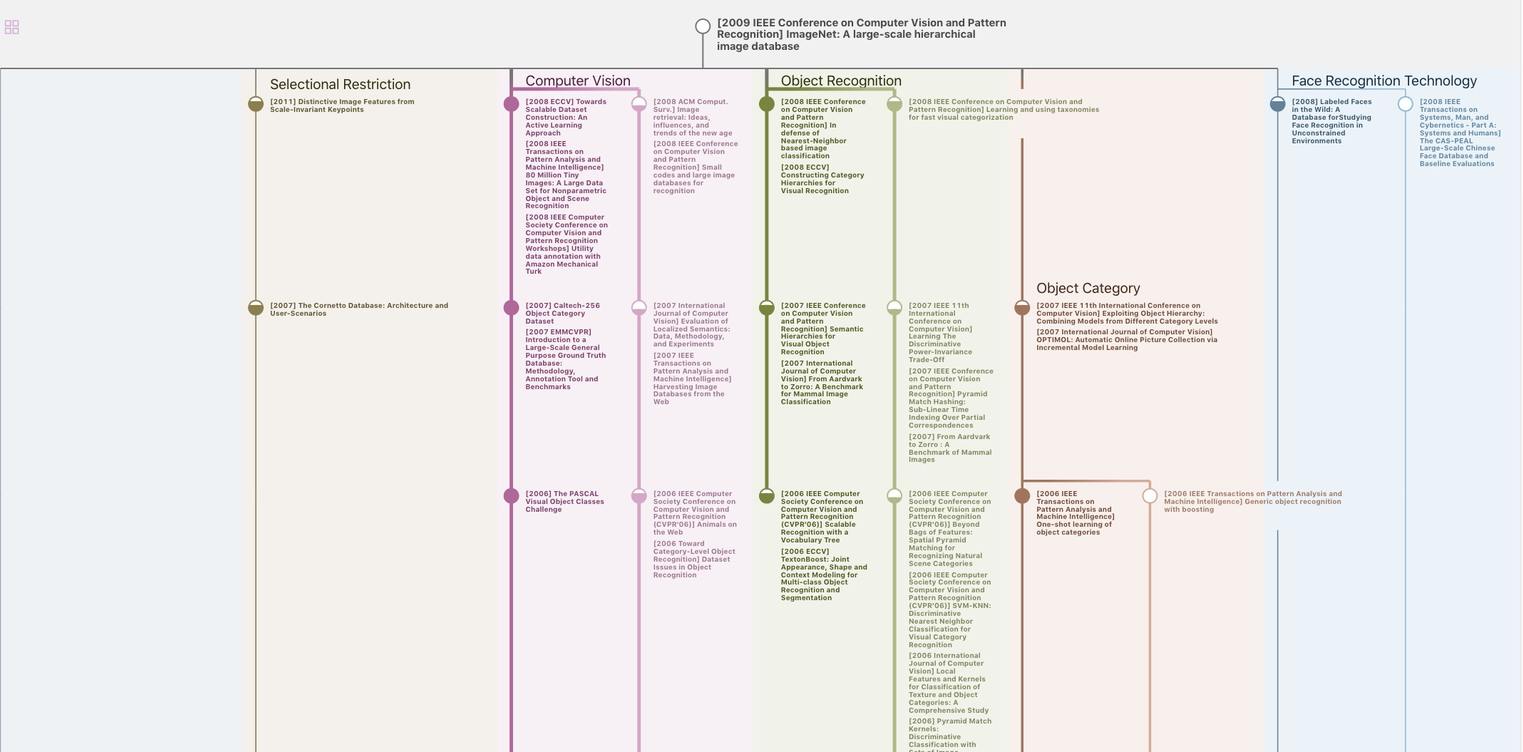
生成溯源树,研究论文发展脉络
Chat Paper
正在生成论文摘要