TEScalib: Targetless Extrinsic Self-Calibration of LiDAR and Stereo Camera for Automated Driving Vehicles with Uncertainty Analysis
IEEE/RJS International Conference on Intelligent RObots and Systems (IROS)(2022)
摘要
In this paper, we present TEScalib, a novel extrinsic self-calibration approach of LiDAR and stereo camera using the geometric and photometric information of surrounding environments without any calibration targets for automated driving vehicles. Since LiDAR and stereo camera are widely used for sensor data fusion on automated driving vehicles, their extrinsic calibration is highly important. However, most of the LiDAR and stereo camera calibration approaches are mainly target-based and therefore time consuming. Even the newly developed targetless approaches in last years are either inaccurate or unsuitable for driving platforms. To address those problems, we introduce TEScalib. By applying a 3D mesh reconstruction-based point cloud registration, the geometric information is used to estimate the LiDAR to stereo camera extrinsic parameters accurately and robustly. To calibrate the stereo camera, a photometric error function is builded and the LiDAR depth is involved to transform key points from one camera to another. During driving, these two parts are processed iteratively. Besides that, we also propose an uncertainty analysis for reflecting the reliability of the estimated extrinsic parameters. Our TEScalib approach evaluated on the KITTI dataset achieves very promising results.
更多查看译文
关键词
lidar,automated driving vehicles,uncertainty analysis,self-calibration
AI 理解论文
溯源树
样例
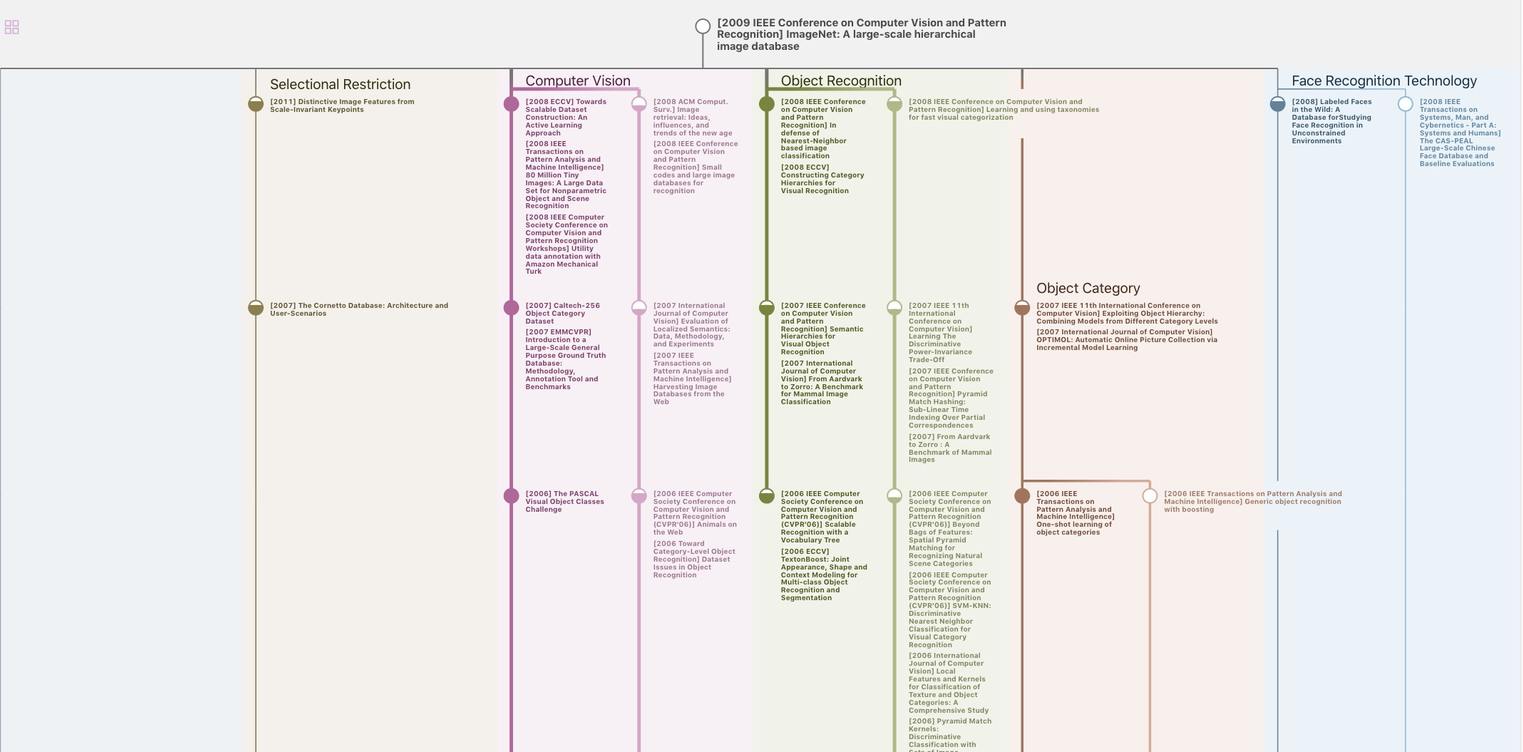
生成溯源树,研究论文发展脉络
Chat Paper
正在生成论文摘要