Spatio-Causal Patterns of Sample Growth
CoRR(2023)
摘要
Different statistical samples (e.g., from different locations) offer populations and learning systems observations with distinct statistical properties. Samples under (1) 'Unconfounded' growth preserve systems' ability to determine the independent effects of their individual variables on any outcome-of-interest (and lead, therefore, to fair and interpretable black-box predictions). Samples under (2) 'Externally-Valid' growth preserve their ability to make predictions that generalize across out-of-sample variation. The first promotes predictions that generalize over populations, the second over their shared exogeneous factors. We illustrate these theoretic patterns in the full American census from 1840 to 1940, and samples ranging from the street-level all the way to the national. This reveals sample requirements for generalizability over space, and new connections among the Shapley value, U-Statistics (Unbiased Statistics), and Hyperbolic Geometry.
更多查看译文
关键词
sample growth,patterns,spatio-causal
AI 理解论文
溯源树
样例
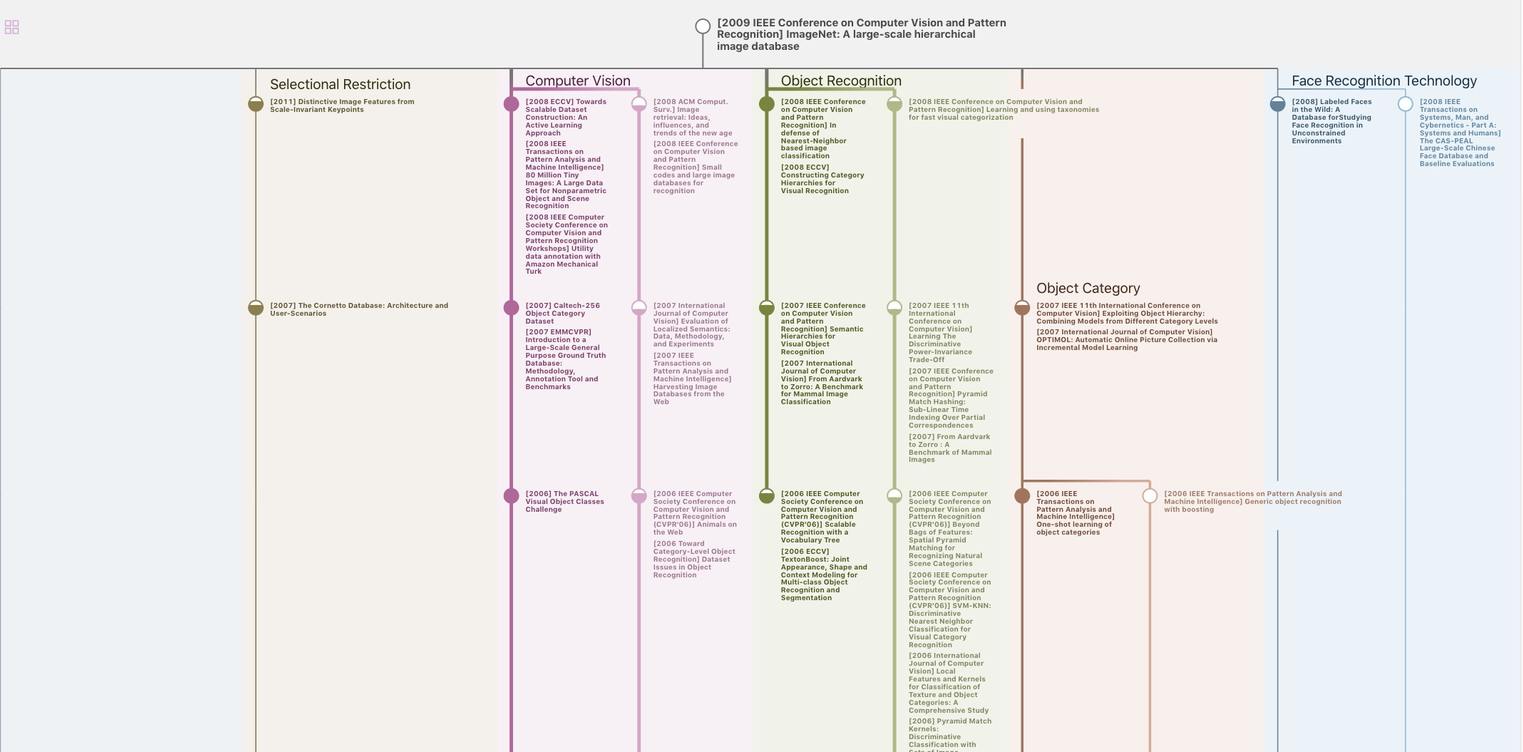
生成溯源树,研究论文发展脉络
Chat Paper
正在生成论文摘要