Model Reduction of Geometrically Nonlinear Structures ViaPhysics-Informed Autoencoders
Conference proceedings of the Society for Experimental Mechanics(2021)
摘要
High-fidelity finite element (FE) models are widely used to simulate the dynamic response of geometrically nonlinear structures. The high computational cost of running long-time-duration analyses; however, it has made nonlinear reduced-order models (ROMs) an attractive alternative. While there are a variety of reduced-order modeling techniques, in general, their shared goal is to project the nonlinear response of the system onto a smaller number of degrees of freedom (DOFs). For linear structures, modal-based projection methods are widely used for model reduction. This general procedure is akin to the dimensionality reduction that occurs when transforming data with autoencoders (AE), a machine learning technique used in unsupervised learning to extract representational features by restricting the capacity of the latent space. This idea is exploited to explore the use of AEs to perform dimensionality reduction of high-fidelity FE models and learn a latent space-based ROM. A physics-informed loss function is included to improve the network’s performance and enhance interpretability. The approach is demonstrated by performing direct time integration of the equations of motion in latent space for a flat beam with geometric nonlinearity.
更多查看译文
关键词
Nonlinear dynamics, Reduced order models, Geometric nonlinearity, Machine learning, Unsupervised learning
AI 理解论文
溯源树
样例
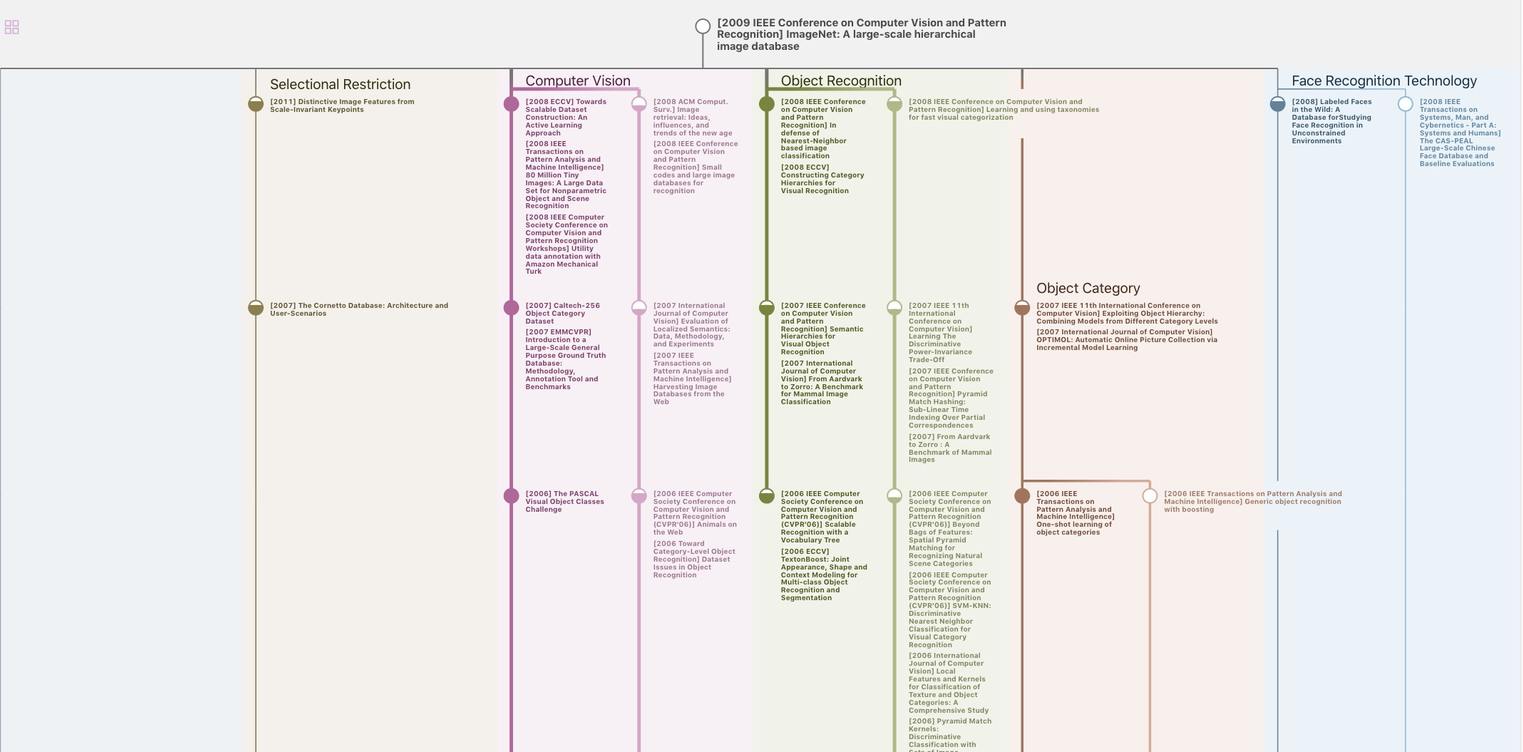
生成溯源树,研究论文发展脉络
Chat Paper
正在生成论文摘要