Similarity Analytics for Semantic Text Using Natural Language Processing
EAI/Springer Innovations in Communication and Computing(2022)
摘要
Determining the similarity among the sentences is a predominant task in natural language processing. The semantic determining task is one of the important research area in today’s applications related to text analytics. The semantic of the sentences get varied according to the textual context it is used. In natural language processing, determining the semantic likeness between sentences is an important research area. As a result, a lot of research is done in determining the semantic likeness in the text. For example, there exists many possible semantics for a word (polysemy) and the synonym of the word; and also these techniques avoid considering the stop words in English which are critical for English phrase/word division, speech investigation, and meaningful comprehension. Our proposed work utilizes Term Frequency-based Inverse Document Frequency model and Glove algorithm-based word embeddings vector for determining the semantic similarity among the terms in the textual contents. Lemmatizer is utilized to reduce the terms to the most possible smallest lemmas. The outcomes demonstrate that the proposed methodology is more prominent than the TF-idf score in ranking the terms with respect to the search query terms. The Pearson correlation coefficient achieved for the semantic similarity model is 0.875.
更多查看译文
关键词
Term Frequency, Lemmatizer, Tokenizer, Semantic similarity, Word embeddings
AI 理解论文
溯源树
样例
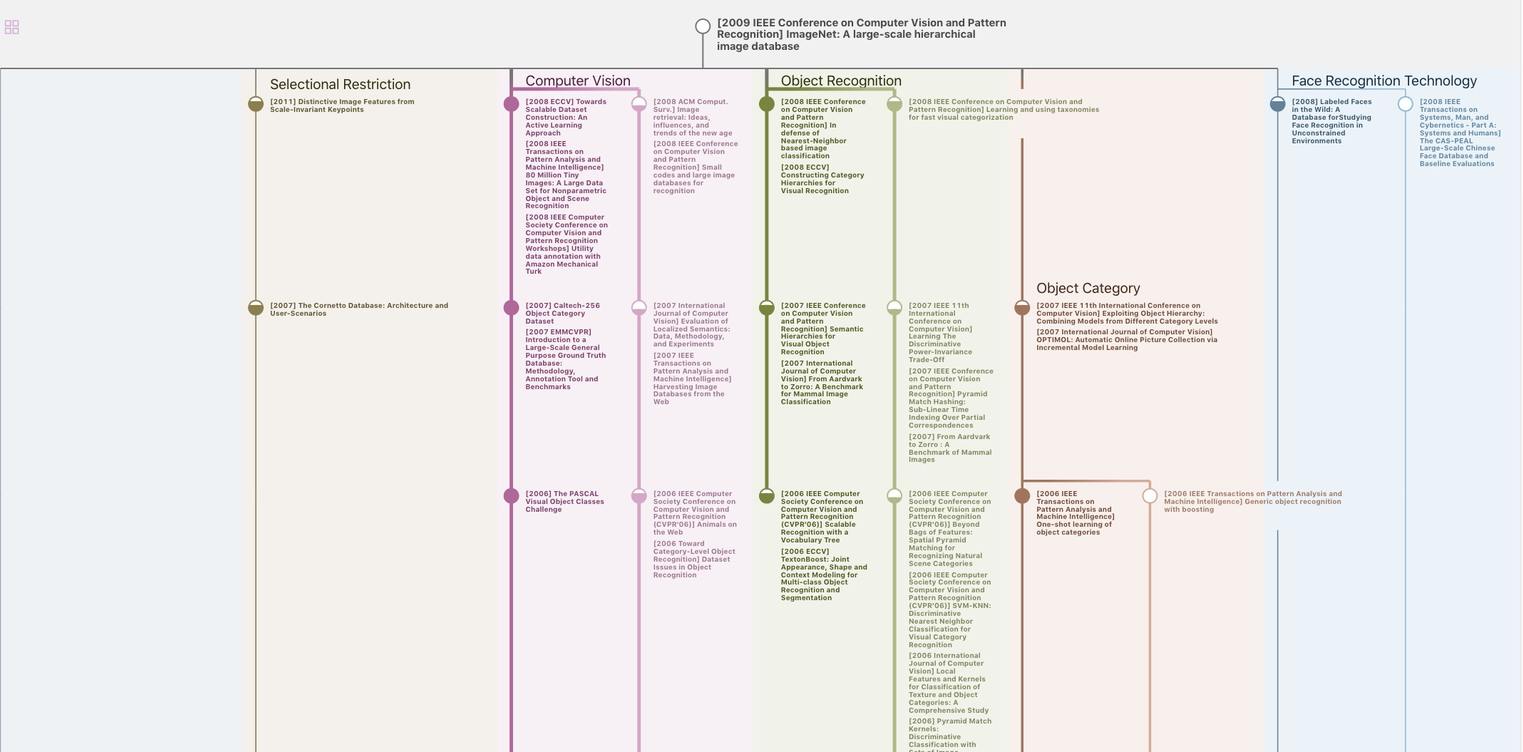
生成溯源树,研究论文发展脉络
Chat Paper
正在生成论文摘要