Annual Rainfall Prediction Using Artificial Neural Networks
crossref
摘要
The hydrological cycle depends primarily on rainfall. It is of utmost importance to measure and predict accurately the spatial and temporal distribution of rainfall for countries flourishing agricultural growth. However, developing and implementing rainfall predictive models is one of the most challenging problems due to its highly nonlinear characteristics. The prediction of rainfall has been observed to deviate from the real data because of dependence on a large number of complex parameters and involving high uncertainty. Artificial neural network (ANN) is a pioneering approach, which facilitates a computationally intelligent system to possess humanlike expertise, adapt itself, and attempt to acquire to do better in varying environments so that decisions become useful for planning and management. Unlike conventional artificial intelligence techniques, the guiding principle of soft computing such as ANN is to achieve tolerance for inaccuracy, uncertainty, robustness, and partial truth to realize tractability and a better understanding of reality. Rainfall is one of nature’s greatest gifts which has become even more important in the states like Rajasthan which has historically been a water-deficient state with only 1% of the country’s water resources available in 10.4% geographical area. It is thus a major concern to identify any trends for rainfall and predict it accordingly so that it would give greater insight among the people and would help the planners, administration, technicians, researchers, and NGOs engaged in the decision-making process of water conservation to make sustainable development and management. Therefore, this paper deals with a case study of annual rainfall prediction in the Chittorgarh district of Rajasthan by taking 53 years of historical rainfall data of Chittorgarh for training the ANN. The ANN has also been validated with another set of data of 13 years. The transfer function used for all three layers of ANN was the radial basis transfer function (RADBAS). The number of iterations involved in the process has been taken as 100,000 which finally leads to achieving a mean square error of 0.005 during the training process of the normalized data. The current manuscript presents the comparison of the network predicted outputs with the actual rainfall data. The results depict that the proposed network model can efficiently scale to other parts of the country and can prove to be a great asset.
更多查看译文
关键词
Artificial neural network, Seasonal rainfall forecast
AI 理解论文
溯源树
样例
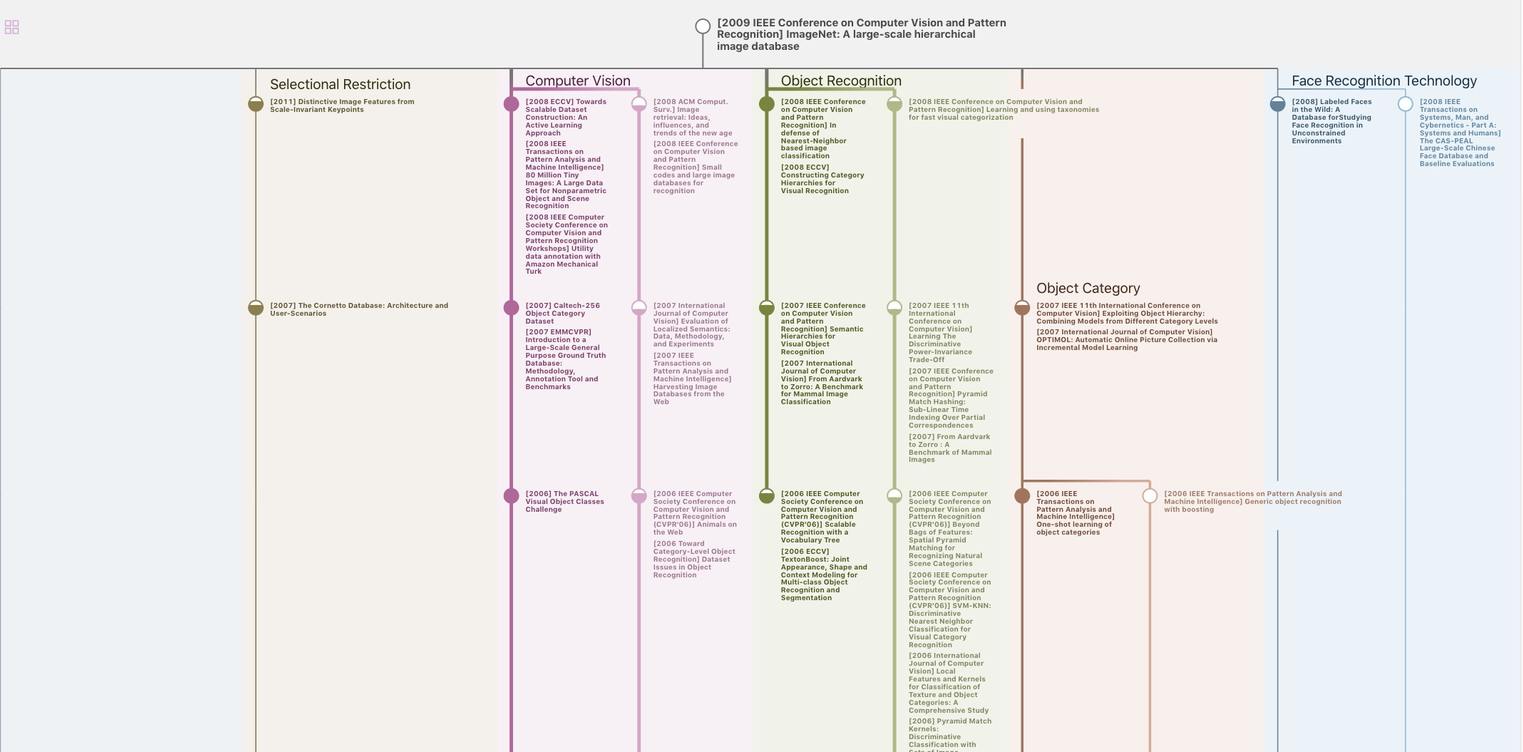
生成溯源树,研究论文发展脉络
Chat Paper
正在生成论文摘要