Adaptive Chromosome Diagnosis Based on Scaling Hierarchical Clusters
ADVANCES IN ARTIFICIAL INTELLIGENCE AND APPLIED COGNITIVE COMPUTING(2021)
摘要
In this study, we present how to divide chromosome data sets into scalable hierarchical clusters. Diagnosis applications of chromosomal abnormalities to identify genetic diseases are mostly implemented with semi-automatic methods, which do not provide high-throughput and fast characterizations of patient specimens. However, selecting and managing specific features of chromosome data sets require dynamic and scalable data models. Here, we adapt the variations in sets to feature units as an effective tool for our dynamic/automated and scalable approach. This method enables geneticists to implement karyotyping techniques easily into an efficient identification of hereditary disorders or characterization of novel chromosomal alterations related with genetic diseases in a trusted/scalable manner. Furthermore, we explore physical limits of available application-specific integrated circuits (ASICs) and on-board computers (OBCs) to extract novel features of chromosomes via spectral analytics for real-time diagnosis and to overcome the bottlenecks of the computational complexity, as well.
更多查看译文
关键词
Distributed computing, Trusted computing, Genetic diseases, Chromosome diagnostic, Trusted AI, Hierarchical neural networks, Machine learning, Deep learning, Markovian chain Monte Carlo
AI 理解论文
溯源树
样例
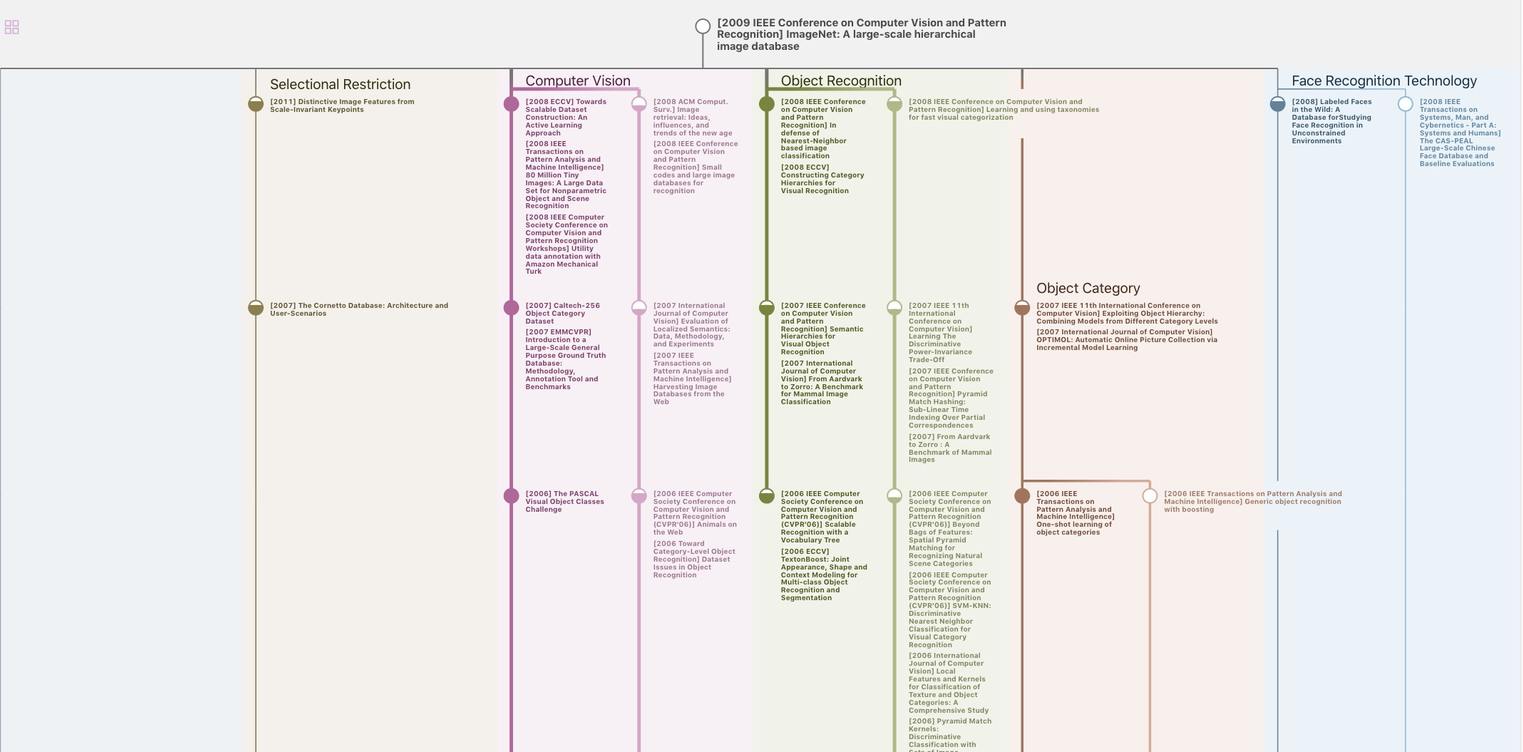
生成溯源树,研究论文发展脉络
Chat Paper
正在生成论文摘要