Machine Learning in the Diagnosis of Disorders of Consciousness: Opportunities and Challenges
Advances in Cognitive Research, Artificial Intelligence and NeuroinformaticsAdvances in Intelligent Systems and Computing(2021)
摘要
The detection of conscious awareness in patients with disorders of consciousness using behavioral signs is challenging in the presence of sensory, motor, or executive function deficits. Brain signal measurements provide additional information for patient stratification, and the large amounts and multidimentional nature of the obtained data motivate the application of machine learning (ML) methods. We review some of the recent studies applying this approach to data from electroencephalography, diffusion magnetic resonance imaging (MRI), and resting-state functional MRI. Each of these modalities provided features correlating with the behaviorally determined levels of consciousness. At the same time, considering the accuracy of the obtained classification algorithms, we highlight the problem of simultaneous model selection and accuracy estimation using the same sample, which requires special techniques of statistical analysis, otherwise the obtained accuracy estimates can be biased and prone to misinterpretation. Additionally, there is a need for greater generalization ability of the classifiers over the whole spectrum from the UWS to the conscious state. The use of ML within brain-computer interfaces for the detection of command following is discussed as a promising complementary method able to detect patients with cognitive motor dissociation, and its results may prove useful as labels in the training of algorithms for the direct stratification of DOC patients.
更多查看译文
关键词
Disorders of consciousness,Diagnosis,Unresponsive wakefulness syndrome,Minimally conscious state,Machine learning,Cross-validation,Brain-computer interface
AI 理解论文
溯源树
样例
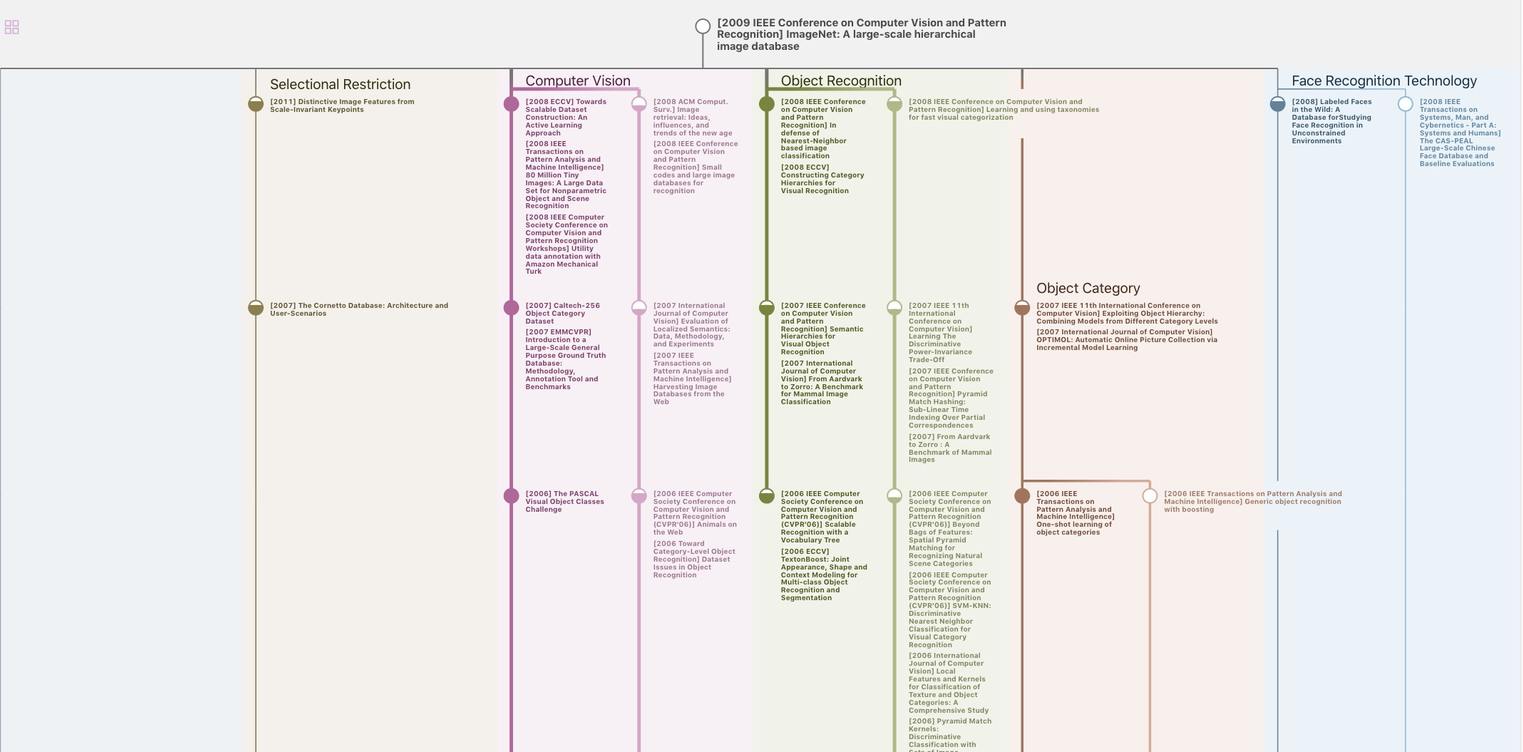
生成溯源树,研究论文发展脉络
Chat Paper
正在生成论文摘要