Privacy-Preserving Scheme Against Inference Attack in Social Networks
springer
摘要
Social networks analytics provide enormous business values for organizational and societal growth. Massive volumes of social network data get collected in every moment and released to other parties for various business objectives. The collected data play an important role in designing policies, plans and future projection of business strategies. These social network data carry sensitive information, and therefore, the adversary can exploit users profile and social relationships to disclose their privacy. Inference attack using the mining technique poses a crucial concern for privacy leakage in social networks. In this paper, we present a privacy-preserving scheme against inference attack. The proposed scheme adds spurious data in the published dataset such that sensitive information is not predicted using mining techniques. The proposed scheme is analyzed against a strong adversarial model, where an adversary is allowed to gather background knowledge from different sources. We have experimented the proposed scheme on real-social network dataset and the experimental results show that the privacy-preserving property of the proposed scheme outperforms in comparison with other related schemes.
更多查看译文
关键词
Social networks, Data privacy, Inference attack, Background knowledge
AI 理解论文
溯源树
样例
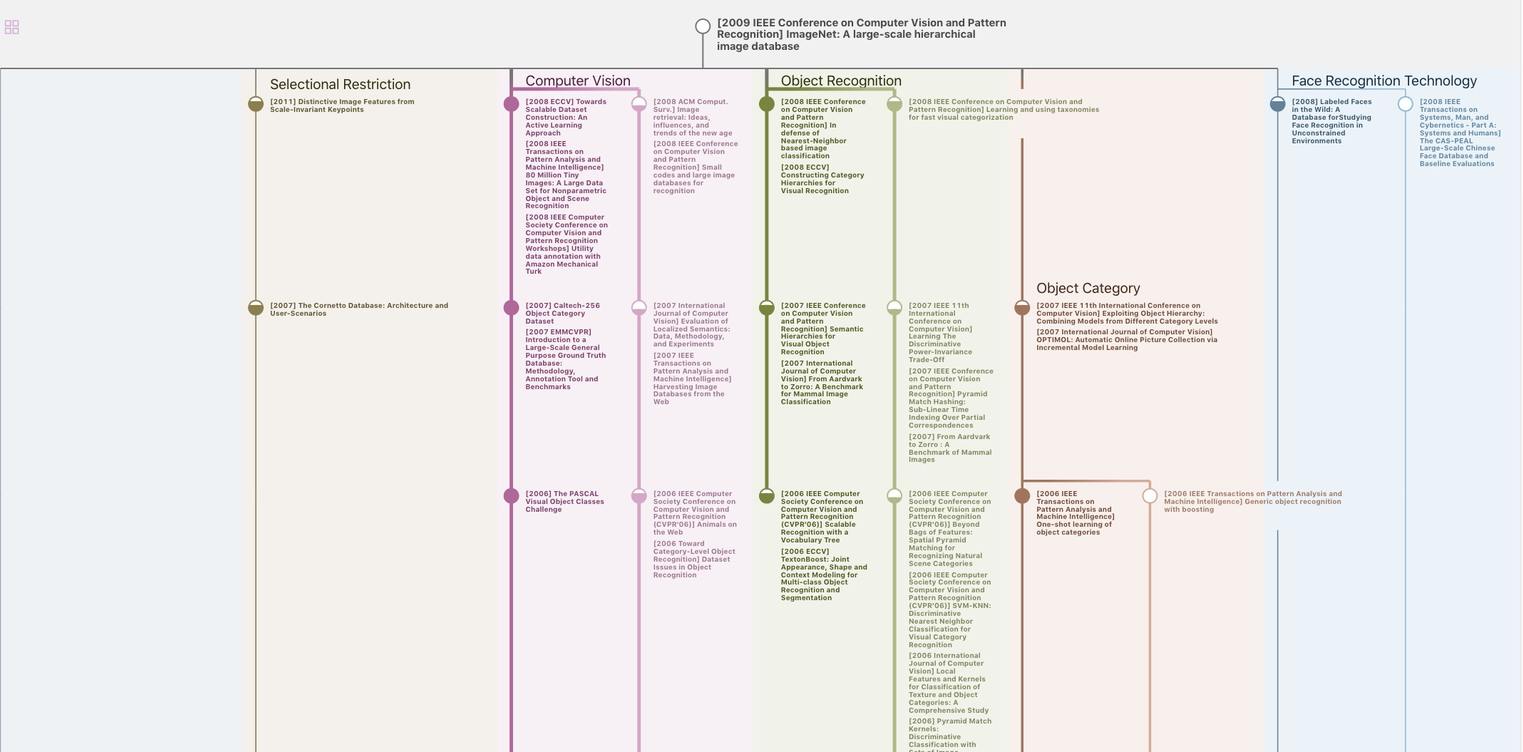
生成溯源树,研究论文发展脉络
Chat Paper
正在生成论文摘要