SMOTE–ENN-Based Data Sampling and Improved Dynamic Ensemble Selection for Imbalanced Medical Data Classification
Advances in intelligent systems and computing(2020)
摘要
During the last few years, the classification of imbalanced datasets is one of the crucial issues in medical diagnosis since it is related to the distribution of normal and abnormal cases which can potentially affect the performance of the diagnosis system. For solving this problem, various techniques have been designed in order to achieve acceptable quality. Ensemble systems are one of those techniques, and they have proven their ability to be more accurate than single classifier models. Classifier selection is related to the choice of an optimal subset within a pool of classifiers. Selection of classifier can be broadly split into two classes: static and dynamic. This paper proposes a novel set selection scheme for the classification of imbalanced medical datasets. The suggested approach is based on the combination of an improved dynamic ensemble selection called META-DES framework combined with a hybrid sampling method called SMOTE–ENN. The experimental results prove the superiority of the proposed ensemble learning system using three UCI datasets.
更多查看译文
关键词
Imbalanced dataset, Medical data classification, Ensemble learning, Smote–ENN, META-DES, Dynamic ensemble selection
AI 理解论文
溯源树
样例
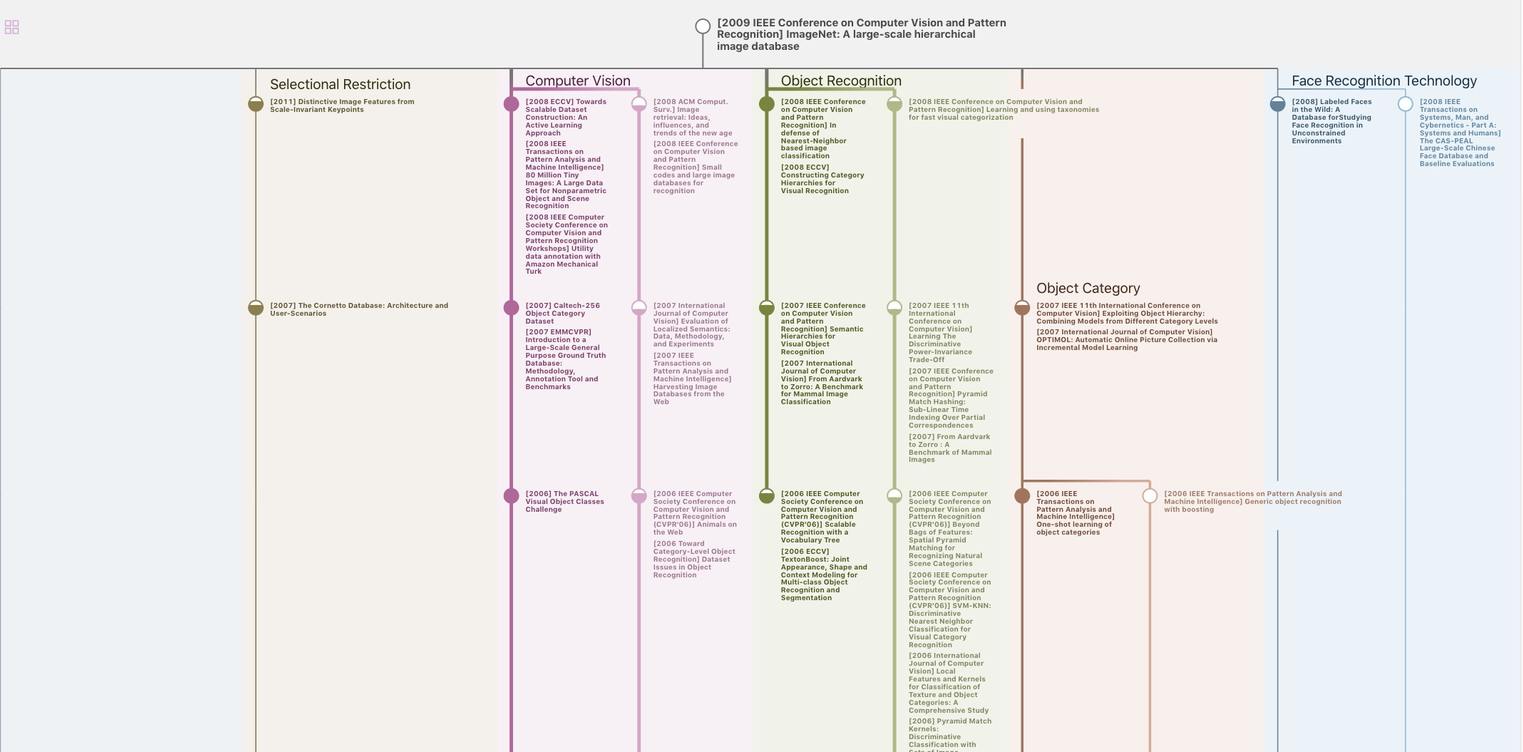
生成溯源树,研究论文发展脉络
Chat Paper
正在生成论文摘要