Online Unit Profit Knapsack with Untrusted Predictions.
Scandinavian Workshop on Algorithm Theory (SWAT)(2022)
摘要
A variant of the online knapsack problem is considered in the settings of trusted and untrusted predictions. In Unit Profit Knapsack, the items have unit profit, and it is easy to find an optimal solution offline: Pack as many of the smallest items as possible into the knapsack. For Online Unit Profit Knapsack, the competitive ratio is unbounded. In contrast, previous work on online algorithms with untrusted predictions generally studied problems where an online algorithm with a constant competitive ratio is known. The prediction, possibly obtained from a machine learning source, that our algorithm uses is the average size of those smallest items that fit in the knapsack. For the prediction error in this hard online problem, we use the ratio $r=\frac{a}{\hat{a}}$ where $a$ is the actual value for this average size and $\hat{a}$ is the prediction. The algorithm presented achieves a competitive ratio of $\frac{1}{2r}$ for $r\geq 1$ and $\frac{r}{2}$ for $r\leq 1$. Using an adversary technique, we show that this is optimal in some sense, giving a trade-off in the competitive ratio attainable for different values of $r$. Note that the result for accurate advice, $r=1$, is only $\frac{1}{2}$, but we show that no algorithm knowing the value $a$ can achieve a competitive ratio better than $\frac{e-1}{e}\approx 0.6321$ and present an algorithm with a matching upper bound. We also show that this latter algorithm attains a competitive ratio of $r\frac{e-1}{e}$ for $r \leq 1$ and $\frac{e-r}{e}$ for $1 \leq r < e$, and no algorithm can be better for both $r<1$ and $1\leq r更多
查看译文
关键词
online unit profit knapsack
AI 理解论文
溯源树
样例
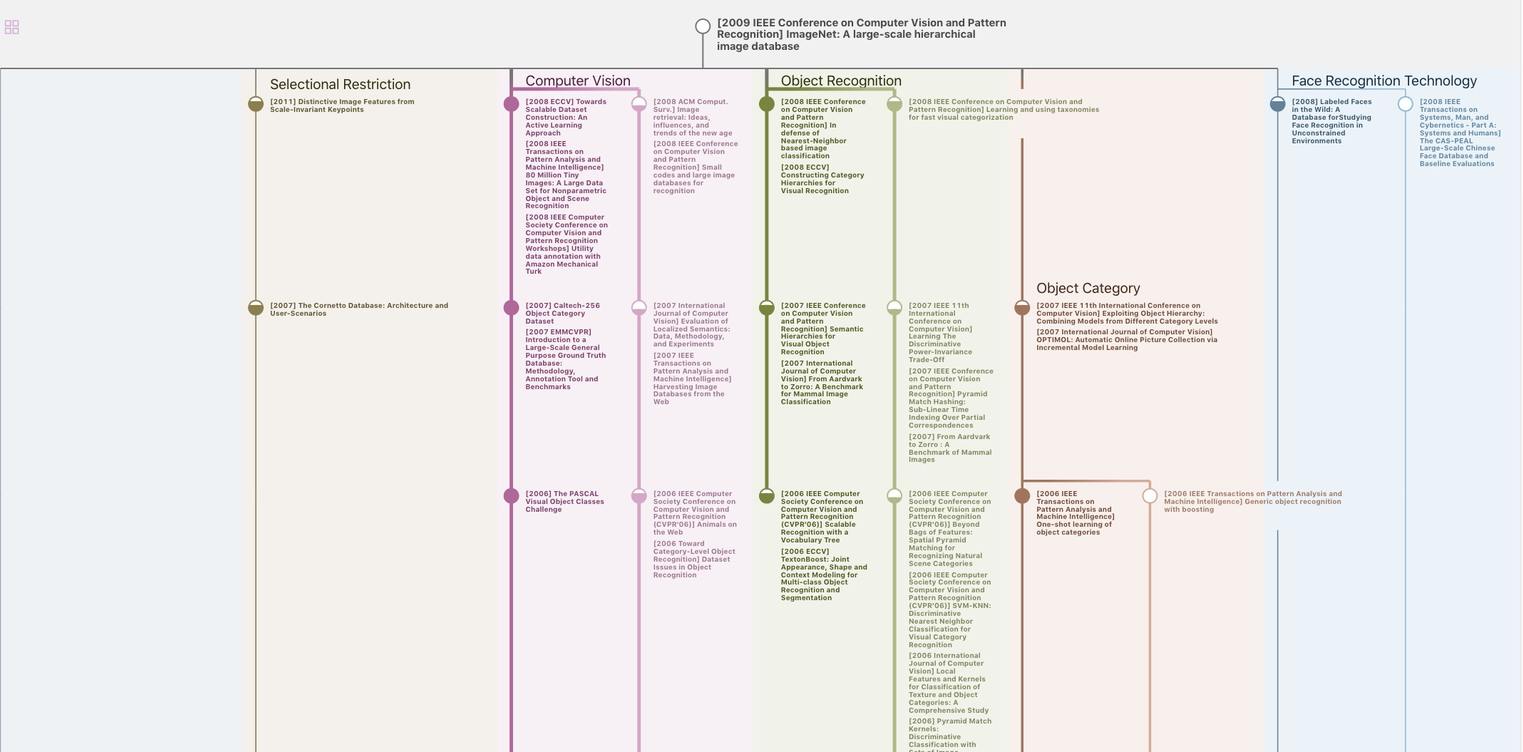
生成溯源树,研究论文发展脉络
Chat Paper
正在生成论文摘要