Physics-Informed Neural Networks for Quantum Eigenvalue Problems
IEEE International Joint Conference on Neural Network (IJCNN)(2022)
摘要
Eigenvalue problems are critical to several fields of science and engineering. We expand on the method of using unsupervised neural networks for discovering eigenfunctions and eigenvalues for differential eigenvalue problems. The obtained solutions are given in an analytical and differentiable form that identically satisfies the desired boundary conditions. The network optimization is data-free and depends solely on the predictions of the neural network. We introduce two physics-informed loss functions. The first, called ortho-loss, motivates the network to discover pair-wise orthogonal eigenfunctions. The second loss term, called norm-loss, requests the discovery of normalized eigenfunctions and is used to avoid trivial solutions. We find that embedding even or odd symmetries to the neural network architecture further improves the convergence for relevant problems. Lastly, a patience condition can be used to automatically recognize eigenfunction solutions. This proposed unsupervised learning method is used to solve the finite well, multiple finite wells, and hydrogen atom eigenvalue quantum problems.
更多查看译文
关键词
neural networks,eigenvalue,eigenfunction,differential equation
AI 理解论文
溯源树
样例
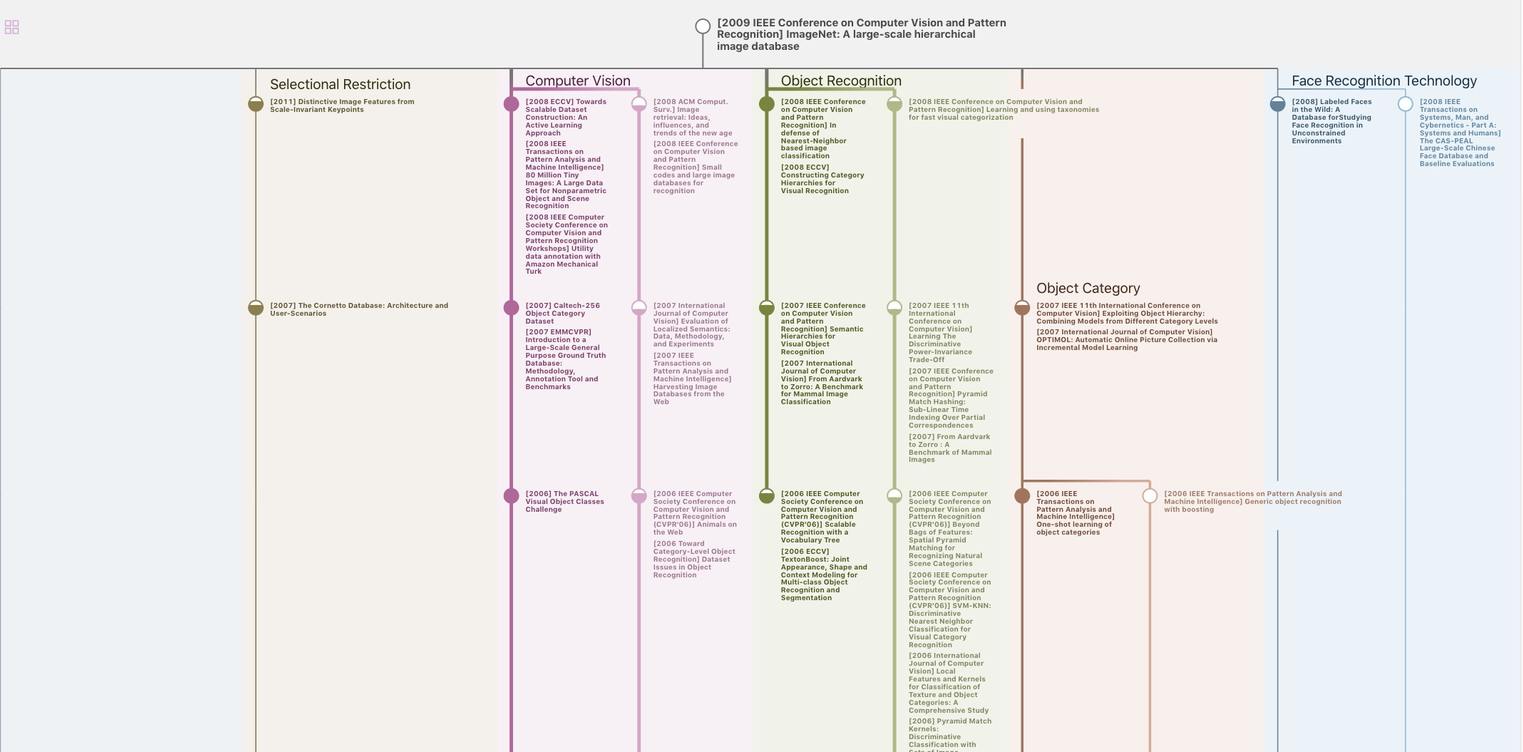
生成溯源树,研究论文发展脉络
Chat Paper
正在生成论文摘要