Learning-Aided Dynamic Access Control in MEC-Enabled Green IoT Networks: A Convolutional Reinforcement Learning Approach
IEEE Transactions on Vehicular Technology(2022)
摘要
The ongoing growth of Internet of Things (IoT) traffic increasesthe emergence of Multi-Access Edge Computing (MEC), which can serve IoT devices to process edge missions properly. The power supply for IoT devices is addressed by energy harvesting (EH) technology. However, the energy arrival model obeys the unknown energy arrival process. In addition, due to the dynamical changes of network state, it is crucial to study the access control in the MEC-based green IoT system. In this paper, we propose an MEC-based access control strategy to maximize the system utility for the green IoT system, considering the spectral efficiency and the successful access ratio from the perspective of operators and users respectively. Specifically, we model the access control problem as a Constrained Markov Decision Process (CMDP), and develop a novel centralized reward-based experience replay deep convolutional Q network algorithm (RCQN) to attain the optimal access control for EH IoT devices. Additionally, to enrich the prior knowledge of access control strategy, we design a Long Short-Term Memory (LSTM) based energy prediction module to predict the energy state of IoT devices by learning the historical energy arrival information, whose output is taken as the state information of RCQN. Simulation results demonstrate that the proposed LSTM-based RCQN access control algorithm significantly improves the system utility with a proper reward design and training mechanism.
更多查看译文
关键词
Deep reinforcement learning,Long short-term memory,energy harvesting,access control,Internet of things,Multi-access edge computing
AI 理解论文
溯源树
样例
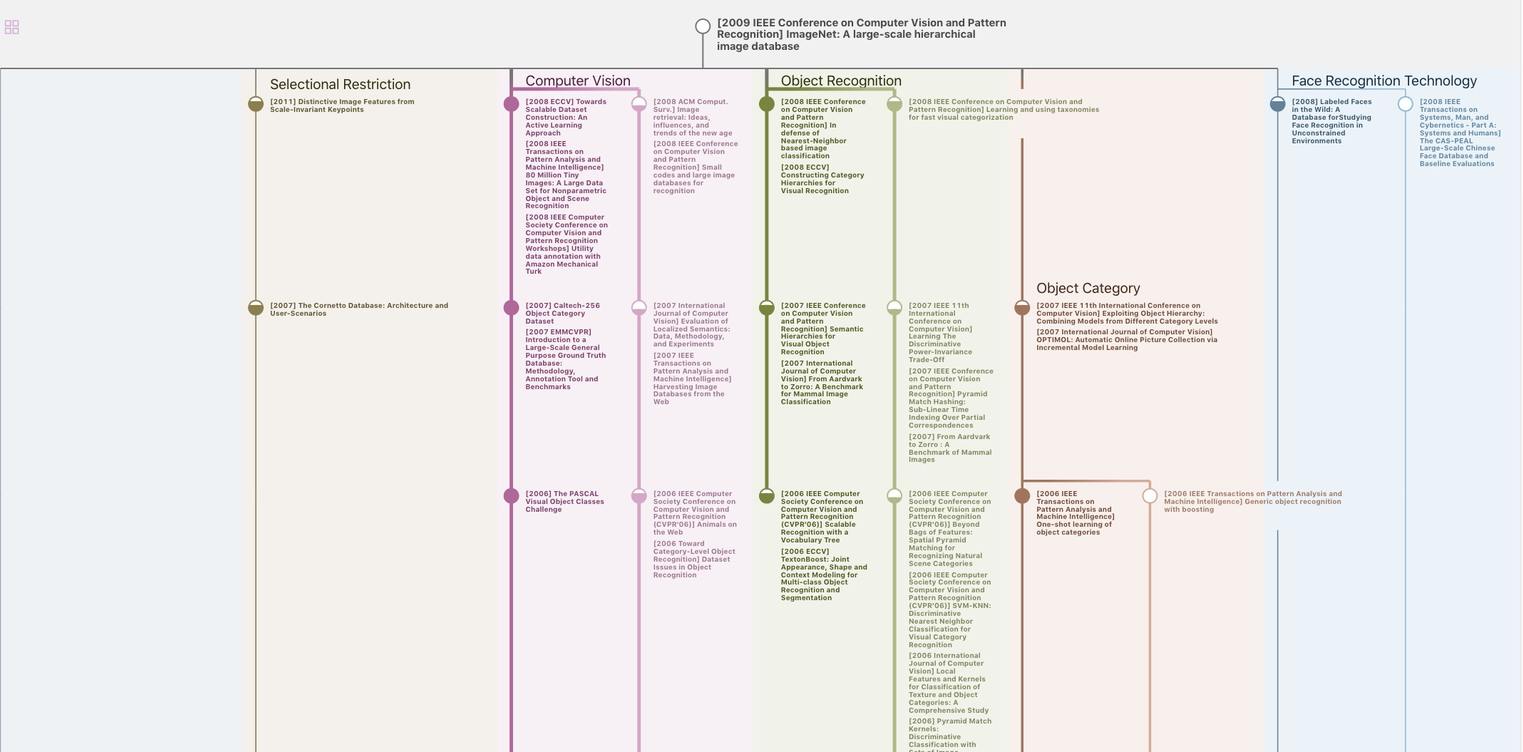
生成溯源树,研究论文发展脉络
Chat Paper
正在生成论文摘要