Adaptive Spatio-Temporal Graph Information Fusion for Remaining Useful Life Prediction
IEEE Sensors Journal(2022)
摘要
Accurate remaining useful life (RUL) prediction is of great significance for maintaining the safety and reliability of many industrial systems. In recent years, deep learning based-methods predict the RUL by automatically learning and fusing degradation features from signals and have shown great potential in improving prediction accuracy. However, these methods only focus on capturing degradation information from the sensor signals in the time domain while ignoring the characteristics of different sensors in the spatial domain. Our key motivation is that spatial characteristic is also critical in RUL prediction and cast the RUL prediction problem into a deep spatio-temporal graph fusion problem, which consists of two parts,
i.e.
, spatial structures learning and spatio-temporal information fusion. We propose a framework, namely, adaptive spatio-temporal graph neural network (ASTGNN) to solve the above problem. In the spatial structures learning part, we propose two spatial graph convolution layers,
i.e.
, ASTGNN-M and ASTGNN-A to learn the spatial structures adaptively from the time-varying signals. In the spatio-temporal information fusion part, we use dilation convolution to alleviate the over-smoothing problem when encountering long sensor signals. Moreover, based on ASTGNN, a model named adaptive spatio-temporal hypergraph neural network (ASTHGNN) is proposed for high-order spatio-temporal feature learning. The performances of ASTGNN-M, ASTGNN-A and ASTHGNN are investigated on the C-MAPSS turbofan engine dataset. Experimental results show that ASTHGNN achieves superior performance compared with the state-of-the-art methods and the proposed methods can effectively learn the graph and hypergraph structures of sensor signals.
更多查看译文
关键词
RUL prediction,spatio-temporal graph fusion,adaptive graph learning,adaptive hypergraph learning
AI 理解论文
溯源树
样例
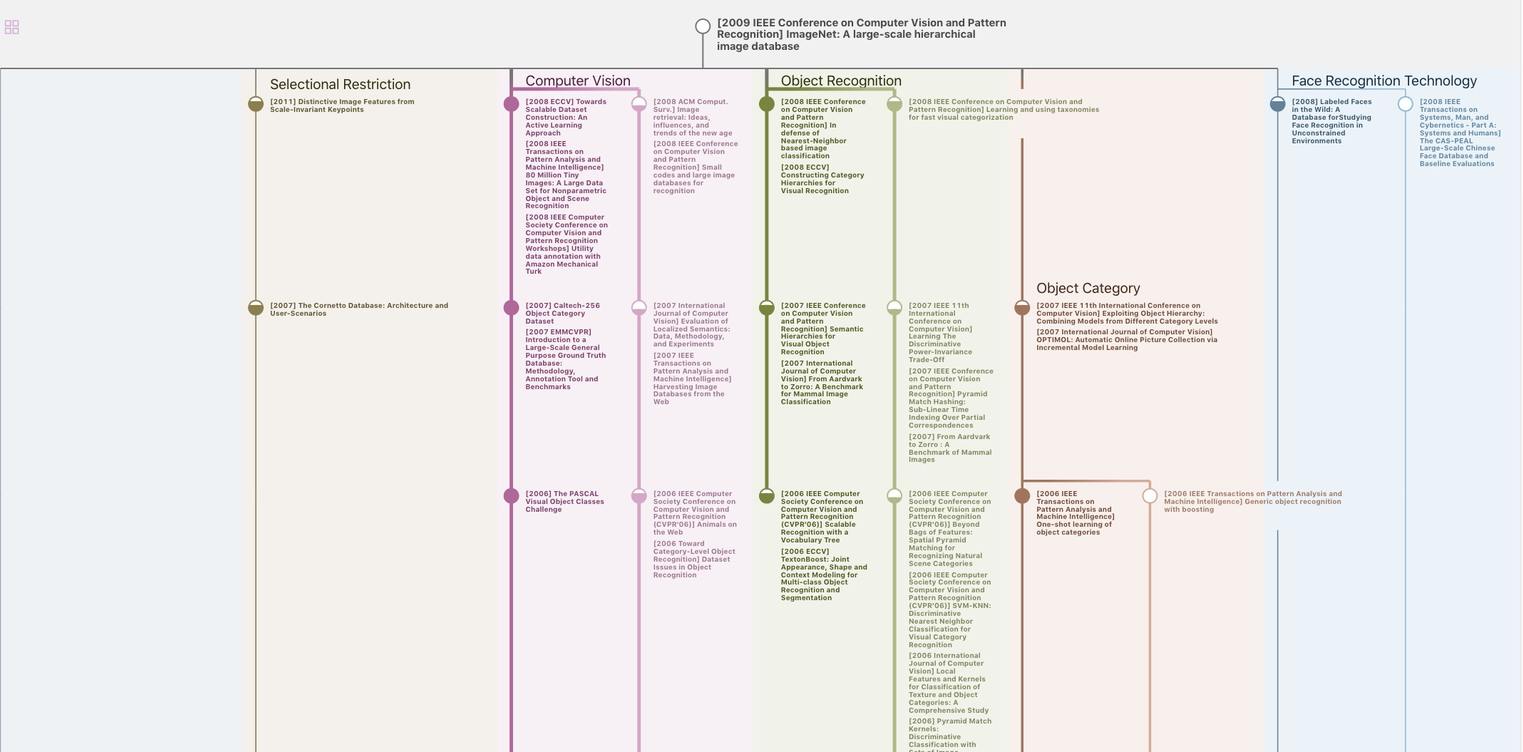
生成溯源树,研究论文发展脉络
Chat Paper
正在生成论文摘要