Unified and Fast Human Trajectory Prediction Via Conditionally Parameterized Normalizing Flow
IEEE Robotics and Automation Letters(2022)
摘要
Human trajectory prediction is crucial for service robots, autonomous driving and advanced driver assistant systems. Current top-performing methods mainly rely on intractable generative models to learn a distribution of future trajectories, and sample multiple plausible ones as prediction results. In this letter, we propose a novel perspective for trajectory prediction, called
Predicting Flow
, which models the probability distribution of future trajectories explicitly and tractably by a conditionally parameterized normalizing flow. Compared with previous methods, our prediction framework is unified and faster by enjoying three advantages: i) Both future trajectories and probability maps of future positions can be predicted with our network, bringing more comprehensive prediction results. ii) For future trajectories, exact probabilities for predicted samples can be directly calculated without the aid of extra modules. iii) Our model is adaptive and compact with the conditional parameterization mechanism, leading to significantly stronger expression capability and higher inference speed. Exhaustive experiments on widely-used benchmarks demonstrate that our approach outperforms state-of-the-art performance in both accuracy and inference speed.
更多查看译文
关键词
AI-Based methods,intelligent transportation systems,computer vision for transportation,human trajectory prediction,normalizing flows
AI 理解论文
溯源树
样例
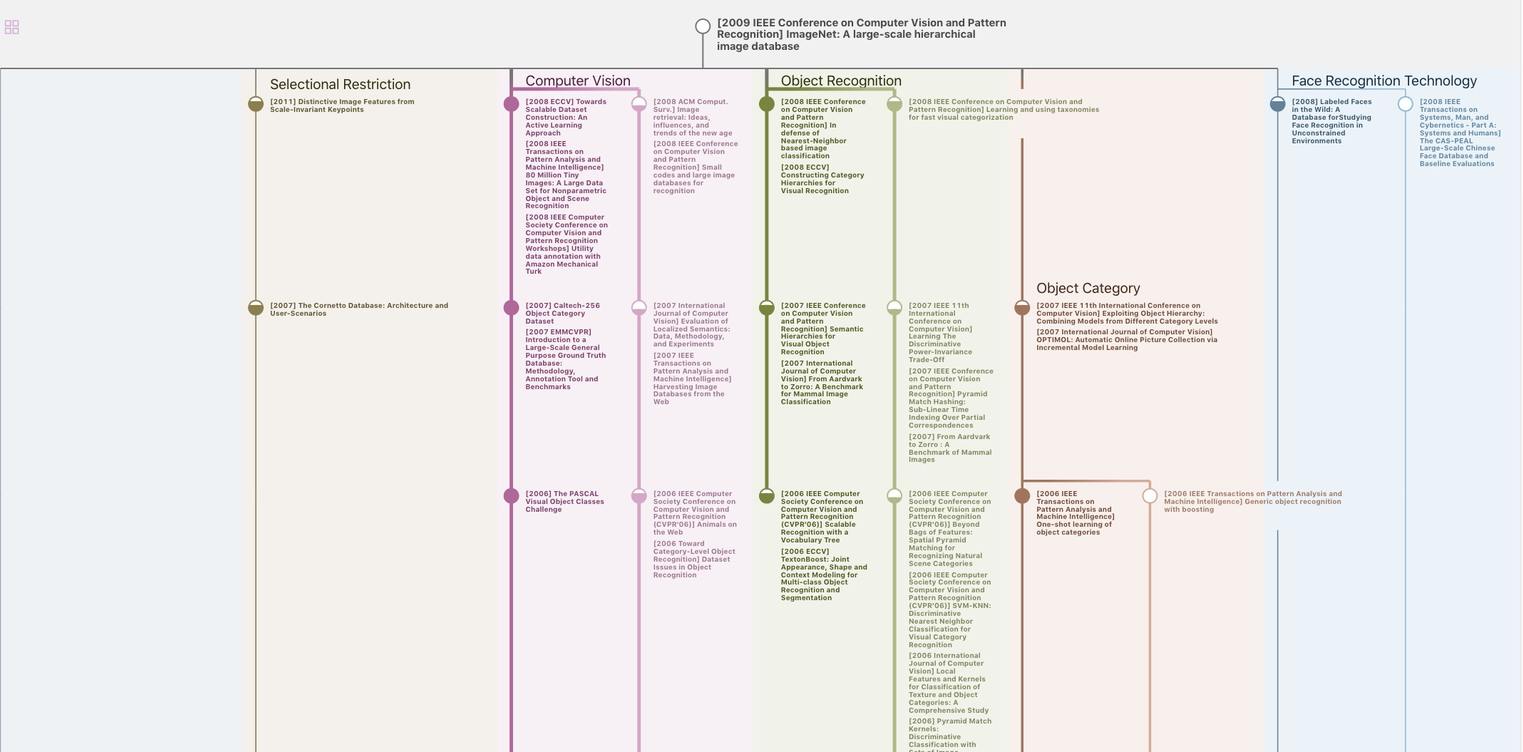
生成溯源树,研究论文发展脉络
Chat Paper
正在生成论文摘要