Experiments in Adaptive Replanning for Fast Autonomous Flight in Forests
IEEE International Conference on Robotics and Automation(2022)
摘要
Fast, autonomous flight in unstructured, cluttered environments such as forests is challenging because it requires the robot to compute new plans in realtime on a computationally-constrained platform. In this paper, we enable this capability with a search-based planning framework that adapts sampling density in realtime to find dynamically-feasible plans while remaining computationally tractable. A paramount challenge in search-based planning is that dense obstacles both necessitate large graphs (to guarantee completeness) and reduce the efficiency of graph search (as heuristics become less accurate). To address this, we develop a planning framework with two parts: one that maximizes planner completeness for a given graph size, and a second that dynamically maximizes graph size subject to computational constraints. This framework is enabled by motion planning graphs that are defined by a single parameter-dispersion-which quantifies the maximum trajectory cost to reach an arbitrary state from the graph. We show through real and simulated experiments how the dispersion can be adapted to different environments in realtime, allowing operation in environments with varying density. The simulated experiment demonstrates improved performance over a baseline search-based planning algorithm. We also demonstrate flight speeds of up to 2.5m/s in real-world cluttered pine forests.
更多查看译文
关键词
adaptive replanning,fast autonomous flight,unstructured environments,cluttered environments,computationally-constrained platform,dynamically-feasible plans,search-based planning,graph search,planner completeness,computational constraints,motion planning graphs,maximum trajectory cost,flight speeds,real-world cluttered pine forests,dynamically maximized graph size,parameter-dispersion,baseline search-based method
AI 理解论文
溯源树
样例
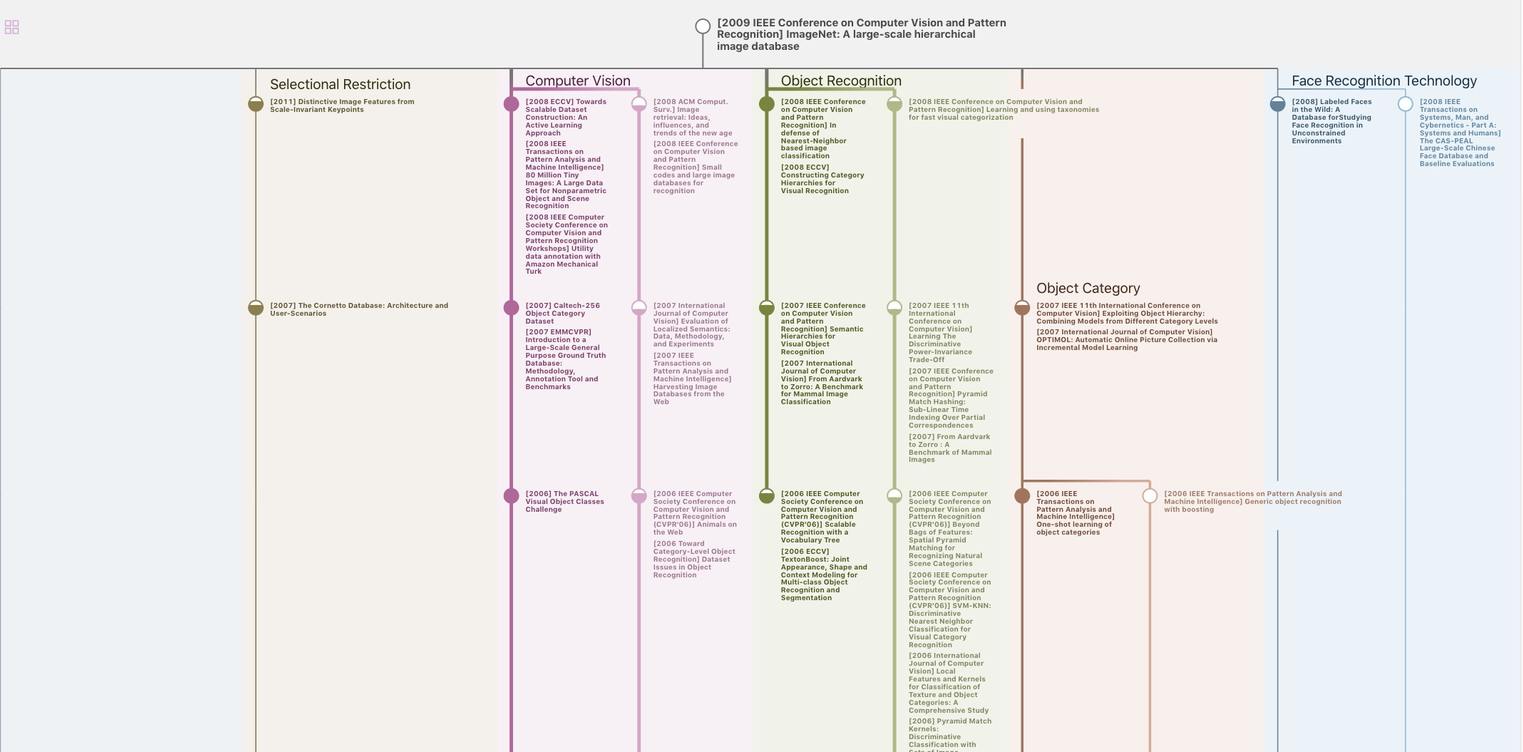
生成溯源树,研究论文发展脉络
Chat Paper
正在生成论文摘要