Uniform Approximations for Randomized Hadamard Transforms with Applications
PROCEEDINGS OF THE 54TH ANNUAL ACM SIGACT SYMPOSIUM ON THEORY OF COMPUTING (STOC '22)(2022)
摘要
Randomized Hadamard Transforms (RHTs) have emerged as a computationally efficient alternative to the use of dense unstructured random matrices across a range of domains in computer science and machine learning. For several applications such as dimensionality reduction and compressed sensing, the theoretical guarantees for methods based on RHTs are comparable to approaches using dense random matrices with i.i.d. entries. However, several such applications are in the low-dimensional regime where the number of rows sampled from the matrix is rather small. Prior arguments are not applicable to the high-dimensional regime often found in machine learning applications like kernel approximation. Given an ensemble of RHTs with Gaussian diagonals, {M-i}(i=1)(m), and any 1-Lipschitz function, f : R -> R, we prove that the average of.. over the entries of {M-i nu}(i=1)(m) converges to its expectation uniformly over ||nu|| <= 1 at a rate comparable to that obtained from using truly Gaussian matrices. We use our inequality to then derive improved guarantees for two applications in the high-dimensional regime: 1) kernel approximation and 2) distance estimation. For kernel approximation, we prove the first uniform approximation guarantees for random features constructed through RHTs lending theoretical justification to their empirical success while for distance estimation, our convergence result implies data structures with improved runtime guarantees over previous work by the authors. We believe our general inequality is likely to find use in other applications.
更多查看译文
关键词
adaptive statistics, random matrix theory, hadamard transforms, kernel approximation, distance estimation, pseudo-randomness
AI 理解论文
溯源树
样例
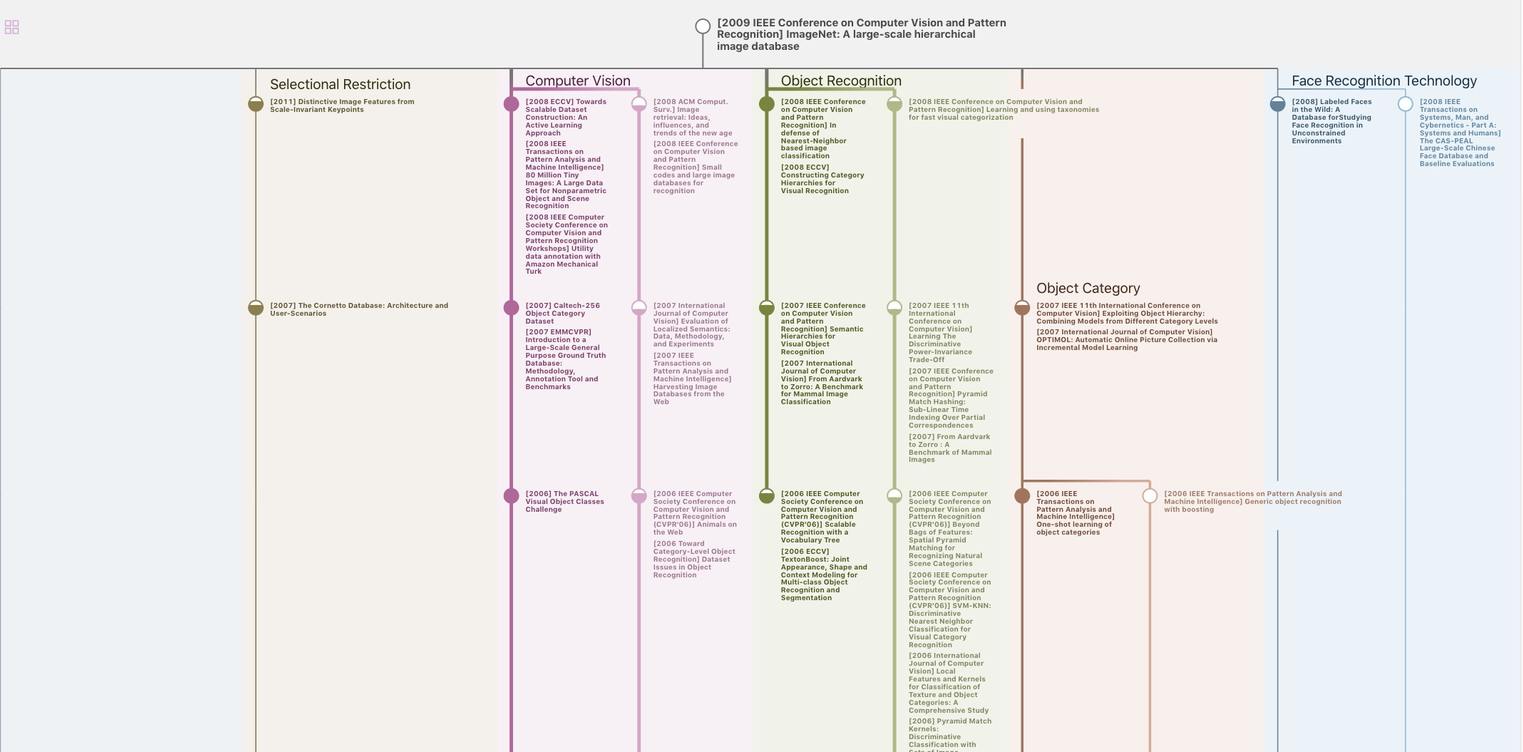
生成溯源树,研究论文发展脉络
Chat Paper
正在生成论文摘要