On Data Augmentation for Models Involving Reciprocal Gamma Functions
arxiv(2023)
摘要
In this paper, we introduce a new and efficient data augmentation approach to the posterior inference of the models with shape parameters when the reciprocal gamma function appears in full conditional densities. Our approach is to approximate full conditional densities of shape parameters by using Gauss's multiplication formula and Stirling's formula for the gamma function, where the approximation error can be made arbitrarily small. We use the techniques to construct efficient Gibbs and Metropolis-Hastings algorithms for a variety of models that involve the gamma distribution, Student's $t$-distribution, the Dirichlet distribution, the negative binomial distribution, and the Wishart distribution. The proposed sampling method is numerically demonstrated through simulation studies.
更多查看译文
关键词
Gauss's multiplication formula,Markov chain Monte Carlo,Reciprocal gamma function,Stirling's formula
AI 理解论文
溯源树
样例
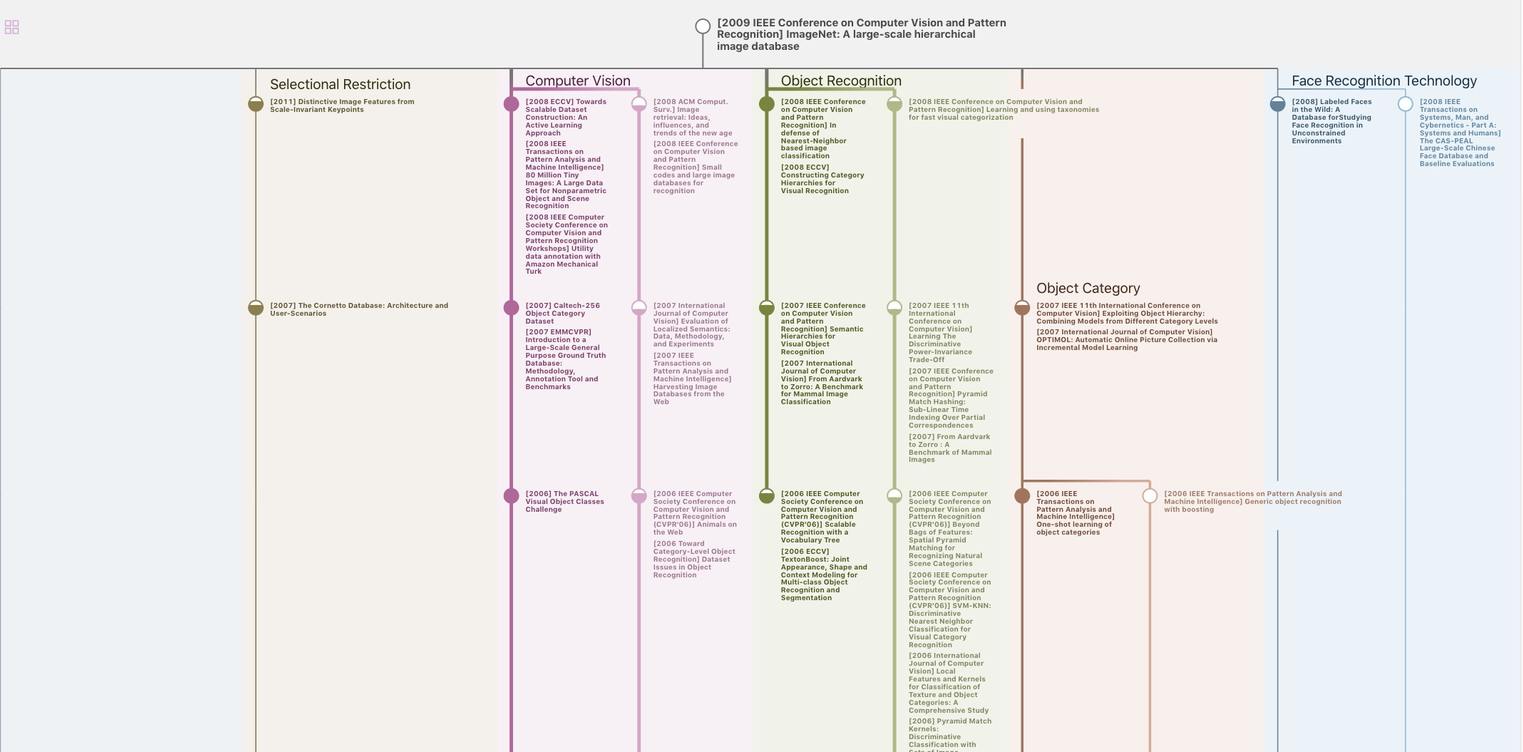
生成溯源树,研究论文发展脉络
Chat Paper
正在生成论文摘要