Identification in Tree-shaped Linear Structural Causal Models
INTERNATIONAL CONFERENCE ON ARTIFICIAL INTELLIGENCE AND STATISTICS, VOL 151(2022)
摘要
Linear structural equation models represent direct causal effects as directed edges and confounding factors as bidirected edges. An open problem is to identify the causal parameters from correlations between the nodes. We investigate models, whose directed component forms a tree, and show that there, besides classical instrumental variables, missing cycles of bidirected edges can be used to identify the model. They can yield systems of quadratic equations that we explicitly solve to obtain one or two solutions for the causal parameters of adjacent directed edges. We show how multiple missing cycles can be combined to obtain a unique solution. This results in an algorithm that can identify instances that previously required approaches based on Grobner bases, which have doubly-exponential time complexity in the number of structural parameters.
更多查看译文
关键词
models,structural,tree-shaped
AI 理解论文
溯源树
样例
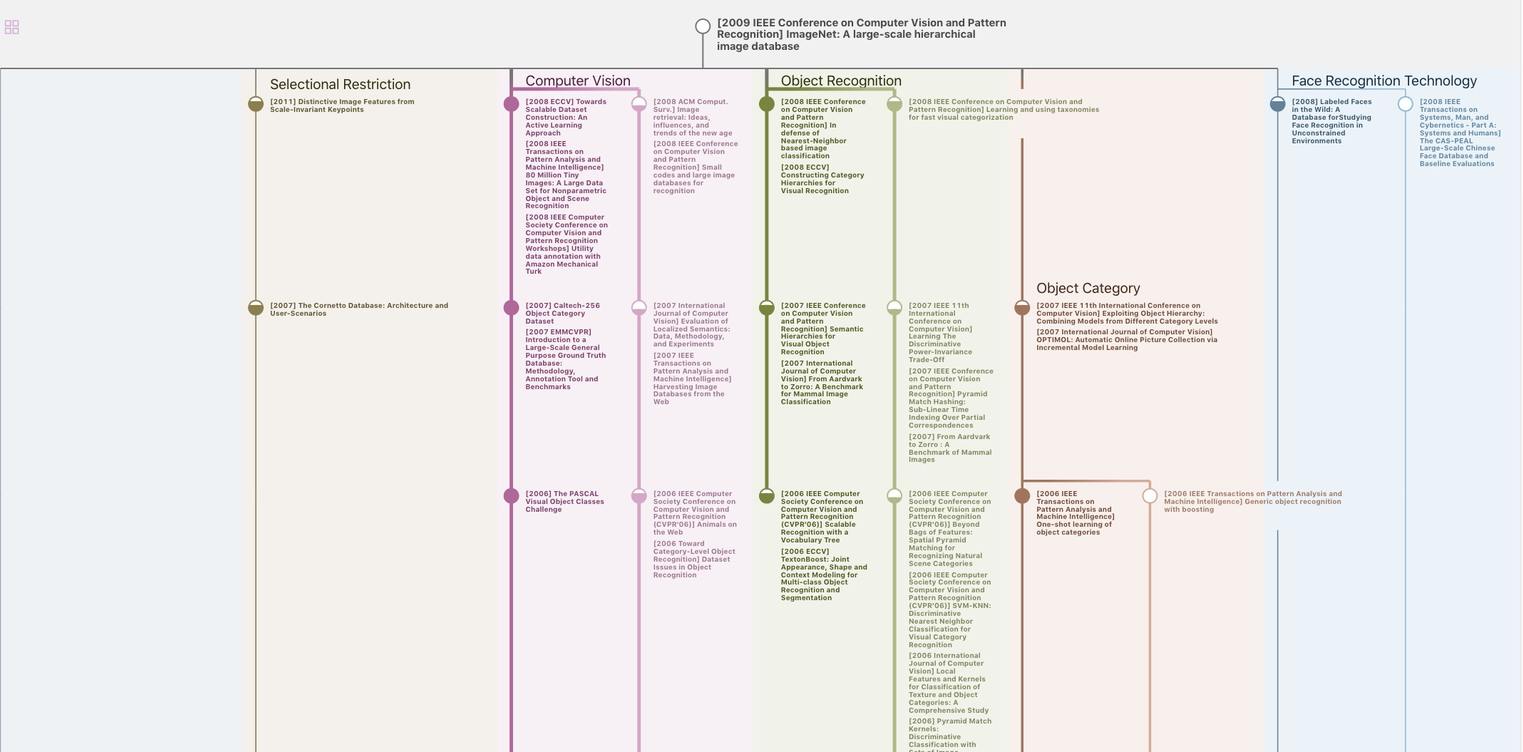
生成溯源树,研究论文发展脉络
Chat Paper
正在生成论文摘要