Cnc-nos
Periodicals(2018)
摘要
AbstractA novel class noise cleaner able not only to remove noisy instances but to successfully relabel them.It introduces an improvement of the noise score measure already proposed in a recent filter, making it sensitive to the proximity of the neighbors.A wide range of noise levels are used to compare the proposal in order to validate its behavior in different scenarios.Experimental results outperform the state-of-the-art approaches in noise filtering and other noise correctors for class noise, the most common approach.A thorough analysis on the effect of the compared approaches in terms of successfully and wrongly treated instances is provided and related to its performance. Obtaining data in the real world is subject to imperfections and the appearance of noise is a common consequence of such flaws. In classification, class noise will deteriorate the performance of a classifier, as it may severely mislead the model building. Among the strategies emerged to deal with class noise, the most popular is that of filtering. However, instance filtering can be harmful as it may eliminate more examples than necessary or produce loss of information. An ideal option would be relabeling the noisy instances, avoiding losing data, but instance correcting is harder to achieve and may lead to wrong information being introduced in the dataset. For this reason, we advance a new proposal based on an ensemble of noise filters with the goal not only of accurately filtering the mislabeled instances, but also correcting them when possible. A noise score is also applied to support the filtering and relabeling process. The proposal, named CNC-NOS (Class Noise Cleaner with Noise Scoring), is compared against state-of-the-art noise filters and correctors, showing that it is able to deliver a quality training instance set that overcomes the limitations of such techniques, both in terms of classification accuracy and properly treated instances.
更多查看译文
关键词
Class noise,Classification,Data preprocessing,Data relabeling,Data reparation,Noise filtering,Noisy data
AI 理解论文
溯源树
样例
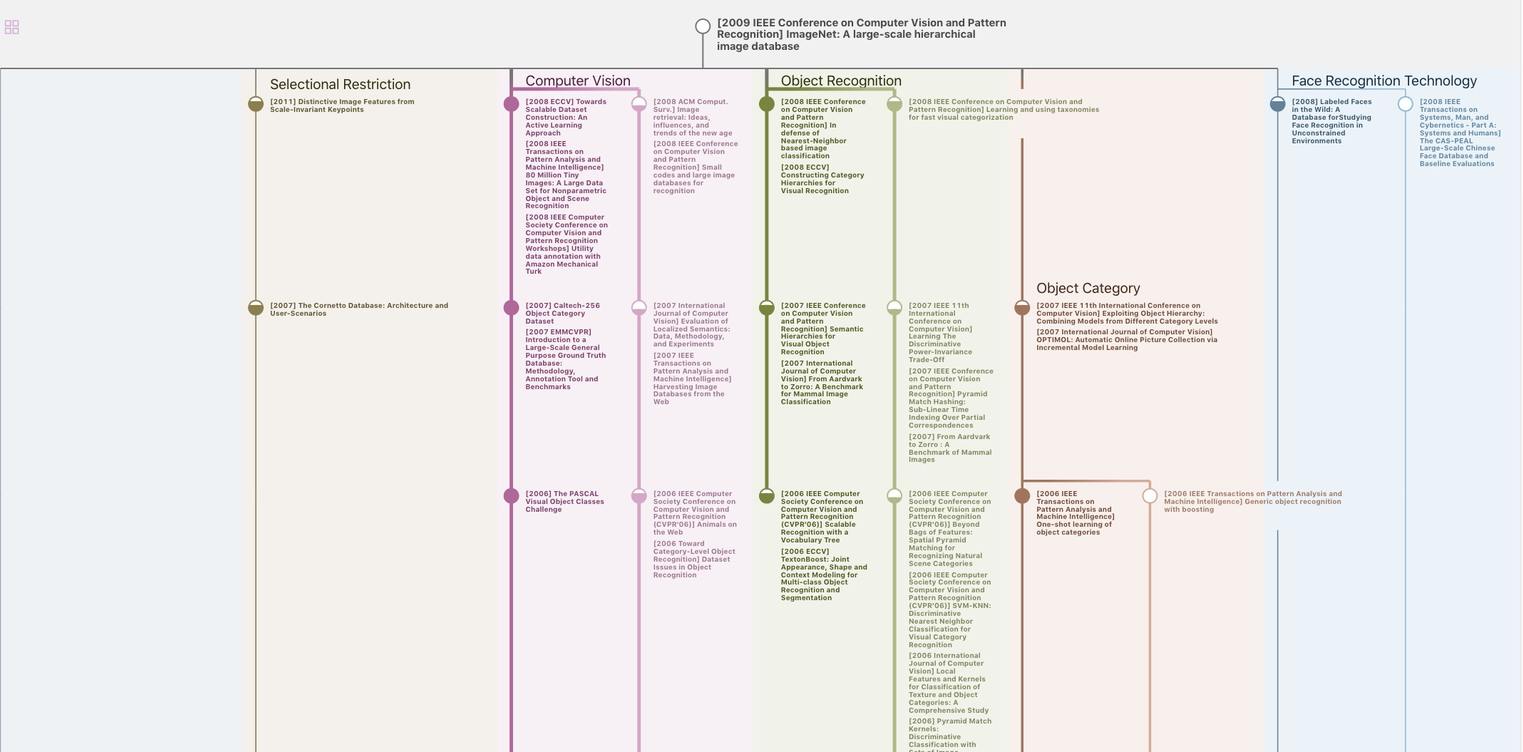
生成溯源树,研究论文发展脉络
Chat Paper
正在生成论文摘要