Tri-training for Dependency Parsing Domain Adaptation
ACM Transactions on Asian and Low-Resource Language Information Processing(2022)
摘要
AbstractIn recent years, the research on dependency parsing focuses on improving the accuracy of the domain-specific (in-domain) test datasets and has made remarkable progress. However, there are innumerable scenarios in the real world that are not covered by the dataset, namely, the out-of-domain dataset. As a result, parsers that perform well on the in-domain data usually suffer from significant performance degradation on the out-of-domain data. Therefore, to adapt the existing in-domain parsers with high performance to a new domain scenario, cross-domain transfer learning methods are essential to solve the domain problem in parsing. This paper examines two scenarios for cross-domain transfer learning: semi-supervised and unsupervised cross-domain transfer learning. Specifically, we adopt a pre-trained language model BERT for training on the source domain (in-domain) data at the subword level and introduce self-training methods varied from tri-training for these two scenarios. The evaluation results on the NLPCC-2019 shared task and universal dependency parsing task indicate the effectiveness of the adopted approaches on cross-domain transfer learning and show the potential of self-learning to cross-lingual transfer learning.
更多查看译文
关键词
Tri-training, dependency parsing, domain adaptation, transfer learning
AI 理解论文
溯源树
样例
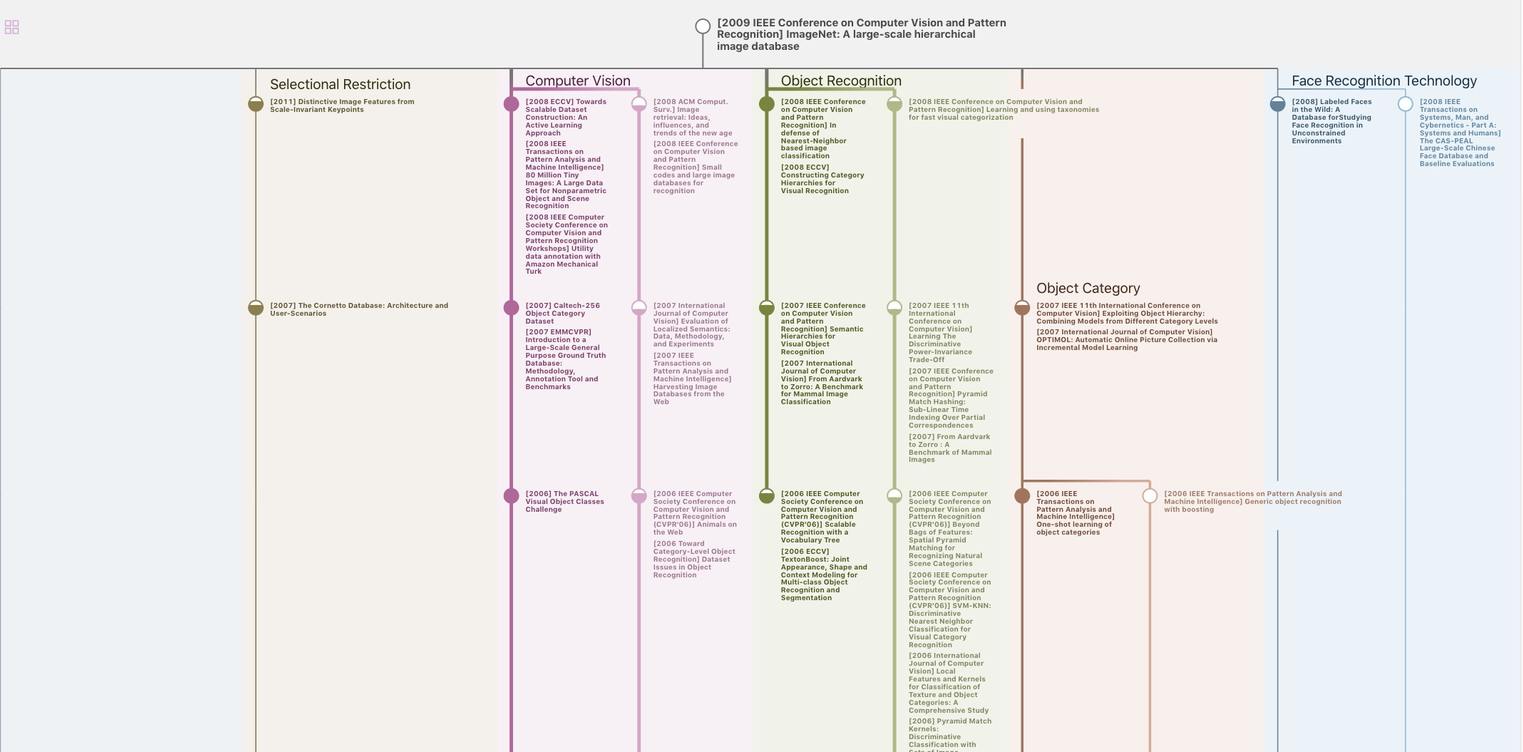
生成溯源树,研究论文发展脉络
Chat Paper
正在生成论文摘要