A Wasserstein distance-based spectral clustering method for transaction data analysis
arxiv(2023)
摘要
With the rapid development of online payment platforms, it is now possible to record massive transaction data. Clustering on transaction data significantly contributes to analyzing merchants' behavior patterns. This enables payment platforms to provide differentiated services or implement risk management strategies. However, traditional methods exploit transactions by generating low-dimensional features, leading to inevitable information loss. In this study, we use the empirical cumulative distribution of transactions to characterize merchants. We adopt Wasserstein distance to measure the dissimilarity between any two merchants and propose the Wasserstein-distance-based spectral clustering (WSC) approach. Based on the similarities between merchants' transaction distributions, a graph of merchants is generated. Thus, we treat the clustering of merchants as a graph-cut problem and solve it under the framework of spectral clustering. To ensure feasibility of the proposed method on large-scale datasets with limited computational resources, we propose a subsampling method for WSC (SubWSC). The associated theoretical properties are investigated to verify the efficiency of the proposed approach. The simulations and empirical study demonstrate that the proposed method outperforms feature-based methods in finding behavior patterns of merchants.
更多查看译文
关键词
spectral clustering method,transaction
AI 理解论文
溯源树
样例
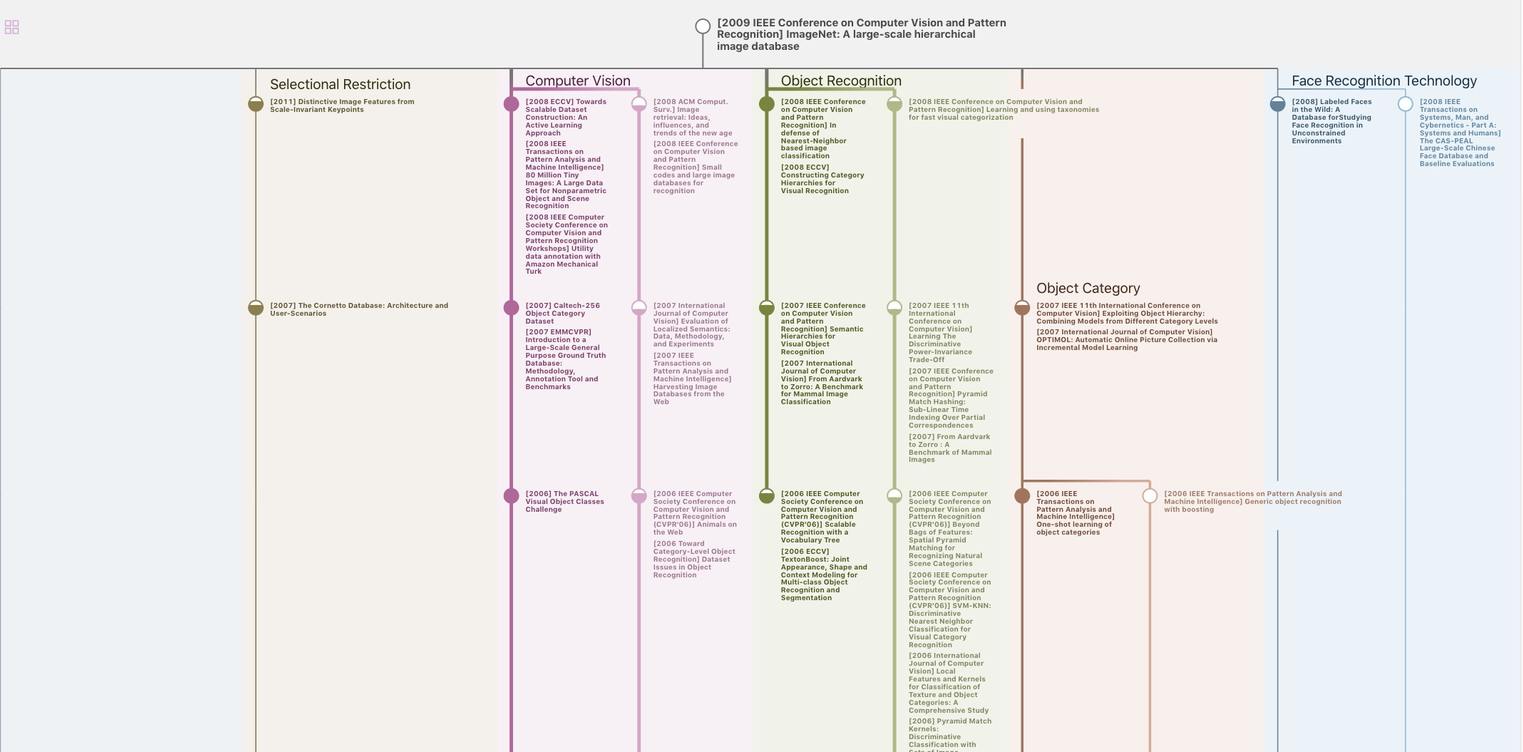
生成溯源树,研究论文发展脉络
Chat Paper
正在生成论文摘要