Divide and Conquer: Text Semantic Matching with Disentangled Keywords and Intents
FINDINGS OF THE ASSOCIATION FOR COMPUTATIONAL LINGUISTICS (ACL 2022)(2022)
摘要
Text semantic matching is a fundamental task that has been widely used in various scenarios, such as community question answering, information retrieval, and recommendation. Most state-of-the-art matching models, e.g., BERT, directly perform text comparison by processing each word uniformly. However, a query sentence generally comprises content that calls for different levels of matching granularity. Specifically, keywords represent factual information such as action, entity, and event that should be strictly matched, while intents convey abstract concepts and ideas that can be paraphrased into various expressions. In this work, we propose a simple yet effective training strategy for text semantic matching in a divide-and-conquer manner by disentangling keywords from intents. Our approach can be easily combined with pretrained language models (PLM) without influencing their inference efficiency, achieving stable performance improvements against a wide range of PLMs on three benchmarks.
更多查看译文
关键词
text semantic matching,disentangled keywords
AI 理解论文
溯源树
样例
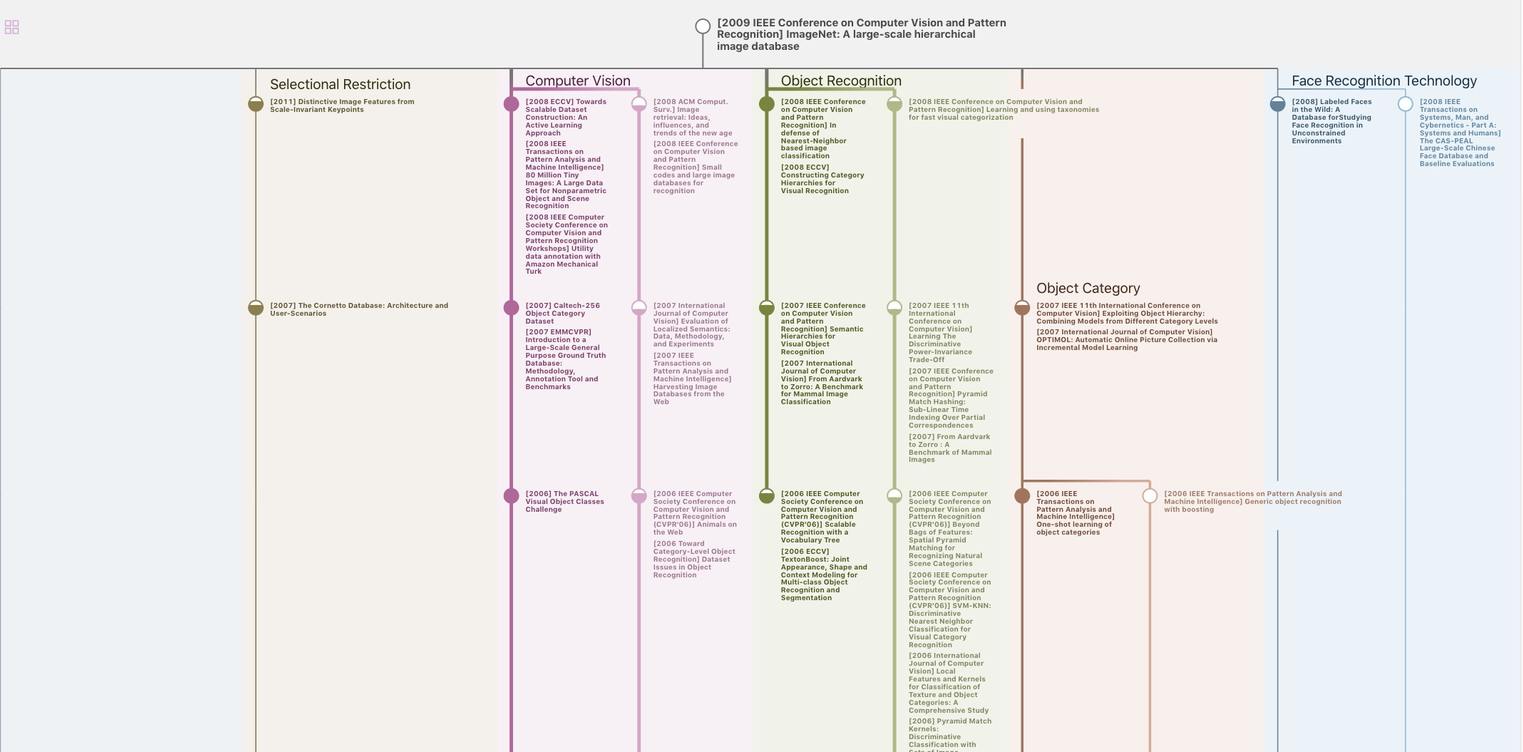
生成溯源树,研究论文发展脉络
Chat Paper
正在生成论文摘要