Machine learning using longitudinal prescription and medical claims for the detection of non-alcoholic steatohepatitis (NASH)
BMJ HEALTH & CARE INFORMATICS(2022)
摘要
Objectives To develop and evaluate machine learning models to detect patients with suspected undiagnosed non-alcoholic steatohepatitis (NASH) for diagnostic screening and clinical management. Methods In this retrospective observational non-interventional study using administrative medical claims data from 1 463 089 patients, gradient-boosted decision trees were trained to detect patients with likely NASH from an at-risk patient population with a history of obesity, type 2 diabetes mellitus, metabolic disorder or non-alcoholic fatty liver (NAFL). Models were trained to detect likely NASH in all at-risk patients or in the subset without a prior NAFL diagnosis (at-risk non-NAFL patients). Models were trained and validated using retrospective medical claims data and assessed using area under precision recall curves and receiver operating characteristic curves (AUPRCs and AUROCs). Results The 6-month incidences of NASH in claims data were 1 per 1437 at-risk patients and 1 per 2127 at-risk non-NAFL patients . The model trained to detect NASH in all at-risk patients had an AUPRC of 0.0107 (95% CI 0.0104 to 0.0110) and an AUROC of 0.84. At 10% recall, model precision was 4.3%, which is 60x above NASH incidence. The model trained to detect NASH in the non-NAFL cohort had an AUPRC of 0.0030 (95% CI 0.0029 to 0.0031) and an AUROC of 0.78. At 10% recall, model precision was 1%, which is 20x above NASH incidence. Conclusion The low incidence of NASH in medical claims data corroborates the pattern of NASH underdiagnosis in clinical practice. Claims-based machine learning could facilitate the detection of patients with probable NASH for diagnostic testing and disease management.
更多查看译文
关键词
machine learning, artificial intelligence, BMJ Health Informatics, data science, medical records
AI 理解论文
溯源树
样例
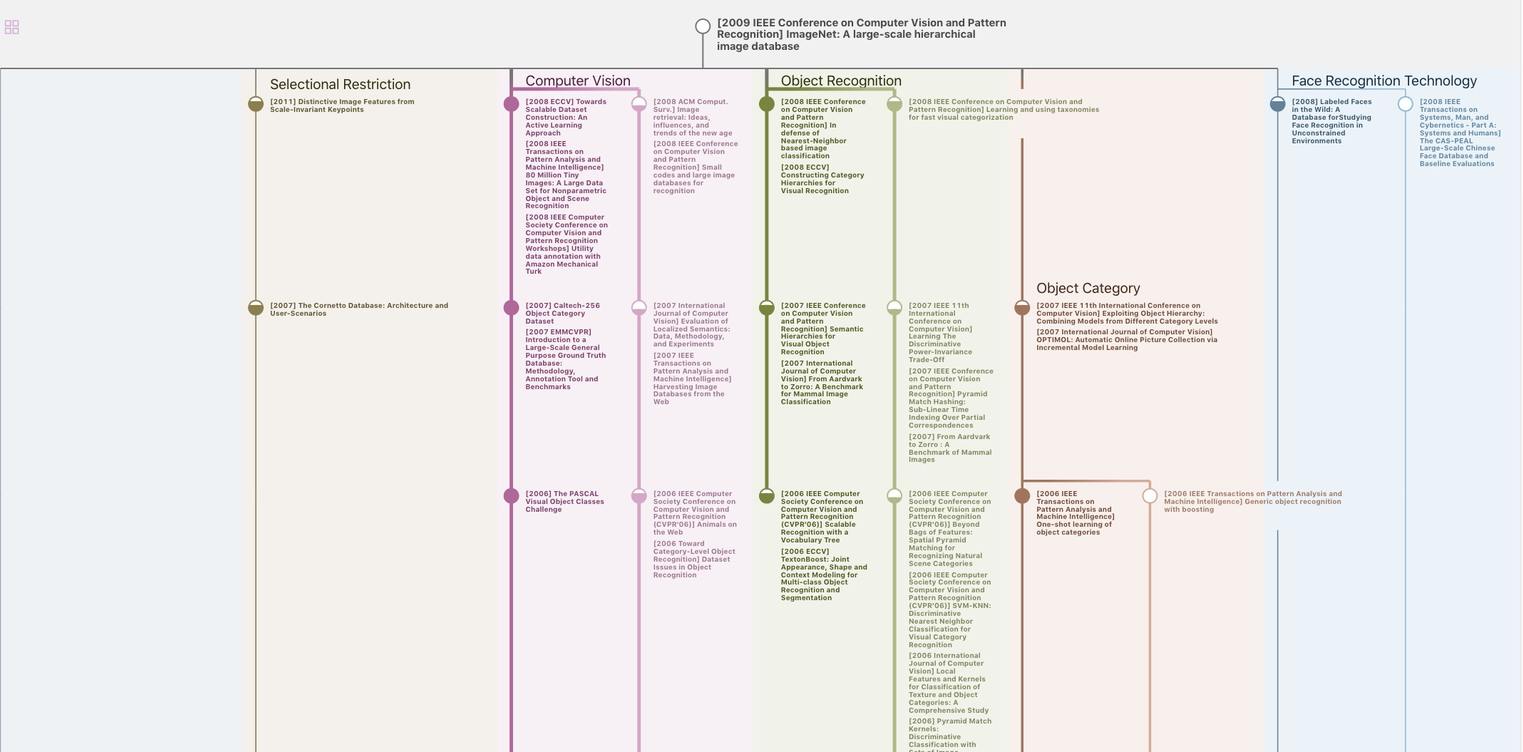
生成溯源树,研究论文发展脉络
Chat Paper
正在生成论文摘要