Multi-Modal Lidar Dataset for Benchmarking General-Purpose Localization and Mapping Algorithms.
IEEE/RJS International Conference on Intelligent RObots and Systems (IROS)(2022)
摘要
Lidar technology has evolved significantly over the last decade, with higher resolution, better accuracy, and lower cost devices available today. In addition, new scanning modalities and novel sensor technologies have emerged in recent years. Public datasets have enabled benchmarking of algorithms and have set standards for the cutting edge technology. However, existing datasets are not representative of the technological landscape, with only a reduced number of lidars available. This inherently limits the development and comparison of general-purpose algorithms in the evolving landscape. This paper presents a novel multi-modal lidar dataset with sensors showcasing different scanning modalities (spinning and solid-state), sensing technologies, and lidar cameras. The focus of the dataset is on low-drift odometry, with ground truth data available in both indoors and outdoors environment with sub-millimeter accuracy from a motion capture (MOCAP) system. For comparison in longer distances, we also include data recorded in larger spaces indoors and outdoors. The dataset contains point cloud data from spinning lidars and solid-state lidars. Also, it provides range images from high resolution spinning lidars, RGB and depth images from a lidar camera, and inertial data from built-in IMUs. This is, to the best of our knowledge, the lidar dataset with the most variety of sensors and environments where ground truth data is available. This dataset can be widely used in multiple research areas, such as 3D LiDAR simultaneous localization and mapping (SLAM), performance comparison between multi-modal lidars, appearance recognition and loop closure detection. The datasets are available at: https://github.com/TIERS/tiers-lidars-dataset.
更多查看译文
关键词
Multi-robot systems, Autonomous driving, dataset, computer vision, solid state LiDAR, SLAM
AI 理解论文
溯源树
样例
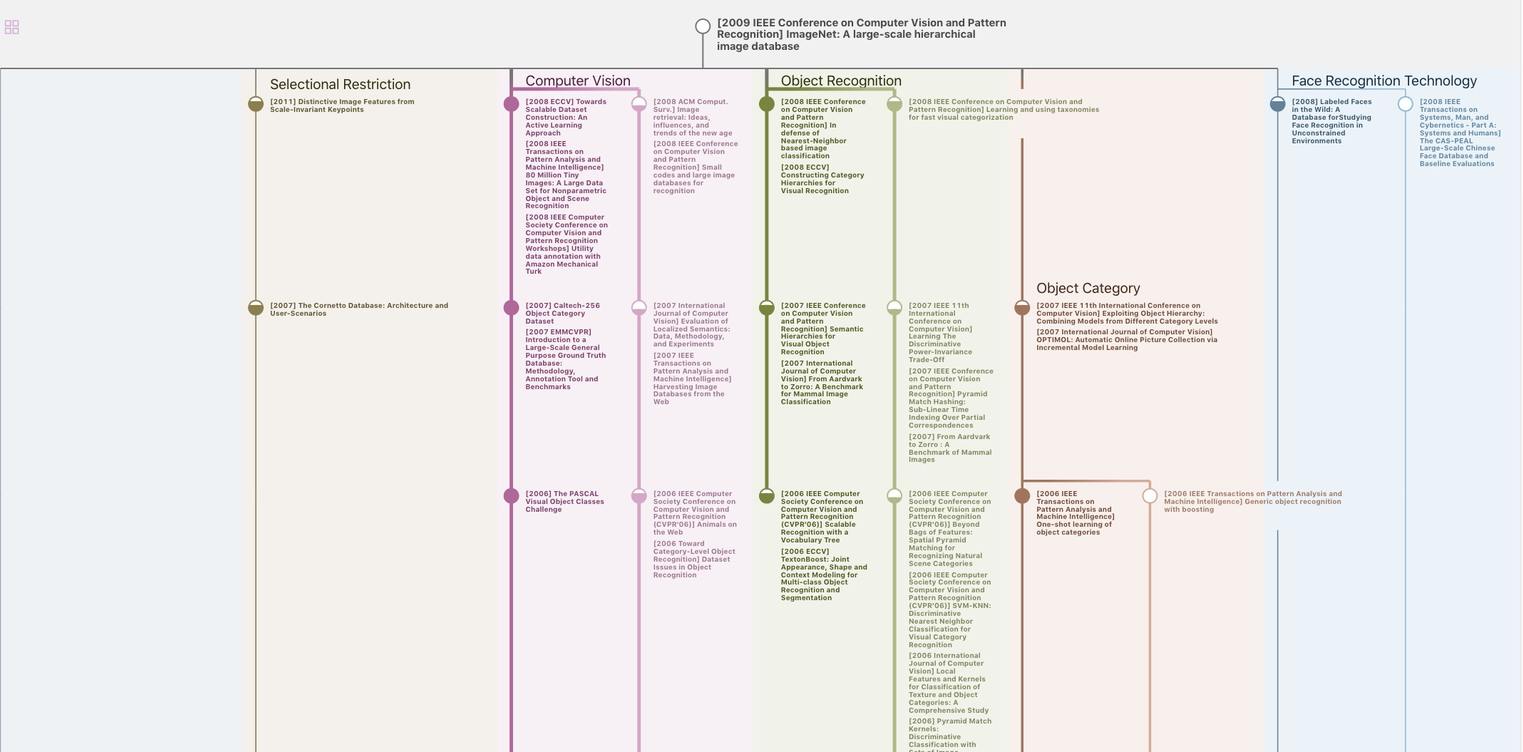
生成溯源树,研究论文发展脉络
Chat Paper
正在生成论文摘要