A new feature selection method based on feature distinguishing ability and network influence
JOURNAL OF BIOMEDICAL INFORMATICS(2022)
摘要
The occurrence and development of diseases are related to the dysfunction of biomolecules (genes, metabolites, etc.) and the changes of molecule interactions. Identifying the key molecules related to the physiological and pathological changes of organisms from omics data is of great significance for disease diagnosis, early warning and drug-target prediction, etc. A novel feature selection algorithm based on the feature individual distinguishing ability and feature influence in the biological network (FS-DANI) is proposed for defining important biomolecules (features) to discriminate different disease conditions. The feature individual distinguishing ability is evaluated based on the overlapping area of the feature effective ranges in different classes. FS-DANI measures the feature network influence based on the module importance in the correlation network and the feature centrality in the modules. The feature comprehensive weight is obtained by combining the feature individual distinguishing ability and feature influence in the network. Then crucial feature subset is determined by the sequential forward search (SFS) on the feature list sorted according to the comprehensive weights of features. FSDANI is compared with the six efficient feature selection methods on ten public omics datasets. The ablation experiment is also conducted. Experimental results show that FS-DANI is better than the compared algorithms in accuracy, sensitivity and specificity on the whole. On analyzing the gastric cancer miRNA expression data, FSDANI identified two miRNAs (hsa-miR-18a* and hsa-miR-381), whose AUCs for distinguishing gastric cancer samples and normal samples are 0.959 and 0.879 in the discovery set and an independent validation set, respectively. Hence, evaluating biomolecules from the molecular level and network level is helpful for identifying the potential disease biomarkers of high performance.
更多查看译文
关键词
Feature Selection, Omics Data, Feature Individual Distinguishing Ability, Feature Network Influence
AI 理解论文
溯源树
样例
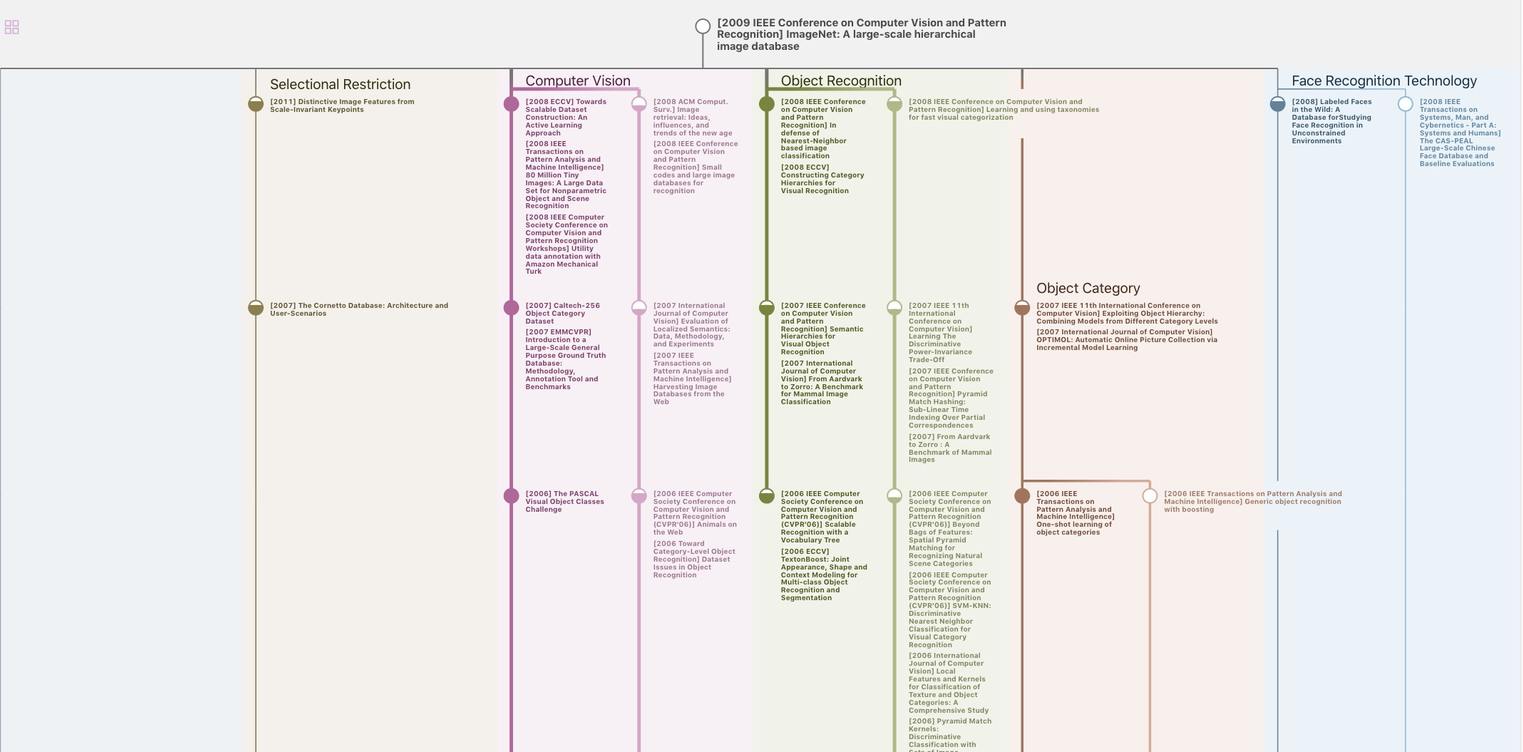
生成溯源树,研究论文发展脉络
Chat Paper
正在生成论文摘要