Convolutional Neural Networks for Raman Spectral Analysis of Chemical Mixtures
2021 5th SLAAI International Conference on Artificial Intelligence (SLAAI-ICAI)(2021)
摘要
In the spectroscopy domain, one-dimensional Convolutional Neural Networks (1D CNN) assist researchers in recognizing one pure chemical compound and distinguishing it from unknown substances. The novelty of this approach is that a trained CNN operates automatically with almost no pre-or post-processing of data. However, the application of 1-D CNNs has typically been restricted to a binary classification of pure chemical substances. This study highlights a new approach in spectral recognition and quantification of components in chemical mixtures. Two 1-D CNN models, RaMixNet I and II, have been developed for this purpose as two multi-label classifiers. Depending on data availability, there is no limit to the number of compounds in an unknown mixture to recognize by RaMixNet models. We trained RaMixNet models using generated Raman spectra utilizing a novel data augmentation technique that adds random noise and different baselines to each spectrum as well as random wavenumber shifts for Raman peaks. The experimental results over hundreds of generated synthetic test mixtures revealed that the classification accuracy of RaMixNet I and II is 100%; at the same time, the RaMixNet II model could reach an average means square error rate of 0.06 and R2 score of 0.76 for the quantification of each component. In a comparison study, RaMixNet models could distinguish components of six actual chemical mixtures better than well-established distance-based techniques in the literature.
更多查看译文
关键词
Deep learning in Chemometrics,Handheld Raman spectrum analyzer,Multi-label classification,1D Convolutional Neural Networks,and Raman mixture analysis.
AI 理解论文
溯源树
样例
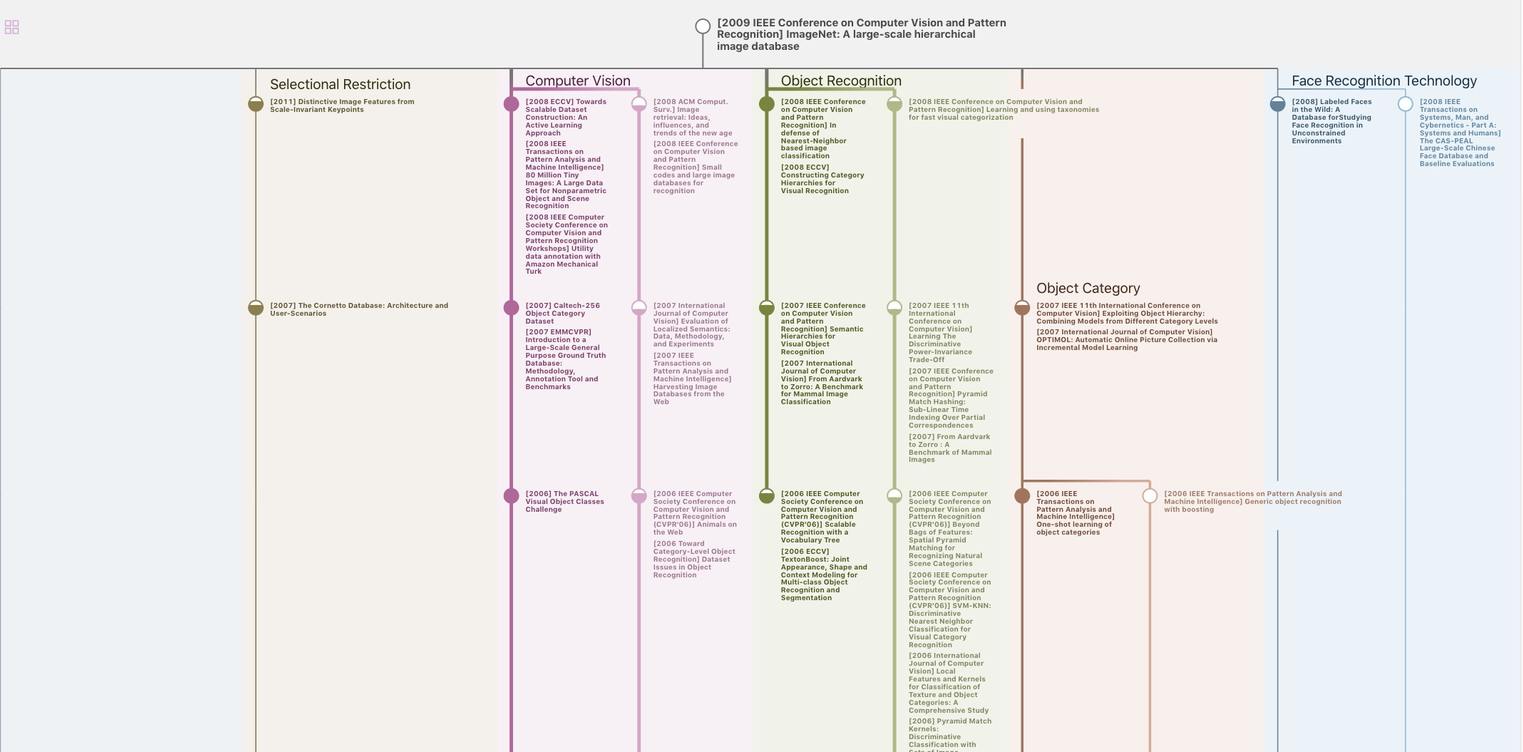
生成溯源树,研究论文发展脉络
Chat Paper
正在生成论文摘要