Towards Efficient Data-Centric Robust Machine Learning with Noise-based Augmentation
arxiv(2022)
摘要
The data-centric machine learning aims to find effective ways to build appropriate datasets which can improve the performance of AI models. In this paper, we mainly focus on designing an efficient data-centric scheme to improve robustness for models towards unforeseen malicious inputs in the black-box test settings. Specifically, we introduce a noised-based data augmentation method which is composed of Gaussian Noise, Salt-and-Pepper noise, and the PGD adversarial perturbations. The proposed method is built on lightweight algorithms and proved highly effective based on comprehensive evaluations, showing good efficiency on computation cost and robustness enhancement. In addition, we share our insights about the data-centric robust machine learning gained from our experiments.
更多查看译文
关键词
augmentation,machine learning,data-centric,noise-based
AI 理解论文
溯源树
样例
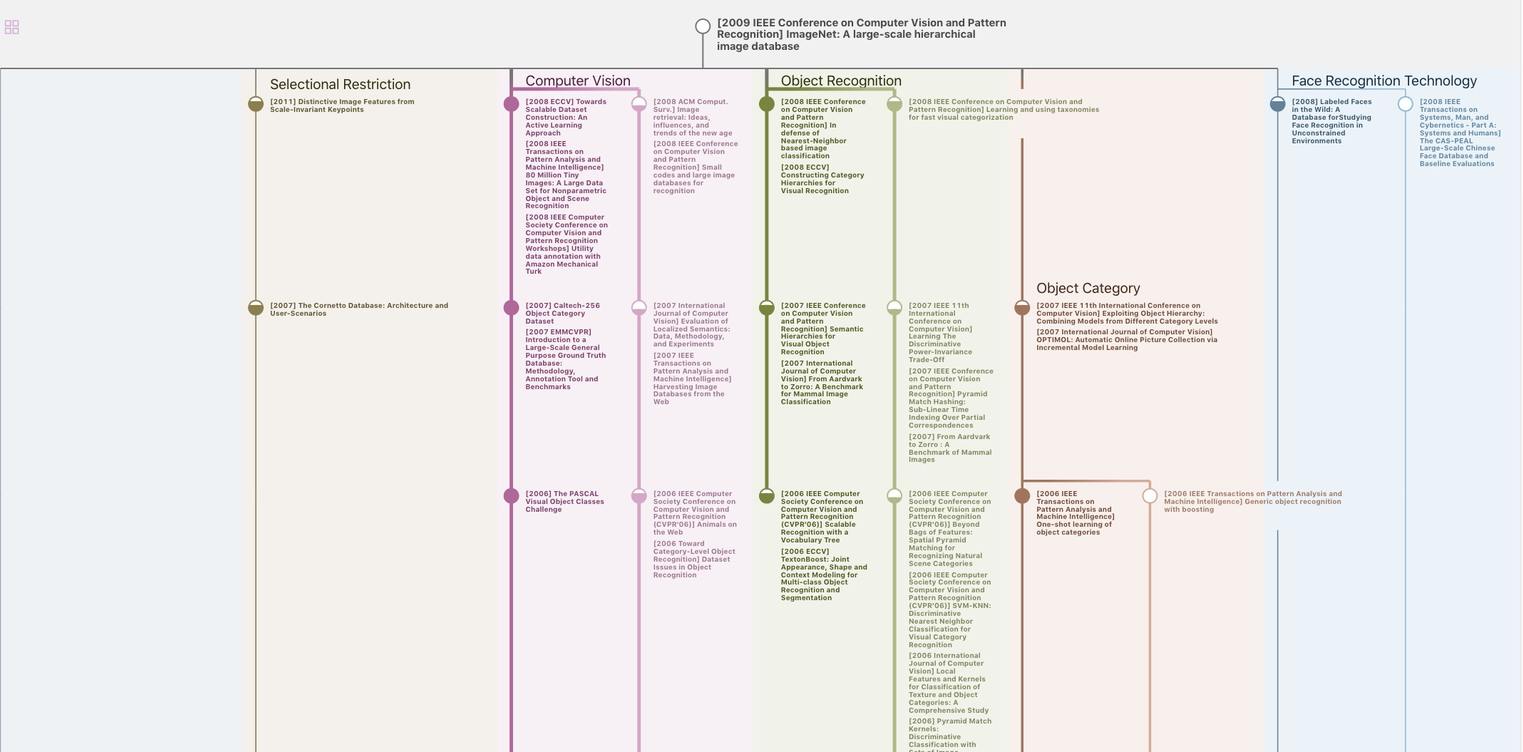
生成溯源树,研究论文发展脉络
Chat Paper
正在生成论文摘要