Detecting apical radiolucencies using deep learning technology: a pilot study
Oral Surgery, Oral Medicine, Oral Pathology, and Oral Radiology(2021)
摘要
BACKGROUND Dentists rely on radiographs in making treatment decisions every day. However, if inattentively read, radiographs can lead to over- or undertreatment. OBJECTIVE(S) The objectives were to assess the diagnostic performance of a deep learning algorithm at detecting apical radiolucencies (ARs) on intraoral (IO) radiographs and compare the performance of the algorithm vs interpretation by experts. STUDY DESIGN A search was conducted using the University of North Carolina cone beam computed tomography (CBCT) referral database for volumes acquired for endodontic evaluation. The inclusion criteria consisted of permanent teeth that exhibited apical radiolucencies measuring ≥2 mm on CBCT with a diagnostic IO radiograph for the same site. The exclusion criteria included patients under 18 years old and CBCT and IO images acquired more than 6 months apart. After applying the inclusion and exclusion criteria, 192 cases were found. The CBCT volumes served as ground truth for the IO radiograph annotation. The IO radiographs were randomly assigned to one of 3 groups: 6 images, observer calibration; 54 images, training the artificial intelligence (AI) algorithm; 132 images, testing set. Additionally, 132 IO radiographs with no evidence of apical radiolucencies were included to serve as controls. For this pilot, 70 images were randomly selected to create a testing subset derived from the main control and positive testing sets. Three experts were presented with this testing subset and asked to independently determine locations of AR and confidence of presence using a 5-point Likert scale. RESULTS The standalone software performance results by tooth were as follows: Sensitivity was 93%, specificity was 88%, and the area under the receiver operating characteristic curve (ROC-AUC) was 94% (95% confidence interval [CI], 89%, 98%). The combined results of the experts were as follows: sensitivity was 87%, specificity was 97%, and ROC-AUC was 93% (95% CI, 88%, 98%). Notably, the software performance metrics were lowest in the maxillary posterior region. DISCUSSION/CONCLUSIONS Using a limited testing data set, AI provided comparable performance to expert observers for this task. Further AI training is necessary to increase the sensitivity and specificity of AR detection in the posterior maxillary region.
更多查看译文
AI 理解论文
溯源树
样例
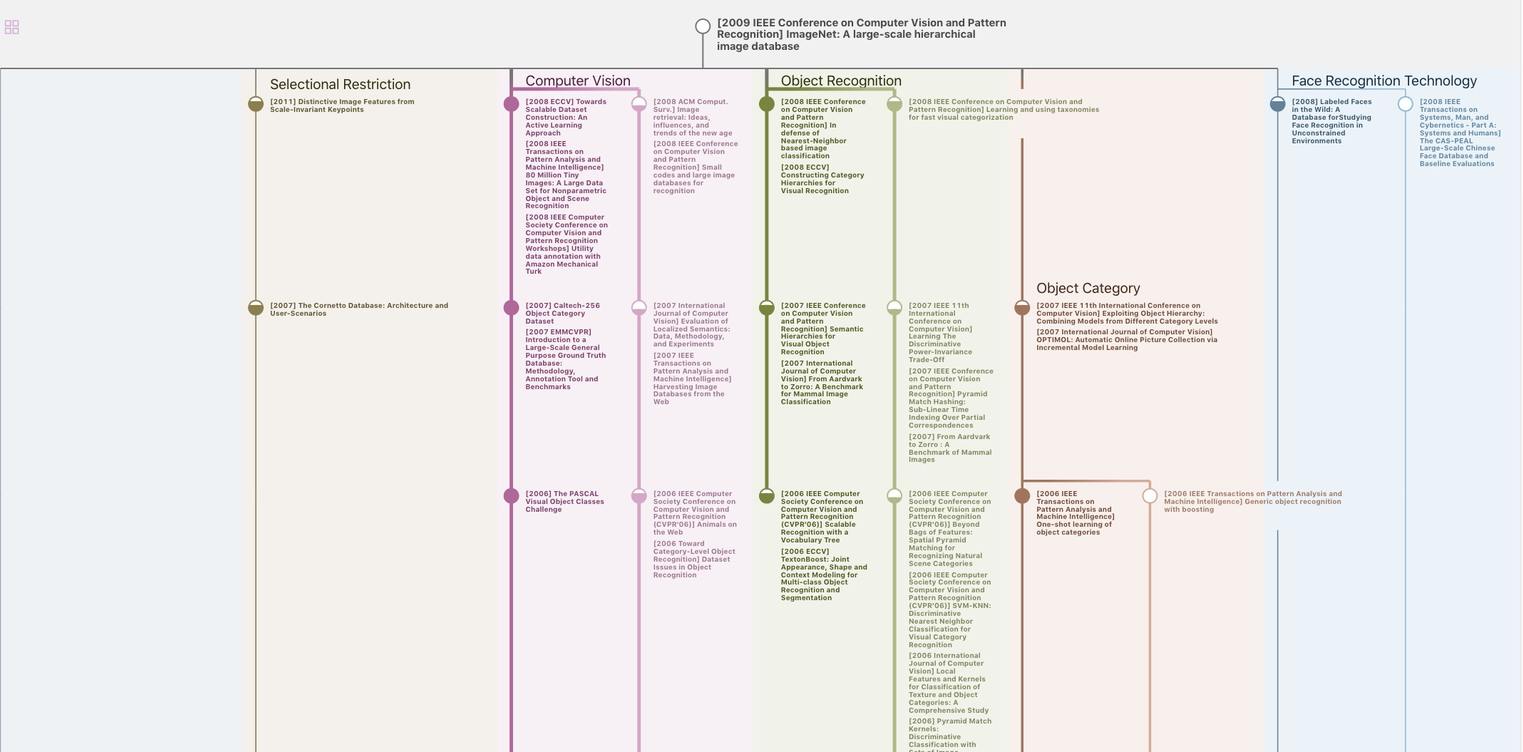
生成溯源树,研究论文发展脉络
Chat Paper
正在生成论文摘要