On-Street Parking Occupancy Inference Based on Payment Transactions
IEEE Transactions on Intelligent Transportation Systems(2022)
摘要
A record of spatial and temporal parking occupancy is critical to optimize on-street parking resources and to develop effective parking policies. Such data are often obtained through advanced and costly occupancy monitoring technologies. Moreover, it is usually challenging to integrate bay-level occupancies and parking data from other systems. Accurate occupancy–payment data are required for a wide range of analytical and practical purposes, including but not limited to investigating payment behavior, estimating and forecasting occupancy, and evaluating the efficiency and effectiveness of enforcement policies. This study proposes a metaheuristic optimization algorithm to integrate snapshots of bay-level parking occupancy, captured using simple cameras, with transactions from a conventional parking payment management system. The resulting integrated data were used to develop, calibrate and validate a parking occupancy estimation method utilizing parking payment data only. Details of the design, implementation, and validation of the proposed algorithm and modelling technique are provided. Logistic regression analysis was used to tune parameters of the data integration algorithm. Deep learning, gradient boosting and random forests were used to develop a model of parking occupancy. Evaluation of the algorithm indicated an accuracy of 76% of correct data integration; that is, individual bay occupancies integrated with the correct corresponding payment transactions. The best occupancy estimation model also showed a very high accuracy, with an R
2
above 94% and a root mean square error (RMSE) of 1.2 (occupied bays), when tested with a random sample from the integrated data.
更多查看译文
关键词
Learning (artificial intelligence),prediction algorithms,software algorithms,inference algorithms,heuristic algorithms
AI 理解论文
溯源树
样例
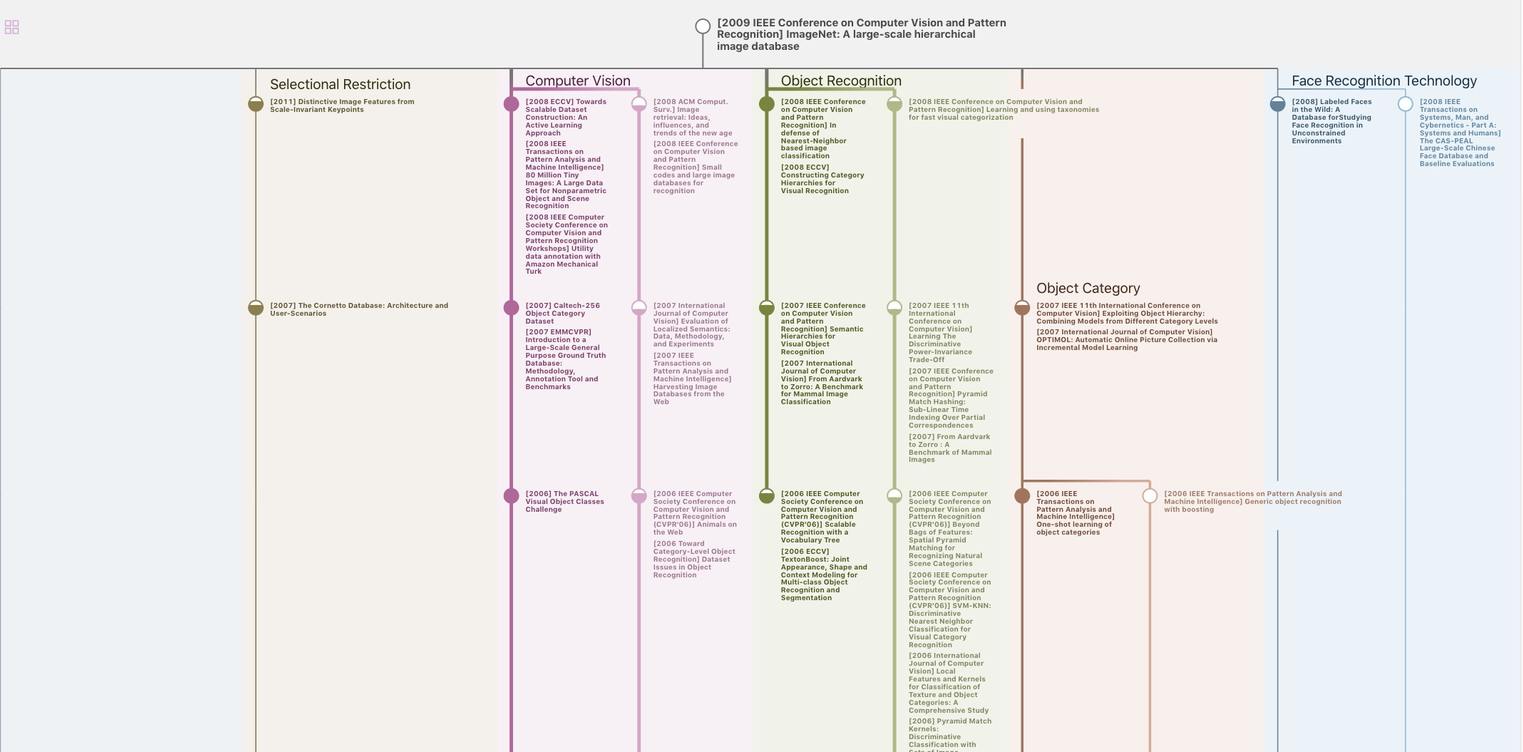
生成溯源树,研究论文发展脉络
Chat Paper
正在生成论文摘要