Automated spider mite damage detection on tomato leaves in greenhouses
Acta Horticulturae(2020)
摘要
Spider mites are one of the most challenging pests in the greenhouse, and their management is more and more challenging due to resistance to pesticides. Spider mites prefer young leaves at the outer canopy, stay at the bottom of leaves, and can crawl to nearby plants. Typical symptoms are small yellow and white spots on the upper side of the leaf due to chlorophyll depletion, leading to bronzing of the leaves. The challenge is to detect the spider mite damage in an early stage, as it is hardly possible to detect the actual spider mites underneath the leaves. Based on a structured requirement list, the following camera systems were identified as detection system candidates. Three multispectral cameras and one RGB camera were further investigated in the research. A lab study with hyperspectral imaging was performed that identified discriminating wavebands between healthy and diseased leaves. The wavebands 700, 716 and 747 nm were selected and used in the multispectral systems. Using Fisher LDA, accuracies up to 0.88 could be reached at pixel level with these multispectral cameras. However, none of the setups could meet the spatial resolution to detect spider mite damage at early stage on the leaves. Second step was to perform color image analysis. An identical accuracy of 0.88 with sufficient spatial resolution was reached. Based on these results, a high resolution RGB camera was mounted on a measurement cart. Experiments were performed in a greenhouse compartment with an ongoing spider mite damage infestation in a tomato crop. The results show the automated pipeline for spider mite damage detection in a time series of four recordings over three months, having an accuracy of 0.90. Heat maps of the infestation rate are presented to the grower and are used for input in models for integrated pest management and to support the decision on the release of beneficial insects.
更多查看译文
关键词
greenhouse, spider mite, deep learning, machine vision, pest detection
AI 理解论文
溯源树
样例
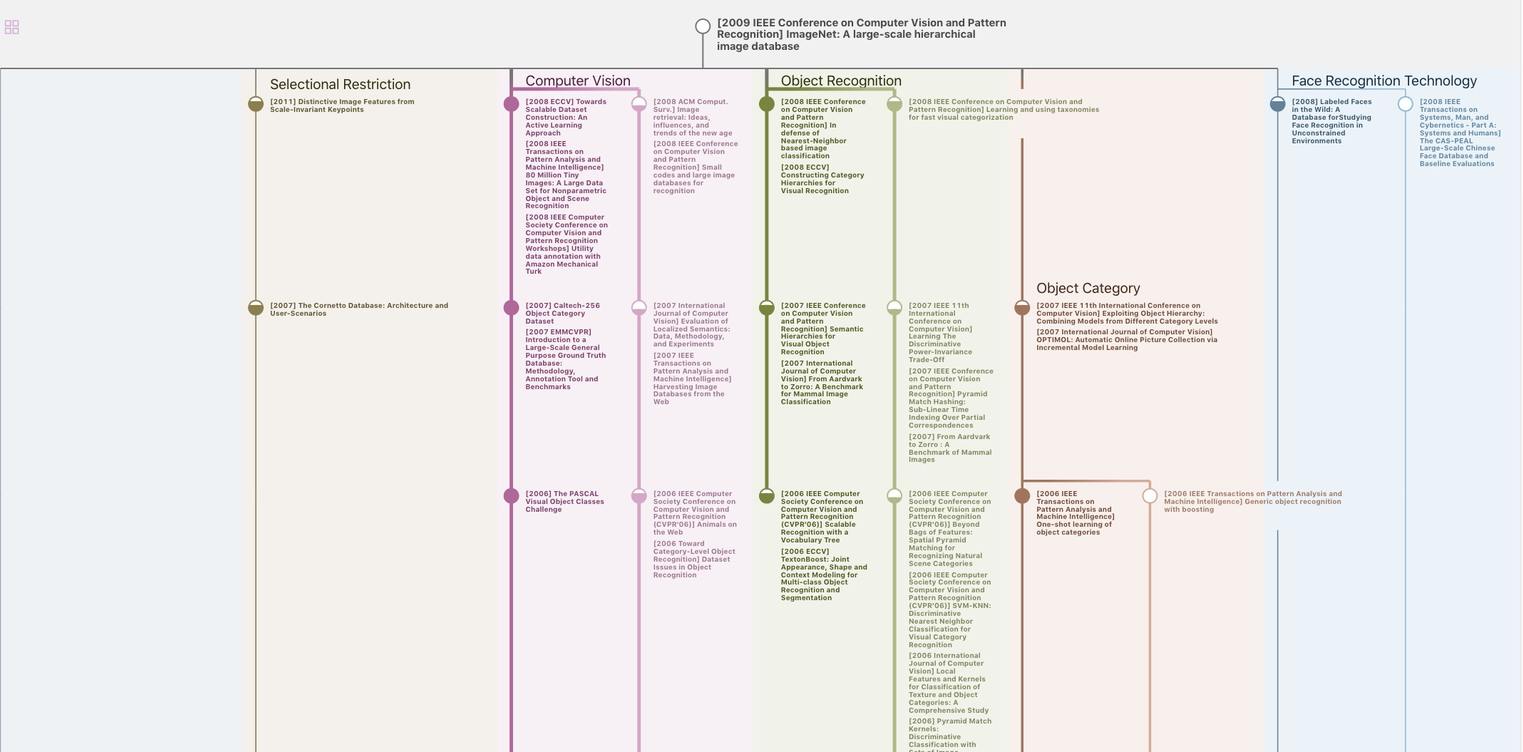
生成溯源树,研究论文发展脉络
Chat Paper
正在生成论文摘要